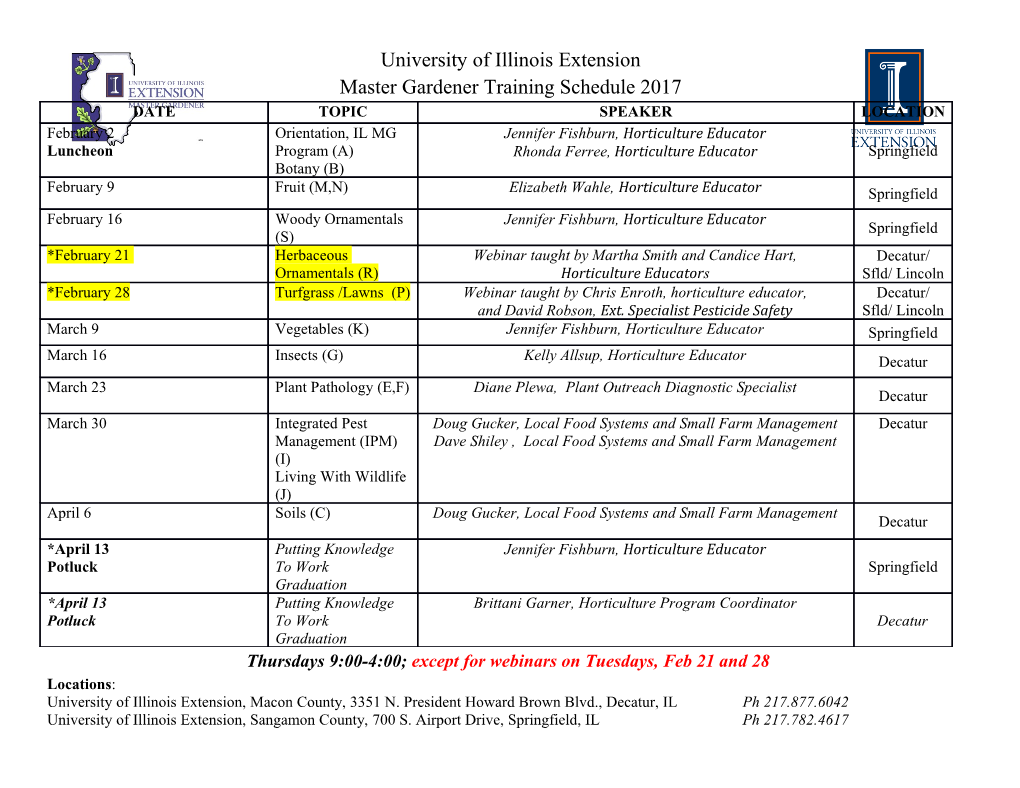
Machine Learning Basics Lecture 3: Perceptron Princeton University COS 495 Instructor: Yingyu Liang Perceptron Overview • Previous lectures: (Principle for loss function) MLE to derive loss • Example: linear regression; some linear classification models • This lecture: (Principle for optimization) local improvement • Example: Perceptron; SGD Task (푤∗)푇푥 = 0 (푤∗)푇푥 > 0 (푤∗)푇푥 < 0 ∗ Class +1 푤 Class -1 Attempt • Given training data 푥푖, 푦푖 : 1 ≤ 푖 ≤ 푛 i.i.d. from distribution 퐷 푇 • Hypothesis 푓푤 푥 = 푤 푥 • 푦 = +1 if 푤푇푥 > 0 • 푦 = −1 if 푤푇푥 < 0 푇 • Prediction: 푦 = sign(푓푤 푥 ) = sign(푤 푥) • Goal: minimize classification error Perceptron Algorithm • Assume for simplicity: all 푥푖 has length 1 Perceptron: figure from the lecture note of Nina Balcan Intuition: correct the current mistake • If mistake on a positive example 푇 푇 푇 푇 푇 푤푡+1푥 = 푤푡 + 푥 푥 = 푤푡 푥 + 푥 푥 = 푤푡 푥 + 1 • If mistake on a negative example 푇 푇 푇 푇 푇 푤푡+1푥 = 푤푡 − 푥 푥 = 푤푡 푥 − 푥 푥 = 푤푡 푥 − 1 The Perceptron Theorem ∗ • Suppose there exists 푤 that correctly classifies 푥푖, 푦푖 ∗ • W.L.O.G., all 푥푖 and 푤 have length 1, so the minimum distance of any example to the decision boundary is ∗ 푇 훾 = min | 푤 푥푖| 푖 1 2 • Then Perceptron makes at most mistakes 훾 The Perceptron Theorem ∗ • Suppose there exists 푤 that correctly classifies 푥푖, 푦푖 ∗ • W.L.O.G., all 푥푖 and 푤 have length 1, so the minimum distance of any example to the decision boundary is ∗ 푇 훾 = min | 푤 푥푖| 푖 Need not be i.i.d. ! 1 2 • Then Perceptron makes at most mistakes 훾 Do not depend on 푛, the length of the data sequence! Analysis 푇 ∗ • First look at the quantity 푤푡 푤 푇 ∗ 푇 ∗ • Claim 1: 푤푡+1푤 ≥ 푤푡 푤 + 훾 • Proof: If mistake on a positive example 푥 푇 ∗ 푇 ∗ 푇 ∗ 푇 ∗ 푇 ∗ 푤푡+1푤 = 푤푡 + 푥 푤 = 푤푡 푤 + 푥 푤 ≥ 푤푡 푤 + 훾 • If mistake on a negative example 푇 ∗ 푇 ∗ 푇 ∗ 푇 ∗ 푇 ∗ 푤푡+1푤 = 푤푡 − 푥 푤 = 푤푡 푤 − 푥 푤 ≥ 푤푡 푤 + 훾 Analysis Negative since we made a • Next look at the quantity 푤푡 mistake on x 2 2 • Claim 2: 푤푡+1 ≤ 푤푡 + 1 • Proof: If mistake on a positive example 푥 2 2 2 2 푇 푤푡+1 = 푤푡 + 푥 = 푤푡 + 푥 + 2푤푡 푥 Analysis: putting things together 푇 ∗ 푇 ∗ • Claim 1: 푤푡+1푤 ≥ 푤푡 푤 + 훾 2 2 • Claim 2: 푤푡+1 ≤ 푤푡 + 1 After 푀 mistakes: 푇 ∗ • 푤푀+1푤 ≥ 훾푀 • 푤푀+1 ≤ √푀 푇 ∗ • 푤푀+1푤 ≤ 푤푀+1 1 2 So 훾푀 ≤ √푀, and thus 푀 ≤ 훾 Intuition The correlation gets larger. Could be: • 푤푇 푤∗ ≥ 푤푇푤∗ + 훾 ∗ Claim 1: 푡+1 푡 1. 