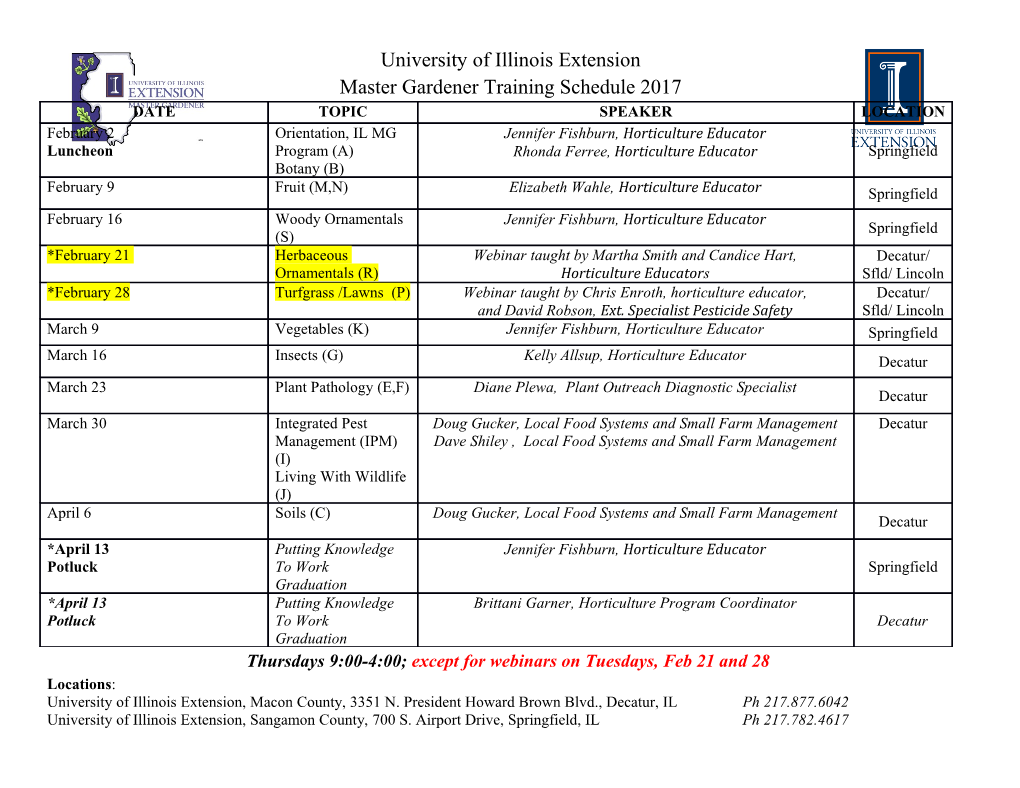
Invited Paper Adaptive spectroscopy: Towards adaptive spectral imaging M.E. Gehm and J. Kinast University of Arizona Department of Electrical and Computer Engineering, Tucson, AZ, 85743 ABSTRACT Spectral imaging is an emerging tool for defense and security applications because it provides compositional information about the objects in a scene. The underlying task—measuring a 3-D dataset using a 2-D detector array—is challenging, and straightforward approaches to the problem can result in severe performance tradeoffs. While a number of ingenious (non-adaptive) solutions have been proposed that minimize these tradeoffs, the complexity of the sensing task suggests that adaptive approaches to spectral imaging are worth considering. As a first step towards this goal, we investigate adaptive spectroscopy and present initial results confirming dramatic cost/performance gains for a particular implementation. Keywords: spectral imaging, adaptive optics, computational sensing 1. INTRODUCTION Spectral imaging is a rapidly-expanding sensing modality for a variety of scientific and engineering applications primarily as a result of the supplementary information it provides about the chemical composition of the materials being imaged. While traditional imagers produce a two-dimensional spatial array of scalar values representing the intensity of a scene, a spectral imager produces a two-dimensional spatial array of vectors which contain the spectral information for the respective spatial locations. This data structure is known as the spectral datacube because of its three-dimensional nature. The addition of spectral data can provide valuable information in a variety of contexts ranging from defense and security applications1, 2 to biochemistry3, 4 to environmental monitoring,5, 6 to astrophysics.7 This manuscript discusses some fundamental challenges to traditional spectral imaging methods and describes a variety of computational sensing approaches that address these challenges. Later sections present the possibility that, for a variety of spectral imaging tasks, reconstructing the complete datacube is not necessary, and that system designs that directly exploit the spectral information would be suitable. In this context, the appeal of adaptive approaches to spectral imaging and spectroscopic exploitation tasks is discussed. The paper concludes with a detailed discussion of dynamic range matching spectroscopy—an implementation of adaptive spectroscopy that is designed for dealing with a common spectroscopic problem. 2. FUNDAMENTAL CHALLENGES IN SPECTRAL IMAGING Straightforward approaches to spectral imaging (those based on simple extensions to traditional imaging or spectroscopy techniques) quickly ran afoul of two major challenges, both of which are fundamentally related to the dimensionality of the spectral imaging datacube. The first difficulty arises from the sheer number of signal elements in the datacube. For systems that involve a large number of spectral channels, the number of signal elements is obviously significantly larger than in traditional images with the same spatial resolution. This has a non-negligible affect on the signal to noise ratio (SNR) of the reconstructed datacube. For a given exposure time, there is some finite number of source photons that can be collected. After measurement acquisition, some methodology must be applied to apportion the photons among the signal elements in the datacube. By virtue of the increased number of signal elements, the overall number of photons assigned to any given signal element of the datacube is necessarily reduced from the result in a traditional imager. Small photon numbers then impact the signal to noise ratio of the estimated datacube in two ways. First, the number may prove small compared to additive noise provided by the measurement system (e.g. readout noise of a detector array). This difficulty can be mitigated with high-end detectors (with low readout noise) or by clever measurement schemes, as will be discussed below. Second, the low photon number leads to an inherent SNR penalty arising from the Visual Information Processing XVII, edited by Zia-ur Rahman, Stephen E. Reichenbach, Mark Allen Neifeld, Proc. of SPIE Vol. 6978, 69780I, (2008) · 0277-786X/08/$18 · doi: 10.1117/12.779174 Proc. of SPIE Vol. 6978 69780I-1 Downloaded from SPIE Digital Library on 01 Oct 2009 to 150.135.220.252. Terms of Use: http://spiedl.org/terms underlying statistical fluctuations of the field (i.e. shot noise). Barring sources with exotic statistics, this penalty is unavoidable. The second difficulty inherent in spectral imaging arises from the dimensionality mismatch between the datacube (3D) and a standard detector array (2D). As we consider datacubes of increasing size, the number of signal elements quickly outpaces the number of available