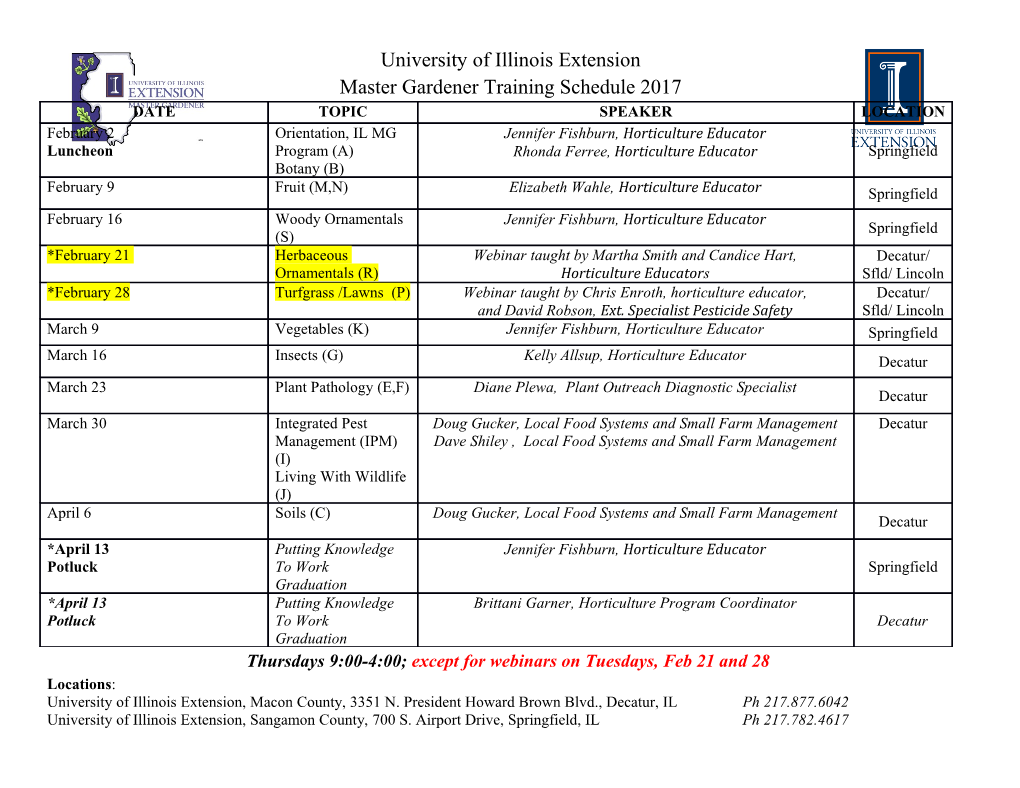
Supplementary Information for Inflammatory and antiviral gene expression in Add Health: Molecular pathways to social disparities in disease emerge by young adulthood Steven W. Cole, Michael J. Shanahan, Lauren Gaydosh, & Kathleen Mullan Harris Corresponding authors: Kathleen Mullan Harris and Steven W. Cole Email: [email protected], [email protected] This PDF file includes: Supplementary text Figures S1 to S2 Tables S1 to S2 Legends for Datasets S1 to S2 SI References Other supplementary materials for this manuscript include the following: Datasets S1 to S2 www.pnas.org/cgi/doi/10.1073/pnas.1821367117 Methods Sample and survey procedures. Data come from the National Longitudinal Study of Adolescent to Adult Health (Add Health), a nationally representative study of U.S. adolescents in grades 7-12 at Wave I in 1994-1995 and followed into adulthood over five waves of data collection. We analyze data from the nationally representative Sample 1 subsample of Wave V, conducted in 2016-2017 when respondents were aged 32-42. Add Health administered Wave V using continuous interviewing over three years (2016-2018), and the sampling design selected 3 random subsamples of eligible Wave V respondents for interview in each year. The subsample of 1126 participants analyzed here are those who consented to provide a blood specimen for RNA analysis during the Sample 1 physical examination visit. Add Health developed this three subsample design in order to release preliminary Wave V data prior to the entire Wave V sample being interviewed (given it took 3 years) and released Sample 1 survey data in 2017. Sample 1 therefore represents a nationally representative sample because a randomly-selected subsample of a nationally-representative sample is also nationally representative (1). The general sampling design, interview procedures, and demographic and biobehavioral variable assessments have been previously described (2, 3). Blood transcriptome profiling. Venipuncture whole blood samples were collected into PAXgene RNA tubes and frozen at -80oC prior to single-pass processing of the first 1143 samples collected during Add Health Wave V (Sample 1). Total RNA was extracted using automated nucleic acid processing systems (Qiagen QIAcube) and tested for suitable mass (RiboGreen RNA > 300 ng; achieved mean = 2,716 ± SD 742) and integrity (Agilent TapeStation RIN > 3; achieved mean = 7.7 ± 0.7) prior to conversion of polyadenylated RNA to cDNA using the QuantSeq 3’ FWD system (Lexogen). Sample-barcoded cDNA libraries were sequenced in multiplex on an Illumina HiSeq 4000 system in the UCLA Neuroscience Genomics Core Laboratory. All assay procedures followed the manufacturers’ standard protocols for this workflow. Multiplex sequencing targeted > 107 single-stranded 65 bp reads per sample. Quality- annotated sequences (FASTQ) derived from Illumina HiSeq Control Software (3.4.0) were mapped to the ENSEMBL hg38 human transcriptome sequence and quantified at the gene level using STAR 2.5.3a. Post-sequencing quality control tested for expected read depth > 107 reads/sample (achieved mean = 11.6 ± 2.6 x 106 mapped reads per sample), >90% of reads aligning to the human transcriptome (achieved