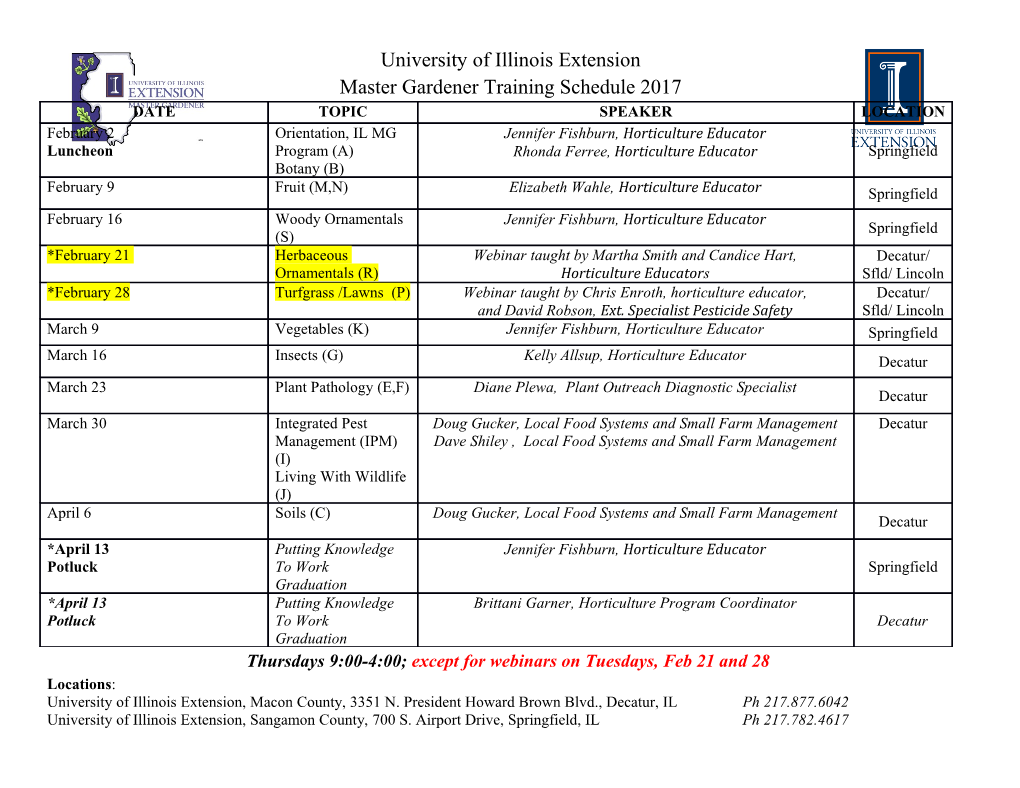
Songklanakarin J. Sci. Technol. 42 (5), 1125-1134, Sep. - Oct. 2020 Original Article Flexible Lomax distribution Muhammad Ijaz*, Muhammad Asim, and Alamgir Khalil Department of Statistics, University of Peshawar, Peshawar, Khyber Pakhtunkhwa, 25130 Pakistan Received: 14 February 2019; Revised: 21 May 2019; Accepted: 29 July 2019 Abstract In this paper, a new probability model is produced, which is actually a modification of the Lomax distribution, called Flexible Lomax (FL). The Flexible Lomax performs better than Lomax and its other invariants. The statistical properties of the Flexible Lomax distribution including quantile function, maximum likelihood estimation, order statistics, and rth moments are derived. To illustrate the efficiency of the proposed distribution, we use two real-life data sets and the results are then compared by means of AIC, CAIC, BIC, and HQIC. It is shown that the proposed distribution fits these data better than Lomax and its different variants. Keywords: Rth moment, quantile function, characteristic function, median, mode, order statistics, TTT plots, maximum likelihood estimation 1 1. Introduction 1 x fx ,1 2 Statistician often need various lifetime probability 1x distributions like Lomax, Weibull-Lomax (Tahir, Cordeiro, Mansoor, Zubair, & Tahir, 2015), and Gamma-Lomax Lemonte and Cordeiro (2013) introduced an extended Lomax (Cordeiro, Ortega, & Popovic, 2015). These distributions have distribution with parameters (, , ac, , ) and the probabi- desirable properties and physical interpretations. This paper lity density function present a new modification of the Lomax distribution, called ac1 1 cx Flexible Lomax (FL). fx 1 1 1 1 xx B ac ,1 Prior literature provides different modifications of (1.1) the Lomax distribution. Ghitany, Awadhi, and Alkhalfan (2007) presented Marshall–Olkin extended Lomax distribution For other modifications of the Lomax distribution, and its application to censored data. The probability density we refer to Afify, Nofal, Yousof, El Gebaly, and (2015), function of MOEL is Ashour and Eltehiwy (2013, 1(6), 7(7)), Al-Zahrani and Sagor (2014), El-Bassiouny, Abdo and Shahen (2015) and Shams (2013). Dias, Alizadeh and Cordeiro (2016) introduced *Corresponding author Email address: [email protected] Beta Nadarajah-Haghighi distribution. Domma and Condino 1126 M. Ijaz et al. / Songklanakarin J. Sci. Technol. 