푤푡+1 gets closer to 푤 2 2 2. 푤푡+1 gets much longer • Claim 2: 푤푡+1 ≤ 푤푡 + 1 Rules out the bad case “2. 푤푡+1 gets much longer” Some side notes on Perceptron History Figure from Pattern Recognition and Machine Learning, Bishop Note: connectionism vs symbolism • Symbolism: AI can be achieved by representing concepts as symbols • Example: rule-based expert system, formal grammar • Connectionism: explain intellectual abilities using connections between neurons (i.e., artificial neural networks) • Example: perceptron, larger scale neural networks Symbolism example: Credit Risk Analysis Example from Machine learning lecture notes by Tom Mitchell Connectionism example Neuron/perceptron Figure from Pattern Recognition and machine learning, Bishop Note: connectionism v.s. symbolism • Formal theories of logical reasoning, grammar, and other higher mental faculties compel us to think of the mind as a machine for rule- based manipulation of highly structured arrays of symbols. What we know of the brain compels us to think of human information processing in terms of manipulation of a large unstructured set of numbers, the activity levels of interconnected neurons. ---- The Central Paradox of Cognition (Smolensky et al., 1992) Note: online vs batch • Batch: Given training data 푥푖, 푦푖 : 1 ≤ 푖 ≤ 푛 , typically i.i.d. • Online: data points arrive one by one • 1. The algorithm receives an unlabeled example 푥푖 • 2. The algorithm predicts a classification of this example. • 3. The algorithm is then told the correct answer 푦푖, and update its model Stochastic gradient descent (SGD) Gradient descent • Minimize loss 퐿 휃 , where the hypothesis is parametrized by 휃 • Gradient descent • Initialize 휃0 • 휃푡+1 = 휃푡 − 휂푡훻퐿 휃푡 Stochastic gradient descent (SGD) • Suppose data points arrive one by one 1 • 퐿 휃 = σ푛 푙(휃, 푥 , 푦 ), but we only know 푙(휃, 푥 , 푦 ) at time 푡 푛 푡=1 푡 푡 푡 푡 • Idea: simply do what you can based on local information • Initialize 휃0 • 휃푡+1 = 휃푡 − 휂푡훻푙(휃푡, 푥푡, 푦푡) Example 1: linear regression 1 • Find 푓 푥 = 푤푇푥 that minimizes 퐿 푓 = σ푛 푤푇푥 − 푦 2 푤 푤 푛 푡=1 푡 푡 1 • 푙 푤, 푥 , 푦 = 푤푇푥 − 푦 2 푡 푡 푛 푡 푡 2휂 • 푤 = 푤 − 휂 훻푙 푤 , 푥 , 푦 = 