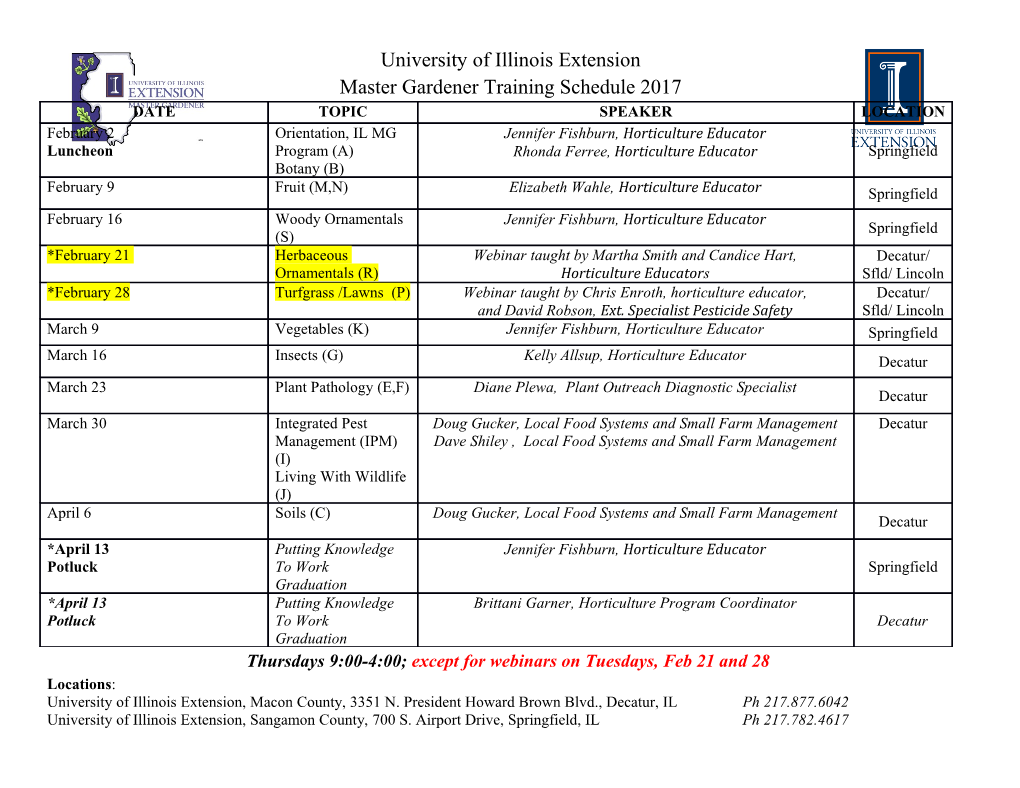
Statistical and machine learning methods to analyze large-scale mass spectrometry data MATTHEW THE Doctoral Thesis Stockholm, Sweden 2018 School of Engineering Sciences in Chemistry, Biotechnology and Health TRITA-CBH-FOU-2018:45 SE-171 21 Solna ISBN 978-91-7729-967-7 SWEDEN Akademisk avhandling som med tillstånd av Kungl Tekniska högskolan framlägges till offentlig granskning för avläggande av Teknologie doktorsexamen i bioteknologi onsdagen den 24 oktober 2018 klockan 13.00 i Atrium, Entréplan i Wargentinhuset, Karolinska Institutet, Solna. © Matthew The, October 2018 Tryck: Universitetsservice US AB iii Abstract Modern biology is faced with vast amounts of data that contain valuable in- formation yet to be extracted. Proteomics, the study of proteins, has repos- itories with thousands of mass spectrometry experiments. These data gold mines could further our knowledge of proteins as the main actors in cell pro- cesses and signaling. Here, we explore methods to extract more information from this data using statistical and machine learning methods. First, we present advances for studies that aggregate hundreds of runs. We introduce MaRaCluster, which clusters mass spectra for large-scale datasets using statistical methods to assess similarity of spectra. It identified up to 40% more peptides than the state-of-the-art method, MS-Cluster. Further, we accommodated large-scale data analysis in Percolator, a popular post- processing tool for mass spectrometry data. This reduced the runtime for a draft human proteome study from a full day to 10 minutes. Second, we clarify and promote the contentious topic of protein false discovery rates (FDRs). Often, studies report lists of proteins but fail to report protein FDRs. We provide a framework to systematically discuss protein FDRs and take away hesitance. We also added protein FDRs to Percolator, opting for the best-peptide approach which proved superior in a benchmark of scalable protein inference methods. Third, we tackle the low sensitivity of protein quantification methods. Current methods lack proper control of error sources and propagation. To remedy this, we developed Triqler, which controls the protein quantification FDR through a Bayesian framework. We also introduce MaRaQuant, which proposes a quantification-first approach that applies clustering prior to identification. This reduced the number of spectra to be searched and allowed us to spot unidentified analytes of interest. Combining these tools outperformed the state-of-the-art method, MaxQuant/Perseus, and found enriched functional terms for datasets that had none before. iv Sammanfattning Modern molekylärbiologi genererar enorma mängder data, som kräver sofisti- kerade algoritmer för att tolkas. Inom området proteomik, det vill säga det storskaliga studier av proteiner, har det under årens lopp byggt upp stora, lättåtkomliga arkiv med data från masspektrometri-experiment. Vidare, finns det fortfarande många outforskade aspekter av proteiner, som är extra in- tressanta eftersom proteiner utgör de viktigaste aktörerna i cellprocesser och cellsignalering. I den här avhandlingen undersöker vi metoder för att utvinna mer information från tillgängliga data med hjälp av