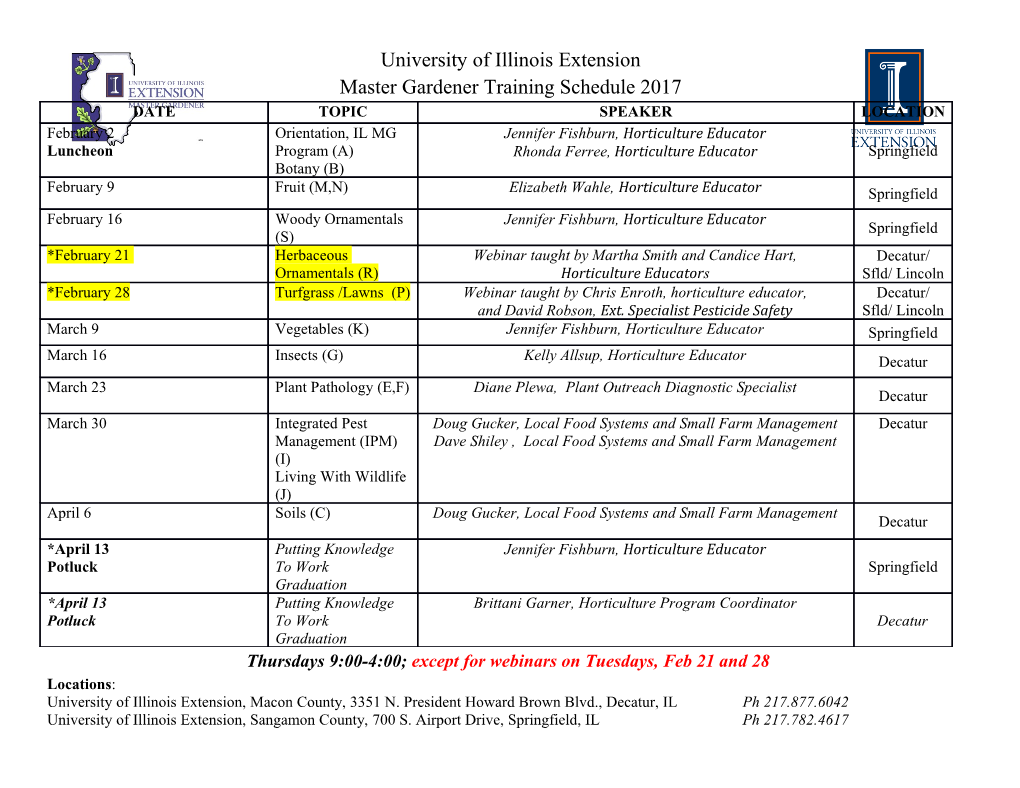
Parallel Processing SSC-0114 ARQUITETURA DE COMPUTADORES 28/09/2017 Recap 2 Recap (continued) • Tesla P100 x V100 3 Recap (continued) 4 Recap (continued) 5 Recap (continued) 6 Recap (continued) 7 Recap (continued) 8 Recap (continued) 9 Recap (continued) 10 News in architecture … https://www.top500.org/news/intel-take-aim-at-ai-with-new-neuromorphic-chip-1/ 11 News in architecture (continued) https://www.top500.org/news/paderborn-university-will-offer-intel-cpu-fpga-cluster-for-researchers/ 12 Agenda • Parallel Processing • Parallel Computing: Flynn Taxonomy • Parallel Computing –Symmetric multiprocessing –Non-uniform memory access (NUMA) –Clusters 13 Parallel processing Definition It is using multiple processors simultaneously to solve a problem. Purpose Improve performance (shorter run time - reduced time needed to solve a problem) Motivations 1. More complex problems 2. Growing problems 3. Concerns about microelectronics limits 4. Concerns about energy/power consumption 5. …. Classifications ? 14 Flynn’s Taxonomy Mike Flynn, “Very High-Speed Computing Systems,” Proceedings of IEEE, 1966 • SISD • SIMD • MISD • MIMD 15 Flynn’s Taxonomy Mike Flynn, “Very High-Speed Computing Systems,” Proceedings of IEEE, 1966 • SISD • SIMD • MISD • MIMD 16 Flynn’s Taxonomy (continued) Mike Flynn, “Very High-Speed Computing Systems,” Proceedings of IEEE, 1966 • SISD • Single instruction operates on single data element • SIMD Corresponds to the Von Neumann architecture • MISD Includes ILP techniques such as • MIMD superscalar and speculative execution Uniprocessor 17 Flynn’s Taxonomy (continued) Mike Flynn, “Very High-Speed Computing Systems,” Proceedings of IEEE, 1966 • SISD • Single instruction operates on multiple data elements • SIMD Vector processors • MISD GPU (SIMT) • MIMD 18 Flynn’s Taxonomy (continued) Mike Flynn, “Very High-Speed Computing Systems,” Proceedings of IEEE, 1966 • SISD • Multiple instructions operate on single data element • SIMD Systolic arrays • MISD • MIMD 19 Flynn’s Taxonomy (continued) Mike Flynn, “Very High-Speed Computing Systems,” Proceedings of IEEE, 1966 • SISD • Multiple instructions operate on multiple data element • SIMD Multiprocessor • MISD Multithreaded processor • MIMD 20 Multiprogramming and Multiprocessing 21 Multiprogramming and Multiprocessing (continued) SISD architectures 22 Multiprogramming and Multiprocessing (continued) SIMD architectures Concurrency arises from performing the same operations on different pieces of data Same processs 23 Multiprogramming and Multiprocessing (continued) MIMD architectures Concurrency arises from performing different operations on different pieces of data Control/thread parallelism: execute different threads of control in parallel multithreading, multiprocessing 24 Taxonomy of Parallel Processor Architectures 25 Multiple Instruction Multiple Data • Most common parallel hardware architecture today – Example: All many-core processors, clusters, distributed systems • In contrast to a SIMD computer, a MIMD computer can execute different programs on different processors. Flexibility?! 