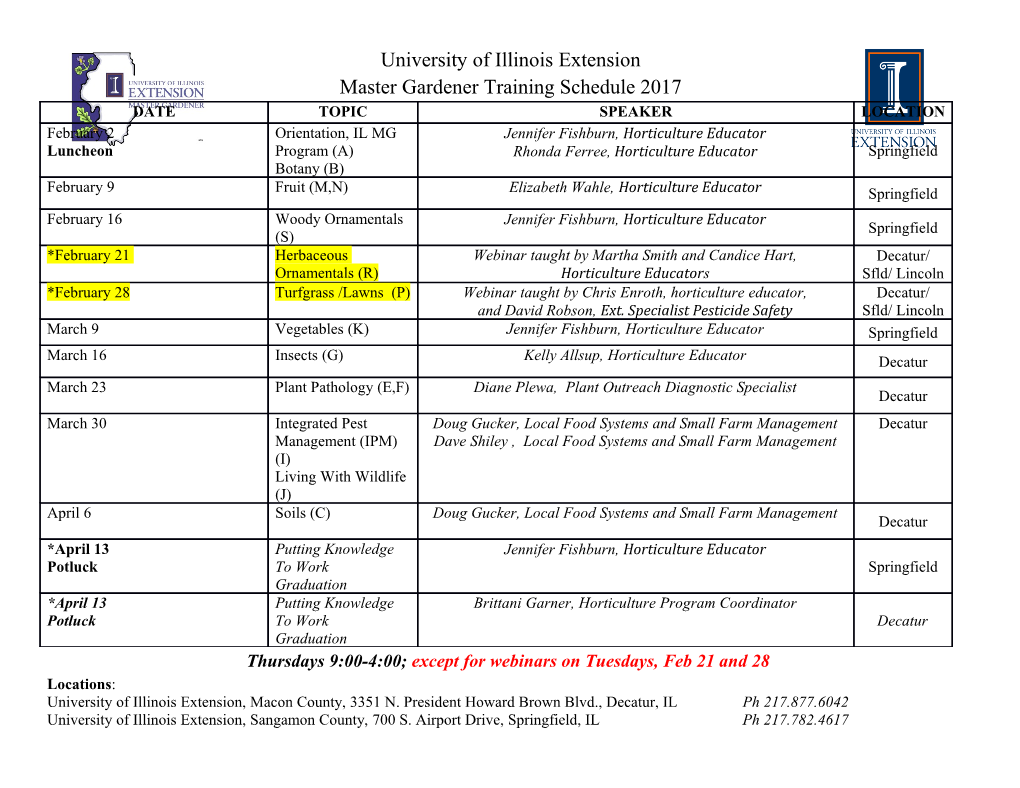
Intensive Care Med (2005) 31:1345–1355 DOI 10.1007/s00134-005-2763-5 ORIGINAL Rui P. Moreno SAPS 3—From evaluation of the patient Philipp G. H. Metnitz Eduardo Almeida to evaluation of the intensive care unit. Barbara Jordan Peter Bauer Part 2: Development of a prognostic model Ricardo Abizanda Campos Gaetano Iapichino for hospital mortality at ICU admission David Edbrooke Maurizia Capuzzo Jean-Roger Le Gall on behalf of the SAPS 3 Investigators Received: 8 April 2005 G. Iapichino randomly selected four fifths of the Accepted: 22 July 2005 Department of Anesthesia and Intensive sample as a development- and the Published online: 17 August 2005 Care Medicine, remaining fifth as validation-set. Lo- Springer-Verlag 2005 Hospital San Paolo, Universit degli Sudi, gistic regression methods were then Milan, Italy used to reduce complexity of the D. Edbrooke model. Final estimates of regression Critical Care Directorate, coefficients were determined by use Royal Hallamshire Hospital, of multilevel logistic regression. Electronic Supplementary Material Sheffield, UK Variables selection and weighting Electronic supplementary material is in- M. Capuzzo were further checked by bootstraping cluded in the online fulltext version of this Department of Anesthesia and Intensive (at patient level and at ICU level). article and accessible for authorised users: Care Medicine, Twenty variables were selected for http://dx.doi.org/10.1007/s00134-005- Hospital of Ferrara, the final model, which exhibited good 2763-5 Ferrara, Italy discrimination (aROC curve 0.848), R. P. Moreno ()) J.-R. Le Gall without major differences across pa- Unidade de Cuidados Intensivos Department Ranimation Mdicale, tient typologies. Calibration was also Polivalente, Hpital St. Louis, Universit Paris VII, satisfactory (Hosmer-Lemeshow Hospital de St. Antnio dos Capuchos, Paris, France goodness-of-fit test Hˆ =10.56, p=0.39, Centro Hospitalar de Lisboa (Zona Central), Cˆ =14.29, p=0.16). Customised equa- Lisbon, Portugal e-mail: [email protected] Abstract Objective: To develop a tions for major areas of the world Fax: +351-21-3153784 model to assess severity of illness and were computed and demonstrate a predict vital status at hospital dis- good overall goodness-of-fit. Con- P. G. H. Metnitz charge based on ICU admission data. clusions: The SAPS 3 admission Department of Anaesthesiology and General Intensive Care, Design: Prospective multicentre, score is able to predict vital status at University Hospital of Vienna, multinational cohort study. Patients hospital discharge with use of data Vienna, Austria and setting: A total of 16,784 patients recorded at ICU admission. Further- consecutively admitted to 303 inten- more, SAPS 3 conceptually dissoci- E. Almeida Unidade de Cuidados Intensivos, sive care units from 14 October to 15 ates evaluation of the individual pa- Hospital Garcia de Orta, December 2002. Measurements and tient from evaluation of the ICU and Pragal, Portugal results: ICU admission data (record- thus allows them to be assessed at ed within €1 h) were used, describ- their respective reference levels. B. Jordan · P. Bauer Department of Medical Statistics, ing: prior chronic conditions and University of Vienna, diseases; circumstances related to and Keywords Intensive care unit · Vienna, Austria physiologic derangement at ICU ad- Severity of illness · ICU mortality · mission. Selection of variables for Hospital mortality · Risk adjustment R. A. Campos inclusion into the model used differ- Department of Intensive Care, Hospital Universitario Asociado General de ent complementary strategies. For Castell, cross-validation, the model-building Castello, Spain procedure was run five times, using 1346 Introduction Second, the SAPS 3 PROBABILITY OF DEATH during a certain period of time (in the case of the main model, the One of the crucial steps in the evaluation of risk-adjusted probability of death at hospital discharge). outcomes is the choice of the reference database for es- Given our objective of evaluating not only individual timating adequate reference lines for the analyzed vari- patient outcome but also the effectiveness of ICU prac- ables. For the SAPS 3 to reflect the standard of practices tices, we focused the model on data available at ICU and outcome in intensive care at the beginning of the 21st admission or shortly thereafter. This model will be century, we decided to collect data from a large sample of completely open and available free of any direct or in- intensive care units (ICUs) worldwide. Other models have direct charges to the scientific community. restricted data collection to large ICUs in Europe or North America—SAPS II [1], MPM II [2], APACHE II [3] and APACHE III [4], a strategy that minimizes the hetero- Methods and statistical analysis geneity of the sample but restricts the generalization of the results. Primary variable selection At the statistical level, there is also a need for change, in order to take into account the hierarchic nature of our Based on the SAPS 3 Hospital Outcome Cohort as de- data [5, 6]. Current general outcome prediction models do scribed in Part 1 of this report, continuous predictive not consider the existence of clinical and nonclinical variables were categorized in mutually exclusive cate- factors, aggregated at the ICU level, that can have an gories based on smoothed curves such as LOWESS [9], important impact on prognosis. Instead, they assume that showing the univariate dependence of hospital mortality these factors are either not important or are randomly on the predictive variables. Classes of categorical vari- distributed throughout large samples and that the variation ables were also collapsed according to their univariate between ICUs is small. This assumption is not likely to be hospital mortality levels using multidimensional tables borne out at the ICU level for either nonclinical factors and clinical judgment as appropriate, depending on the (e.g. organization and management, organizational cul- nature of the data. Additively, regression trees (MART) ture) or clinical factors (e.g. clinical management, diag- [10] were applied to check the cutoffs. nostic and therapeutic strategies). If the variation between Missing values were coded as the reference or “nor- ICUs is not negligible, it will compromise the stability of mal” category for each variable. When dual data collec- the equations used to compute predicted mortality. Fur- tion was used—maximum and minimum values recorded thermore, the published models consider the relation be- during a certain time period—missing maximum values tween performance and severity of illness to be constant, of a variable were replaced by the minimum, if docu- and that may not be the case, since performance can vary mented, and vice versa. Some regression imputations within ICUs according to the severity of illness of the were performed if