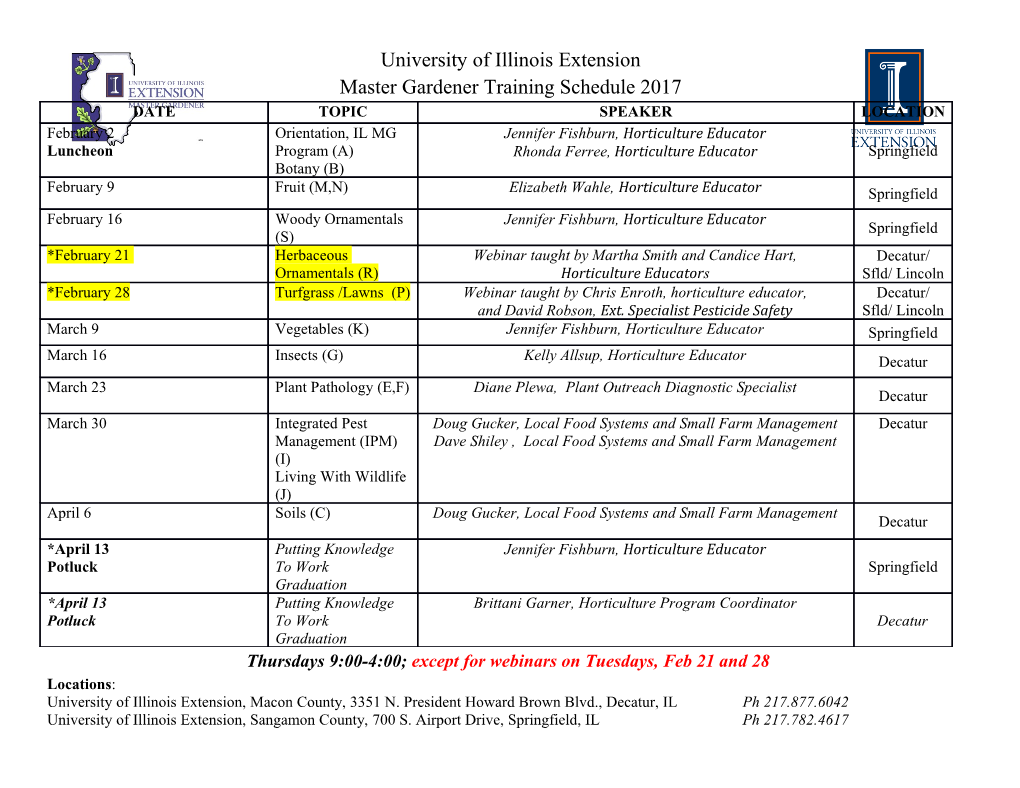
Belle II Starterkit MVA Tutorial Belle II Starterkit Wehle, Simon BNL, 01.08.2019 Disclaimer Personal Experience • Additional resources • The presented recommendations originate purely from • General lecture on multivariate analysis by Thomas Keck personal experiences, you might be of different opinion MultivariateClassificationLecture.pdf and are encouraged to share and discuss them • (A lot of distribution plots in this talk are taken from this reference) Context of this talk • Confluence, MVA package • Focus on machine learning for classification • Presentation of various methods • More on the packages to come • Presentation of available tools • The Belle II MVA package • Personal recommendations | MVA Tutorial | Simon Wehle, 3.2.2018 !2 Impact of Machine Learning Era of data driven methods • Huge boost in efficiency • Rapid developments due to efforts of billion dollar companies • Many open source machine learning platforms forming Prospects for Physics • Improvements due to machine learning can correspond to years of running an experiment • In my opinion we might be just scratch at the beginning of what is possible for our field | MVA Tutorial | Simon Wehle, 3.2.2018 !3 Multivariate Methods Which and when to use What is the Problem? Where can we use machine learning? Problem Solution • Statistical methods • Machine learning • Classification • Supervised (We have labeled data like a MC • Regression truth) Result • Unsupervised (Can be • Clustering directly applied to data) • Reinforced Learning (Self learned strategy based on rewards) | MVA Tutorial | Simon Wehle, 3.2.2018 !5 Available Methods Focusing on classification mainly Conventional Machine Learning / Statistical Evaluation • Many different methods available, I will list a few one which were/are used in physics • LDA, SVM, Neural Networks, Bayesian Methods, Nearest Neighbors, Decision Trees, Boosting... (but there are of course many more) Deep Learning • Very popular machine learning method right now • Has proven to be extremely efficient and powerful • Many different techniques, Feed forward DNN, Convolutional Neural Network, Recurrent Neural Networks, LSTMs … | MVA Tutorial | Simon Wehle, 3.2.2018 !6 LDA – Linear Discriminant Analysis Apparently do not call it Fisher’s discriminant anymore Linear Discriminant Analysis • Commonly used under the name Fisher’s Discriminant • Only requires mean and covariance of the sample • Can be expanded to Quadratic Discriminant Analysis | MVA Tutorial | Simon Wehle, 3.2.2018 !7 Decision Trees Decision Trees • Simple to comprehend • Easy to train and implement • Reasonably powerful | MVA Tutorial | Simon Wehle, 3.2.2018 !8 Boosted Decision Trees Very good Boosting • Use may ”weak” classifiers • Reweight events according to prediction of previous tree(s) for Robust the next tree to focus on falsely Good classified events Stochastic Boosted Decision Trees Bagging • Use only a fraction of events in each tree • Robustness against statistical Stochastic Gradient Decision Trees fluctuations • Widely used in HEP • Robustness against overfitting • Out of the box very good performance | MVA Tutorial | Simon Wehle, 3.2.2018 !9 Artificial Neural Networks (ANN) Artificial