푤 − 푡 푤푇푥 − 푦 푥 푡+1 푡 푡 푡 푡 푡 푡 푛 푡 푡 푡 푡 Example 2: logistic regression • Find 푤 that minimizes 1 1 퐿 푤 = − log휎(푤푇푥 ) − log[1 − 휎 푤푇푥 ] 푛 푡 푛 푡 푦푡=1 푦푡=−1 1 퐿 푤 = − log휎(푦 푤푇푥 ) 푛 푡 푡 푡 −1 푙 푤, 푥 , 푦 = log휎(푦 푤푇푥 ) 푡 푡 푛 푡 푡 Example 2: logistic regression • Find 푤 that minimizes −1 푙 푤, 푥 , 푦 = log휎(푦 푤푇푥 ) 푡 푡 푛 푡 푡 휂 휎 푎 1−휎 푎 푤 = 푤 − 휂 훻푙 푤 , 푥 , 푦 = 푤 + 푡 푦 푥 푡+1 푡 푡 푡 푡 푡 푡 푛 휎(푎) 푡 푡 푇 Where 푎 = 푦푡푤푡 푥푡 Example 3: Perceptron • Hypothesis: 푦 = sign(푤푇푥) • Define hinge loss 푇 푙 푤, 푥푡, 푦푡 = −푦푡푤 푥푡 핀[mistake on 푥푡] 푇 퐿 푤 = − 푦푡푤 푥푡 핀[mistake on 푥푡] 푡 푤푡+1 = 푤푡 − 휂푡훻푙 푤푡, 푥푡, 푦푡 = 푤푡 + 휂푡푦푡푥푡 핀[mistake on 푥푡] Example 3: Perceptron • Hypothesis: 푦 = sign(푤푇푥) 푤푡+1 = 푤푡 − 휂푡훻푙 푤푡, 푥푡, 푦푡 = 푤푡 + 휂푡푦푡푥푡 핀[mistake on 푥푡] • Set 휂푡 = 1. If mistake on a positive example 푤푡+1 = 푤푡 + 푦푡푥푡 = 푤푡 + 푥 • If mistake on a negative example 푤푡+1 = 푤푡 + 푦푡푥푡 = 푤푡 − 푥 Pros & Cons Pros: • Widely applicable • Easy to implement in most cases • Guarantees for many losses • Good performance: error/running time/memory etc. Cons: • No guarantees for non-convex opt (e.g., those in deep learning) • Hyper-parameters: initialization, learning rate Mini-batch • Instead of one data point, work with a small batch of 푏 points (푥푡푏+1,푦푡푏+1),…, (푥푡푏+푏,푦푡푏+푏) • Update rule 1 휃 = 휃 − 휂 훻 푙 휃 , 푥 , 푦 푡+1 푡 푡 푏 푡 푡푏+푖 푡푏+푖 1≤푖≤푏 • Other variants: variance reduction etc. Homework Homework 1 • Assignment online • Course website: http://www.cs.princeton.edu/courses/archive/spring16/cos495/ • Piazza: https://piazza.com/princeton/spring2016/cos495 • Due date: Feb 17th (one week) • Submission • Math part: hand-written/print; submit to TA (Office: EE, C319B) • Coding part: in Matlab/Python; submit the .m/.py file on Piazza Homework 1 • Grading policy: every late day reduces the attainable credit for the exercise by 10%. • Collaboration: • Discussion on the problem sets is allowed • Students are expected to finish the homework by himself/herself • The people you discussed with on assignments should be clearly detailed: before the solution to each question, list all people that you discussed with on that particular question..
Details
-
File Typepdf
-
Upload Time-
-
Content LanguagesEnglish
-
Upload UserAnonymous/Not logged-in
-
File Pages33 Page
-
File Size-