statistik och maskinin- lärningsmetoder. För det första, presenterar vi framsteg för att kunna hantera analyser av stu- dier av sammanslagna mängder med hundratals aggregerade körningar. Vi beskriver MaRaCluster, en ny metod för klustring av masspektra som använ- der statistiska metoder för att bedöma likheten mellan masspektra. Metoden identifierar upp till 40% fler peptider än den etablerade metoden, MS-Cluster. Dessutom har vi lagt till funktioner till Percolator, den populära mjukvaran för efterbehandling av masspektrometridata. Detta minskade exekveringsti- den för en storskalig studie av det mänskliga proteomet från ett dygn ner till 10 minuter på en standarddator. För det andra, förtydligar vi och förespråkar användandet av ett mått på osäkerhet i identifiering av protein från masspektrometridata, det så kallade False Discovery Rate (FDR) på proteinnivå. Många studier rapporterar inte FDR på proteinnivå, trots att dessa väljer att redovisa sina resultat i form av listor av proteiner. Här presenterar vi ett statistiskt ramverk för att kunna systematiskt diskutera FDR på proteinnivå och för att undanröja tveksam- heter. Vi jämför skalbara inferensmetoder för proteiner och inkluderade den bästa-peptidinferensmetoden i Percolator. För det tredje, tar vi upp bristen på känslighet för proteinkvantifieringsme- toder. Nuvarande metoder saknar ordentlig kontroll av felkällor och felfort- plantning. För att åtgärda detta utvecklade vi ett nytt verktyg, Triqler, som tillhandahåller adekvat falsk upptäcktshastighetsstyrning för proteinkvanti- fiering genom ett Bayesiska ramverk. Vi beskriver också MaRaQuant, där vi introducera kvantifiering-först-metoden som tillämpar klustring före iden- tifiering. Detta minskade antalet spektra som behövde matchas, men tillät oss också att upptäcka oidentifierade intressanta analyter. Kombinationen av dessa två verktyg överträffade den etablerade proteinkvantifieringsmetoden, MaxQuant/Perseus, och hittade anrikning av funktionella anteckningstermer för dataset där inget hittades tidigare. Contents Contents v Acknowledgments 1 List of publications 3 1 Introduction 5 1.1 Thesis overview . 5 2 Biological background 7 2.1 From DNA to proteins . 7 2.2 Proteins . 9 2.3 Current state of research . 10 3 Shotgun proteomics 13 3.1 Protein digestion . 13 3.2 Liquid chromatography . 14 3.3 Tandem mass spectrometry . 14 4 Data analysis pipeline 21 4.1 Feature detection . 21 4.2 Peptide identification . 22 4.3 Protein identification . 24 4.4 Protein quantification . 25 5 Statistical evaluation 27 5.1 Terminology . 27 5.2 False discovery rates . 28 5.3 The Percolator software package . 33 6 Mass spectrum clustering 35 6.1 Distance measures . 36 6.2 Clustering algorithms . 38 v vi CONTENTS 6.3 Evaluating clustering performance . 40 7 Label-free protein quantification 41 7.1 Feature detection . 41 7.2 Peptide quantification . 43 7.3 Matches-between-runs . 43 7.4 Missing value imputation . 44 7.5 Peptide to protein summarization . 44 7.6 Differential expression analysis . 44 8 Present investigations 47 8.1 Paper I: MaRaCluster . 47 8.2 Paper II: Protein-level false discovery rates . 48 8.3 Paper III: Percolator 3.0 . 48 8.4 Paper IV: Triqler . 49 8.5 Paper V: MaRaQuant . 