noticeable correlations to available patients [7, 8]. To overcome this problem, we chose to values could be exploited. For a detailed description of adopt a new strategy for the development of the SAPS 3 data collection and handling, see Part 1 of this report. score and to apply statistical modelling techniques that Selection of variables was done according to their control for the clustering of patients within ICUs instead association with hospital mortality, together with expert of assuming the independence of observations. Concep- knowledge and definitions used in other severity of illness tually, the SAPS 3 admission score comprises the fol- scoring systems. The objective of using this combination lowing parts: of techniques rather than regression-based criteria alone First, the SAPS 3 ADMISSION SCORE, represented by was to reach a compromise between over-sophistication the arithmetic sum of three subscores, or boxes: of the model and knowledge from sources beyond the sample with its specific case mix and ICU characteristics. – Box I: What we know about the patient characteristics before ICU admission: age, previous health status, co- morbidities, location before ICU admission, length of Cross validation stay in the hospital before ICU admission, and use of major therapeutic options before ICU admission. For being able to cross-validate the model, we randomly – Box II: What we know about the circumstances of extracted five roughly equal-sized parts based on number ICU admission: reason(s) for ICU admission, anatomic of patients from the database, as suggested previously site of surgery (if applicable), planned or unplanned [11]. In a second approach, partitioning was based on ICU admission, surgical status and infection at ICU ICUs and not on patients. It was thus possible to run the admission. model-building procedure five times in each of the two – Box III: What we know about the presence and degree approaches, each time taking four parts of the sample as a of physiologic derangement at ICU admission (within development set and the remaining one as the validation 1 h before or after admission). set. This allowed to estimate the variability of prediction 1347 resulting from the construction process of the prognostic the final construction of the SAPS 3 admission score score. A further check of the stability of the predictions sheet. was made by partitioning the sample according to major The stability of the processes of variable selection and patient characteristics, such as surgical status and infec- reducing complexity was further checked by bootstraping tion status. with replacement the total sample 100 times, both at pa- The quality of predictions in the validation sets was tient level and at ICU level. assessed by looking at the goodness-of-fit in terms of the p values for the Hosmer-Lemeshow tests Cˆ and Hˆ [13] and the discriminative capability of the models by the use Predicting hospital mortality of the area under the receiver operating characteristic (aROC) curve [14, 15]. Another criterion to judge the After this step was completed, a shrinking power trans- appropriateness of the model was the fit in certain sub- formation was applied. This procedure uses log-transfor- samples, defined according to major patient typologies mation of the score to reduce the influence of extreme [16].
Details
-
File Typepdf
-
Upload Time-
-
Content LanguagesEnglish
-
Upload UserAnonymous/Not logged-in
-
File Pages11 Page
-
File Size-