mean = 94.0% ± 3.9% mapped), and profile consistency with other samples (average Pearson r with 95 adjacent samples > .85; achieved mean r = .94 ± .02). Samples were assayed in 3 sets of 384 (4 x 96 well plates). Among 1131 unique samples assayed, none failed quality control criteria based on poor input RNA quality and 5 (.44%) failed based on poor endpoint quality metrics (profile consistency r < .85). Data analysis and bioinformatics. Transcript abundance values for each gene were pre-normalized to transcripts per million (TPM), standardized on average expression of 11 pre-specified reference genes (4), floored at 1 TPM to suppress spurious variability, and log2-transformed for analysis by standard linear statistical models relating transcript abundance to individual demographic characteristics (age, sex, race/ethnicity), contextual demographic conditions (U.S. region, family poverty status), biobehavioral factors (BMI, smoking, alcohol consumption), and technical covariates (sample RIN, assay plate, sequencing depth / total mapped reads, and profile consistency with other samples). Linear models were estimated using SAS PROC MIXED with the following specification: proc glm; class Plate; model Expression = RIN Plate TotalMappedReads AvgCorr Sex Age Black Hispanic Asian OthRace Poverty USRegion2 USRegion3 USRegion4 BMI Smoke Drink HeavyDrink / solution; In this syntax, “Expression” represents RNA transcript abundance measures as outlined below and regressor variables were coded as follows: age (continuous self-reported years), sex (self-reported biologically assigned male sex at birth, coded by an indicator relative to reference point female), race/ethnicity (self-identified Asian, non-Hispanic Black, Hispanic, and Other race/ethnicity, each coded by an indicator relative to reference point non-Hispanic White), US region (census regions 2-4: Midwest, South, and West, each coded by an indicator relative to reference point region 1, Northeast), family poverty status (self-reported household income <= 2015 US Federal poverty level based on household size, coded by an indicator relative to non-poverty status), BMI (continuous kg/m2 derived from self- reported continuous height and weight), smoking history (self-reported ever smoked coded by an indicator relative to never smoked reference point), and alcohol consumption (represented as 2 variables; one “regular drinking” variable indicating whether participants self-reported drinking beer, wine, or liquor every day or almost every day, relative to less frequent drinking during the past 12 mo; and a second “binge drinking” ordinal variable reflecting days during the past 12 mo during which participants drank [female 4/male 5] or more drinks in a row, coded none=0, 1-2 d/yr = 1, 3-12 day/yrs=1 d/mo=2, 2-3 d/mo=3, 1-2 d/wk=4, 3-5 d/wk=5, every/almost every day=6), assay batch (nominal indicators for plates 1- 11 relative to reference point plate 12), sample RNA integrity (continuous 0-10 RIN), total mapped reads per sample (continuous/106), read alignment rate (continuous %), and profile consistency (average Pearson r with 95 adjacent samples). Among the 1143 total samples assayed, 17 came from subsequent re-assessments of a given individual and were