26 MIMD – From software perspective [Gregory F. Pfister, 1993] • SPMD - Single Program Multiple Data – Sometimes denoted as ‘application cluster’ – Examples: Load-balancing cluster or failover cluster for databases, web servers, application servers, ... • MPMD - Multiple Program Multiple Data – Multiple implementations work together on one parallel computation – Example: Master / worker cluster, map / reduce framework 27 MIMD – Main characteristics • MIMD processors work asynchronously, and don’t have to synchronize with each other. – Local clocks can run at higher speeds. • They can be built from commodity (off-the-shelf) microprocessors with relatively little effort. • They are also highly scalable, provided that an appropriate memory organization is used. 28 SIMD vs MIMD • A SIMD computer requires less hardware than a MIMD computer (single control unit). • However, SIMD processors are specially designed, and tend to be expensive and have long design cycles. • Not all applications are naturally suited to SIMD processors. 29 SIMD vs MIMD (continued) • Conceptually, MIMD computers cover also SIMD need. – By letting all processors executing the same program (single program multiple data streams ─ SPMD). • On the other hand, SIMD architectures are more power efficient, since one instruction controls many computations. – For regular vector computation, it is still more appropriate to use a SIMD machine (e.g., implemented in GPUs). 30 MIMD Communications methods 1. Centralized Memory or Shared Memory Architectures Shared memory located at centralized location – consisting usually of several interleaved modules – the same distance from any processor. – Symmetric Multiprocessor (SMP) – Uniform Memory Access (UMA) 31 MIMD Communications methods (continued) 2. Distributed Memory: Memory is distributed to each processor – improving scalability. – Message Passing Architectures: No processor can directly access another processor’s memory. – Hardware Distributed Shared Memory (DSM): Memory is distributed, but the address space is shared. • Non-Uniform Memory Access (NUMA) – Software DSM: A level of OS built on top of message passing multiprocessor to give a shared memory view to the programmer. 32 MIMD with Shared Memory Bottleneck? memory access • Tightly coupled, not very scalable. • Typically called Multi-processor systems. 33 MIMD with Distributed Memory Shared nothing Shared disks RAID • Loosely coupled with message passing, scalable. • Typically called Multi-computer systems. 34 Shared Memory Architectures • All processors act independently, access the same global address space • Changes in one memory location are visible for all others Uniform memory access (UMA) system • Equal load and store access for all processors to all memory • Default approach for majority of SMP (symmetry) systems in the past 35 Shared Memory Architectures (continued) Non-uniform memory access (NUMA) system • Delay on memory access according to the accessed region • Typically realized by processor interconnection network and local memories • Cache-coherent NUMA (CC-NUMA), completely implemented in hardware • About to become standard approach with recent x86 chips 36 NUMA Classification 37 MIMD Approaches • SMP (Symmetric Multiprocessors) • NUMA architecture • Clusters 38 Symmetric Multiprocessors (SMP) • Originally for science, industry, and business where custom multithreaded software could be written • Stand alone computer with the following traits – 2 or more processors of comparable capacity –Processors share same memory and I/O – The processors are connected by a bus or other interconnections. –They share the same memory. • A single memory or a set of memory modules. 