49 9 Future perspectives 51 Bibliography 53 Acknowledgements I owe many thanks to all the people who have made all the work in my Ph.D. and this thesis possible. First of all, none of this would have been possible without my marvelous supervisor, Lukas. Your support, optimism and never-ending stream of suggestions made this journey an immensely positive experience. I would also like to thank my collaborators, William Stafford Noble, Fredrik Edfors, Johannes Griss, Yasset Perez-Riverol and Juan Antonio Vizcaíno, as well as the OpenMS crew for their help and comradery, Timo Sachsenberg, Julianus Pfeuffer, Leon Bichmann and Oliver Alka. A special thanks to Oliver Serang, for all your fascinating stories and planting the Bayesian seed, and Wout Bittremieux, for your companionship all around the globe. All the people who have passed through the Käll lab over the years. Viktor and Luminita, for providing me with a warm welcome to the group, no matter how short it was. David, Heydar, Xuanbin, Ayesha, Sara, Daniel, Yunyi, Alicia, Revant, Aslıand Yang for all the good times in- and outside the lab. Jorrit, for injecting some “Hollandse nuchterheid” every so often. Yrin, for all the amusing discussions and board game evenings, go finish those degrees! Vital, for making the office an enjoyable place to come to. Richard, for sharing all your kooky ideas and stories. Gustavo, for your contagious good mood, stimulating conversations and for always being up for hot pot and a beer (or two). Patrick, for your infectious high-energy can-do attitude, go apply that to the mess I left you! To everyone at Gamma 6 for all the enjoyable lunches, fikas and after work activi- ties, Mattias, Linus, Pekka, Ikram, Owais, Mehmood, Auwn, Hashim, Mohammad, Hazal, Seong, Jovana, Andrei, Parul, Johannes, Ilaria, Daniel, Yue and Mandi. Also to all of you from SciLifeLab, you are simply too many to name, but in par- ticular thanks to Johannes, for being my TA buddy and all-round good company, and to Wenjing, Marcel and Fran, for providing me with a second group when our numbers were particularly low and all the times after that as well. To my friends that I was fortunate enough of meeting here in Stockholm, Steffen, Lisa, Lukas, Thomas, Nicole and Dmitry, and also my Berlin Master/Ph.D. buddies, Luis, Carlos and Xiao, for all the comradery and good times. 1 2 Acknowledgments A big thanks as well to my family and friends in the Netherlands and extended family in Denmark. In particular, my parents, for all your continuous support and for always showing great interest in my work. And last but definitely not least, Ditte, for all your encouragements, patience and affection, I would not have come so far today if it wasn’t for you. List of publications I have included the following articles in the thesis. PAPER I: MaRaCluster: A fragment rarity metric for clustering frag- ment spectra in shotgun proteomics. Matthew The & Lukas Käll Journal of Proteome Research, 15(3), 713-720 (2016). DOI: 10.1021/acs.jproteome.5b00749 PAPER II: How to talk about protein-level false discovery rates in shot- gun proteomics. Matthew The, Ayesha Tasnim & Lukas Käll Proteomics,
Details
-
File Typepdf
-
Upload Time-
-
Content LanguagesEnglish
-
Upload UserAnonymous/Not logged-in
-
File Pages70 Page
-
File Size-