deleted, yielding a sample of 1126 unique participants. Among these 1126 participants, 57 were missing data on one or more of the demographic and behavioral variables analyzed, leaving a total of 1,069 individuals in the final analytic sample. For Level 1 analyses of pre-specified gene sets, inflammatory and Type I interferon composite scores were derived from previous research (5) and computed by averaging z-score standardized RNA abundance values for 19 gene transcripts involved in inflammation (CXCL8, FOS, FOSB, FOSL1, FOSL2, IL1A, IL1B, IL6, JUN, JUNB, JUND, NFKB1, NFKB2, PTGS1, PTGS2, REL, RELA, RELB, TNF) and 30 gene transcripts involved in Type I interferon responses (GBP1, IFI16, IFI27, IFI27L1, IFI27L2, IFI30, IFI35, IFI44, IFI44L, IFI6, IFIH1, IFIT1, IFIT2, IFIT3, IFIT5, IFITM1, IFITM2, IFITM3, IFITM4P, IFITM5, IFNB1, IRF2, IRF7, IRF8, MX1, MX2, OAS1, OAS2, OAS3, OASL). For consistency with the use of the Type I interferon composite in previous research (5), we also included in that score two gene transcripts that were originally implicated in antibody production (JCHAIN, IGLL1) but have since been discovered to co-express with Type I interferon genes in dendritic cells (6-8). A composite score assessing the CTRA profile was computed as the difference between the average standardized value of the 19 pro- inflammatory indicator genes and the average standardized value of the 32 Type I interferon indicator genes. Standard OLS linear statistical models were employed to quantify the magnitude of variation in inflammatory, interferon, and CTRA composite score expression as a function of the demographic, biobehavioral, and technical factors (using the SAS PROC GLM syntax above. A global omnibus F ratio was used to simultaneously test the aggregate contributions of ten dimensions of demographic variation, including six dimensions of individual demographic variation (age, sex, and four indicator parameters representing variation as a function of race/ethnicity) and four dimensions of contextual demographic variation (indicator variables for poverty status and variation across 4 US Census regions), across the three gene sets analyzed (9-12). Contingent on a significant omnibus test of global sociodemographic variation in gene set expression, we conducted interpretive follow-up analyses testing for significant sociodemographic variation in expression of each gene composite in isolation (with False Discovery Rate (13) correction for multiple testing). For gene sets showing a significant omnibus test of global sociodemographic variation, we present the individual parameter estimates underlying those global results for descriptive/interpretive purposes and conduct follow-up nested hypothesis tests to assess the respective effects of individual vs. contextual demographic factors (again with False Discovery Rate correction for multiple testing), as well as ancillary hypotheses involving biobehavioral factors that might potentially confound sociodemographic effects. Individual parameter estimates are presented for descriptive purposes only and do not serve as the analytic basis
Details
-
File Typepdf
-
Upload Time-
-
Content LanguagesEnglish
-
Upload UserAnonymous/Not logged-in
-
File Pages11 Page
-
File Size-