detector elements. Using the standard mantra that solving a system of n unknowns requires at least n equations, we see that this mismatch will prove problematic for traditional reconstruction methods. Ultimately, the only possibilities are to minimize the size of the datacube or to employ some form of scanning approach in which a sequence of data acquisitions produces a total number of measurements (number of detector elements multiplied by number of acquisitions) that equals or exceeds the number of signal elements in the desired data cube. Scanning approaches, of course, incur a considerable time cost and limit the utility of the method for dynamic sources. Recent approaches have shown that, at least in some cases, the requirement that there be a number of measurements equal to or greater than the number of signal elements is also avoidable given clever measurement schemes. This will be discussed below. 2.1 SOLUTIONS TO THESE CHALLENGES Advances in the field of spectral imaging over the past decade have largely focused on system designs that tackle the above challenges. The SNR penalty arising from small photon counts coupled with additive noise can be effectively overcome through the use of signal multiplexing. In multiplexed systems, measurements are not made on individual signal elements, but instead on combinations of elements. By combining multiple signal elements together, the measurement involves a larger number of photons, and the impact of additive noise is greatly reduced. The big challenges in multiplexed systems are in selecting the particular combinations of signal elements to measure and in designing an optical system that is capable of implementing these combinations. One of the first attempts at a multiplexed spectral imager was Mooney’s direct-view system.8 In this approach, an intensity image of the scene was collected through a dispersive element. Whereas a standard approach (e.g. one based on a sequence of measurements through narrowband spectral filters) would collect only a small fraction of available light in a given acquisition, the direct-view system collects nearly all available light (losing only the small portion which misses the detector after interaction with the dispersive element). The system is clearly multiplexed, as any particular detector element receives light from a variety of position/wavelength points in the source datacube. The dimensionality mismatch remains, however, and the number of measurements in a single acquisition is far smaller than the number of signal elements. The direct-view system resolves this difficulty by rotating the dispersive element, and collecting a sequence of acquisitions at different disperser orientations. The technique is effectively a tomographic one, as a given acquisition is equivalent to a projection through the source datacube at an angle with respect to the wavelength axis. The magnitude of the angle is set by the linear dispersion of the system. As is common in tomographic systems, there is a conical, unsampled region of Fourier space (the “missing cone” or “limited angle” problem9) that impacts the final resolution of the reconstruction. Mooney later introduced algorithmic approaches for estimating the missing regions, reducing, though not eliminating, the resolution penalty.10 Not long after the introduction of the direct-view system, Descour and Dereniak introduced a system that also is based on tomographic sampling of the source datacube.11 Their system, dubbed CTIS (Computed-Tomography Imaging Spectrometer), uses a holographic diffraction grating rather than a prism to produce the system disper- sion. As with the direct-view system, there is a dimensionality mismatch between the datacube and the detector array. In contrast to the scanning approach used in the direct-view method, the CTIS approach implements all of the different disperser orientations into different spatial locations on the detector array, sacrificing datacube size (specifically spatial field-of-view) for all-at-once acquisition. As a result, the CTIS approach is applicable to time-varying sources whereas the direct-view method is not. Like the direct-view method, CTIS suffers from the missing cone problem; however the same algorithmic post-processing techniques can minimize the effect. Of the tomographic approaches, the CTIS technique is more widely adopted, and a variety of implementations and applications have appeared.12–14 Recent years have also seen a number of other, non-tomographic multiplexing approaches. The question of which particular signal element combinations should be formed for optimal performance can be formulated Proc. of SPIE Vol. 6978 69780I-2 Downloaded from SPIE Digital Library on 01 Oct 2009 to 150.135.220.252.
Details
-
File Typepdf
-
Upload Time-
-
Content LanguagesEnglish
-
Upload UserAnonymous/Not logged-in
-
File Pages11 Page
-
File Size-