Neural Networks • Attempt to “simulate” neurons in a brain • Usually a feed-forward approach, where data flows from input over hidden layers to the output layer • Nice visualizations made by google to play around with neural networks in the browser: • http://playground.tensorflow.org • ANN without hidden layer is similar to a LDA • A neural network with one hidden layer can can approximate continuous functions on compact subsets of Rn | MVA Tutorial | Simon Wehle, 3.2.2018 !10 Deep Neural Networks (DNN) The glorious future Deep Learning • Basically a large, mostly feed-forward ANN with many hidden layers • Progress in computing power made backpropagation algorithm possible (mostly on gpus) • Can/should be fed with “low level” features • Benefit of DNNs: They can learn high level features themselves • Idea: Give raw data, they figure out how to do a vertex fit or constrain invariant masses.. (okay it does unfortunately not work so easy for us) • The input data has to be adequately complex or the danger of overfitting becomes imminent! | MVA Tutorial | Simon Wehle, 3.2.2018 !11 Convolutional Neural Networks (DNN) The glorious future Convolutional Neural Networks • Used for image recognition • Profit extremely from parallelization in gpus | MVA Tutorial | Simon Wehle, 3.2.2018 !12 Implementation Overview A few notable implementations ROOT TMVA Scikit-Learn Keras • Features many different classifiers • Python (numpy) based framework • Python based “meta” framework for • Ability to perform preprocessing • Many classification, regression, deep learning • Nice overview and comparison of clustering and preprocessing tools • Can use various implementations as methods after training are available backend, like tensorflow or theano • https://root.cern.ch/tmva • http://scikit-learn.org/ • Very simple syntax • keras.io FastBDT Other notable candidates • Used often in the Belle II software • FANN • Tensorflow • Extremely(!) fast and robust • Fast Artificial Neural Network • Generic library for large matrix/ tensor operation • https://github.com/thomaskeck/ • http://fann.sourceforge.net/fann.pdf FastBDT • Optimized for gpu usage • XGBoost • https://www.tensorflow.org/ • Industry standard GBDT • NeuroBayes | MVA Tutorial | Simon Wehle, 3.2.2018 !13 Belle II MVA Package Overview of the package Belle II MVA Package Overview Subheading, optional | MVA Tutorial | Simon Wehle, 3.2.2018 !15 Belle II MVA Package Overview Goals and existing use cases Main goals Use cases • The mva package was introduced to provide: • Analysis: Full Event Interpretation, over 100 classifiers • Provides tools to integrate mva methods in BASF2 have to be trained without user interaction and have to be loaded from the database automatically if a user wants to • Collection of examples for basic and advanced mva apply the FEI to MC or data usages • Analysis: Flavor Tagger • Backend independent evaluation and validation tools • Analysis: Continuum Suppression • The mva package is NOT: • Tracking: Track finding in CDC and VXD, classifiers should • Yet another mva framework → Avoids reimplementing be retrained automatically using the newest MC, maybe existing functionality even run-dependent, and automatically loaded and applied • A wrapper around