39 Symmetric Multiprocessors (continued) • Memory access time is approximately the same for each processor • All processors share access to I/O. – Either through the same channels or different channels giving paths to the same devices. • All processors can perform the same functions (hence symmetric) • System controlled by integrated operating system providing interaction between processors • Performance improvement of SMP will not be a multiple of the number of processors – choked by RAM access and cache coherence 40 Organization of Tightly Coupled Multiprocessor • Individual processors are self-contained, i.e., they have their own control unit, ALU, registers, one or more levels of cache, and private main memory • Access to shared memory and I/O devices through some interconnection network • Processors communicate through memory in common data area 41 Organization of Tightly Coupled Multiprocessor (continued) • Memory is often organized to provide simultaneous access to separate blocks of memory • Bus – Time-shared or common bus – Multiport memory – Central controller (arbitrator) 42 Organization of Tightly Coupled Multiprocessor (continued) 43 Time Shared BUS • Simple extension of single processor bus – Can be likened to relationship between DMA and single processor • Following features provided – Addressing – distinguish modules on bus – Arbitration • Any module can be temporary master • Must have an arbitration scheme – Time sharing – if one module has the bus, others must wait and may have to suspend • Now have multiple processors as well as multiple I/O modules on a single bus 44 Time Shared BUS (continued) • Waiting for bus creates bottleneck – Limited number of processors can be managed • How to improve memory access? CPU CPU CPU CPU Memory 45 SMP with BUS time sharing Time Shared BUS (continued) • Local CPU cache – Decrease bus usage • More processors can be used: 16 or 32 (TANENBAUM; GOODMAN, 1999). • How to deal with cache coherence? CPU CPU CPUCPU CPU CPU Memory 46 SMP with BUS time sharing + CACHE Time Shared BUS (continued) 47 Time Shared BUS (continued) • Advantages – Simplicity – not only is it easy to understand, but its use has been well-tested with DMA – Flexibility – adding processor involves simple addition of processor to bus – Reliability – As long as arbitration does not involve single controller, then there is no single point of failure 48 Time Shared BUS (continued) • Disavantages – Waiting for bus creates bottleneck • Can be helped with individual caches • Usually L1 and L2 – Cache coherence policy must be used (usually hardware) 49 Multiport Memory • Direct independent access of memory modules by each processor and I/O module • Memory handles "arbitration" – Logic internal to memory required to resolve conflicts
Details
-
File Typepdf
-
Upload Time-
-
Content LanguagesEnglish
-
Upload UserAnonymous/Not logged-in
-
File Pages135 Page
-
File Size-