42 (5), 1125-1134, 2020 (2013) presented the beta-Dagum distribution. Korkmaz and Figure 1 shows the graphs of the probability density function Genç (2017) introduced a new generalized two-sided class of and the cumulative distribution function, for various para- distributions with an emphasis on a two-sided generalized meter values. normal distribution. For the different families of distributions, we refer to Aldeni and Famoye (2017), Cordeiro (2017), Alzaatreh, Famoye and Ghosh (2016), Alzaatreh and Famoye (2014), and Nasir, Aljarrah, Jamal, and Tahir (2017). Otunuga (2017) has worked on the Pareto-g extended Weibull distribution. For further details on relevant distributions, see Eddy (2007), El-Gohary, Alshamrani and Otaibi (2013), Famoye, Alzaatreh (2013), Aarset (1987), and Boyd (1988). 2. Flexible Lomax (FL) Distribution The main objective of the present paper is to introduce a new modification of the Lomax distribution with increased number of parameters. The proposed distribution (FL) has three parameters, and its cumulative distribution function takes the form c a x F x 1 1 , x 0 b (2.1) Other statistical characteristics implied by (2.1) are as follows. c a 1 acc1 x f x x1 , x 0 bc b Figure 1. The Pdf and Cdf of Flexible Lomax. (2.2) The hazard rate function of the FL distribution is 3. Behavior of the Pdf and Hazard Rate Function ac xc1 Theorem 3.1. The Pdf of Flexible Lomax distribution fx hx cc b x 1 is b a. increasing when a, b 0, c 1 (2.3) b. decreasing when a0, 0 c 1, b 0 The survival function of the FL distribution is Proof. The first derivative from Equation (2) is c a x Sx 1 b c a 1 d acc1 x (2.4) fx () c x 1 dx b b M. Ijaz et al. / Songklanakarin J. Sci. Technol. 42 (5), 1125-1134, 2020 1127 cca 2 xx acxc2 1ac 1 c 1 b b (3.1.1) bc Since fx() 0 for 01c the fx() is decreasing. If c 1, then fx( ) 0 gives the mode 1 c 1 c (3.1.2) xbm ,c 1 and b 0 ac 1 For parameter values , cca 3 2 c c3 x x x acx 1 ac 1 ac 2 c 1 3 a 1 c 4 cc 1 2 b b b fx() bc satisfies fx( ) 0 , and hence is convex. Figures 1 shows plots for various parameter choices. Theorem 3.2. The hazard rate function of Flexible Lomax abc,, distribution hx is a. increasing when b. decreasing when Proof. The derivative of the hazard rate function in Equation (3) is given by c x acxc2 c 1 (3.2.1) b hx c x bc 12 b hx 0 for , so the hazard rate function is decreasing. If , then hx 0 specifies the global maximum of at 1 xm b1 cc (3.2.2) Now, the second derivative of the hazard rate function is cc a, b 0, c 1 c3 xx 22 acx 2 c 3 c 4 c 3 c 2 bb a 0, 0 c 1, b 0 (3.2.3) h x c 3 x bc 1 b For parameter values , and hence, the hazard rate function has the ability to model shapes both a, b 0, c 1 h xm 0 monotonically and non-monotonically in bathtub shape. Figure 2 shows plots of the hazard function of the Flexible Lomax distribution for various choices of the parameters. 1128 M. Ijaz et al. / Songklanakarin J. Sci. Technol. 