existing mva frameworks → Tries to on MC and data during the reconstruction phase avoid artificial restrictions • ECL: Classification and regression tasks • BKLM: KLong ID | MVA Tutorial | Simon Wehle, 3.2.2018 !16 Belle II MVA – Setting up a Training Subheading, optional Interface GlobalOptions import basf2_mva • Fitting and Inference go = basf2_mva.GeneralOptions() • basf2_mva_teacher go.m_datafiles = basf2_mva.vector("train.root") • basf2_mva_expert go.m_treename = "tree" • Condition database go.m_identifier = "Identifier" • basf2_mva_upload go.m_variables = basf2_mva.vector('p', 'pz', 'M') go.m_target_variable = "isSignal" • basf2_mva_download • basf2_mva_available • Evaluation SpecificOptions • basf2_mva_evaluate.py • basf2_mva_info sp = basf2_mva.FastBDTOptions() • basf2_mva_extract sp.m_nTrees = 100 sp.m_shrinkage = 0.2 fastbdt_options.m_nLevels = 3 | MVA Tutorial | Simon Wehle, 3.2.2018 !17 Belle II MVA – How to Perform a Training Perform a training using basf_mva Training in Python Training in Shell import basf2_mva basf2_mva_teacher --datafiles train.root --treename tree go = basf2_mva.GeneralOptions() --identifier \ go.m_datafiles = DatabaseIdentifier basf2_mva.vector("train.root") --variables p pz M go.m_treename = "tree" --target_variable go.m_identifier = "DatabaseIdentifier" isSignal go.m_variables = --method FastBDT basf2_mva.vector('p', 'pz', 'M') go.m_target_variable = "isSignal" basf2_mva_teacher –help sp = basf2_mva.FastBDTOptions() basf2_mva_teacher –method FastBDT –help basf2_mva.teacher(go, sp) | MVA Tutorial | Simon Wehle, 3.2.2018 !18 Belle II MVA – Evaluate the Training Apply method in basf2 Validate the training on command line basf2_mva_evaluate.py -id DatabaseIdentifier -train train.root -data test.root -o validation.pdf | MVA Tutorial | Simon Wehle, 3.2.2018 !19 Belle II MVA – How to apply a Training Apply method in basf2 Apply method in basf2 path.add_module('MVAExpert', listNames=['D0'], extraInfoName='Test', identifier='DatabaseIdentifier') | MVA Tutorial | Simon Wehle, 3.2.2018 !20 Belle II MVA – Applying Expertise to Data Apply method in shell basf2_mva_expert --identifiers DatabaseIdentifier --datafiles test.root --treename tree --outputfile expert.root Apply method in python import basf2_mva basf2_mva.expert(basf2_mva.vector('DatabaseIdentifier'), basf2_mva.vector('test.root'), 'tree', 'expert.root') | MVA Tutorial | Simon Wehle, 3.2.2018 !21 Belle II MVA – Further Information Please consider further information on confluence and the examples $BELLE2_RELEASE_DIR/mva/examples/ MVA Package on Confluence | MVA Tutorial | Simon Wehle, 3.2.2018 !22 Machine Learning Good Practice (Pure personal recommendations) Evaluate your Training! One or the most important aspects! How to evaluate a training • One can compare trainings and different methods on the same data with the ROC curve • Often (but not always) a training with higher area under the curve (AUC) performs better • Compare both training data and test data performance to spot overfitting Always use an independent control sample ! | MVA Tutorial | Simon Wehle, 3.2.2018 !24 Evaluate your
Details
-
File Typepdf
-
Upload Time-
-
Content LanguagesEnglish
-
Upload UserAnonymous/Not logged-in
-
File Pages40 Page
-
File Size-