42 (5), 1125-1134, 2020 5. Rth Moments Theorem 5.1. If X has a Flexible Lomax distribution with parameters abc,, then the rth moments (about the origin) of , say ur do not exist. rr ur E()() x x f x dx 0 c a 1 acrc1 x ur c x1 dx 0 b b Figure 2. Hazard rate function of the Flexible Lomax 4. Quantile Function and Median c a 1 ac x (5.1.1) xrc11 dx c Among the related statistical functions, the quantile b 0 b function is often useful. The quantile function Qx() of ()FL c x the FLabc,, is the real solution to the following equation Let, y 1 , then (5.1.1) can be rewritten as b c a x r (4.1) a 1 11 u r c (5.1.2) b ab y1 y dy 1 where u ~Uniform (0,1). Now, by using the binomial expansion we have r rk r Solving (4.1) for x , the result is c yy11c k c k0 k log 1 u acl og b (4.2) So, equation (5.1.2) takes the form xe ac rk r r c ka1 (5.1.3) ab 1 c y dy 1 k0 1 For the median, we have to put u in equation (4.2) to k 2 find In (5.1.3) the integral is undefined and thus the statement 1 log acl og b proved. However, one can find the moments if ka , and 2 xe ac they take the form r c r ck k u abr 1 (5.1.4) r ak k0 M. Ijaz et al. / Songklanakarin J. Sci. Technol. 42 (5), 1125-1134, 2020 1129 6. Order Statistics c ac nnlxog i (7.3) nlogcc c 1 l og x a 1 bbii11i Let be ordered random variables, XXXX1, 2 , 3 ... n Now we have to compute the first partial derivatives of (7.3) and on setting them equal to zero we have then the pdf of the ithorder statistic is, lxog c n! (i 1) ( n i ) n n i (7.4) f f( x ) F ( x ) [1 F ( x )] , c 0 (i ; n )( x ) (i 1)!( n i )! a i1 b (6.1) c n lxog nc ca 10 i (7.5) The 1st and nth order probability density functions of FL can i1 bc1 b be obtained by substituting (2.1) and (2.2) in (6.1) is given by, n c2 a1 l og x n i a 1 a n 1 n i1 (7.6) cc lxog 0 ac x x i f()x n xc11 1 c i1 bc1 (1):n c b bb (6.2) The above equations from (7.4) to (7.6) are not in closed form, instead numerical methods are needed to get the MLE. n1 aa 1 cc acc1 x x f()x nc x 1 11 (:)nn b bb 8. Asymptotic Confidence Bounds (6.3) Since, the MLE of the unknown parameters abc,, 7. Parameter Estimation are not in closed form, it is not possible to derive the exact distribution of the MLE. We have derived asymptotic confidence bounds for the unknown parameters of In this section, the usual maximum likelihood based on the asymptotic distribution of MLE. For approach is used to estimate the unknown parameters in FL a,, b c based on complete information. Let us assume the information matrix, we have to find the second partial derivatives of the equations from (7.4) to (7.6), and these are that we have a sample from . The likelihood function is given by n I (8.1) aa2211 n (7.1) L f xi;,, a b c i1 c n lxog i (8.2) Ic ab 12 i1 bc1 Substituting (2.2) in (7.1), we get c n lxog i (8.3) a 1 Ic c 13 c1 n acc1 x (7.2) ac i1 b L c x 1 i1b b n 2c c 1 a 1 l og x nc i I i1 (8.4) Then the log-likelihood function is b222 b 2 bc 2 1130 M.
Details
-
File Typepdf
-
Upload Time-
-
Content LanguagesEnglish
-
Upload UserAnonymous/Not logged-in
-
File Pages10 Page
-
File Size-