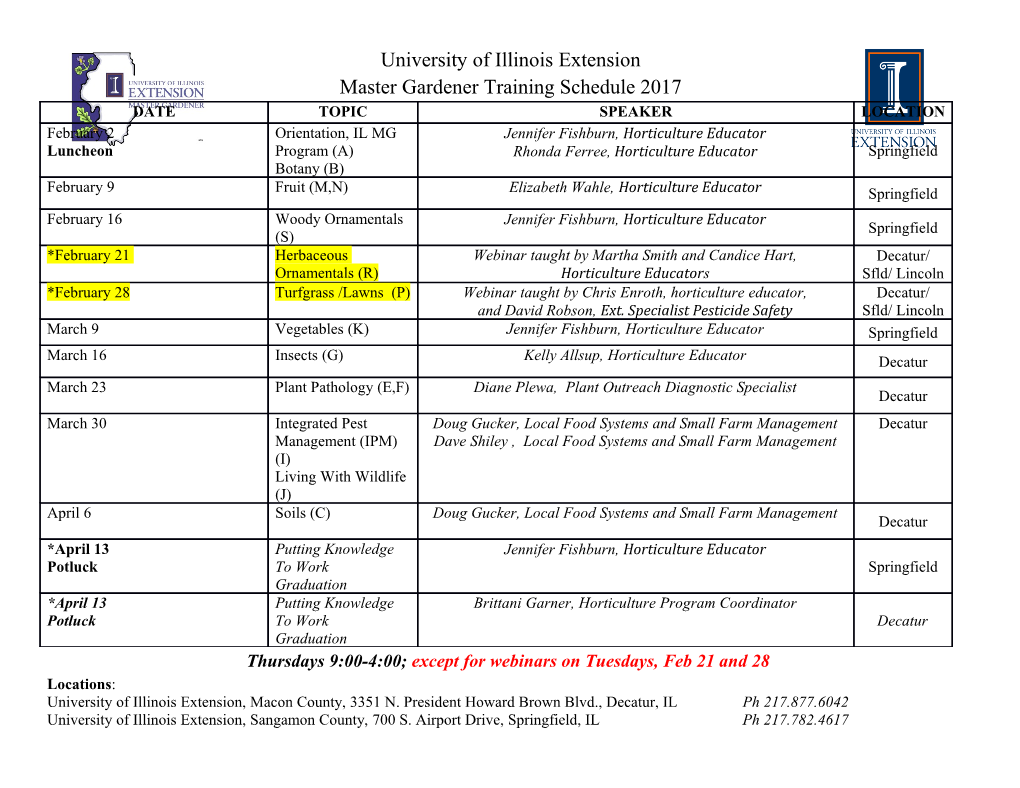
Technical white paper Location Intelligence Location Intelligence for Big Data Maximizing the distributed nature of big-data clusters to achieve breakthrough performance Page 2 Add agility to big data analysis. Companies struggle to generate positive returns on big data implementations. Many find it difficult to generate actionable insight from their big data assets. Geospatial processing changes the dynamics. Location Intelligence for Big Data makes vast quantities of data consumable using GeoEnrichment and location analytics. Run spatial operations within a native environment. Then visualize relationships in a spatial context to improve analysis and decision making. Pitney Bowes offers a unique approach, embedding location technology within big data solutions. When you embed Discover the technology that delivers richer insights and a faster ROI. rather than connect, you can interpret • High scalability, high speed transactional data faster and resolve data processing critical business issues with the clarity • GeoEnrichment you need. • Cluster-level data partitioning • Node-level data processing Location Intelligence for Big Data A Pitney Bowes technical white paper Page 3 The big data challenge Big data technologies increasingly allow companies to The Location Intelligence advantage store and process incredibly large datasets of customer Location Intelligence brings critical perspective to data calls, financial transactions and social media feeds. analysis. Big data typically comes with a locational Yet, many companies struggle to generate meaningful, component. This could be a customer address, a mobile actionable insights. Key performance indicators remain phone GPS signal, the location of an ATM, a store elusive as data volume and velocity continue to grow. transaction or a social media check-in. The challenge is to connect data within and across Through GeoEnrichment, a process for appending datasets in a way that: location-based ancillary data, organizations can augment records with latitude/longitude coordinates. Then, that • Ensures accuracy and precision coordinate data can be used to integrate additional context into every record. • Enables enrichment With this enriched embedded insight: • Keeps pace with the extraordinary speed and scale required • Rules-based workflows can utilize this appended data to automate business decisions. • Spatial aggregation can condense data volumes, making them more manageable. • Data can be generalized in a spatial context for results that are easier to visualize and analyze. • Organizations can gain new perspectives into business drivers and subsequent company responses. Valuable applications An embedded approach enables companies to formulate business questions and solve them within a big data environment. For example: Telecommunications companies continually Financial services firms continually process an process a huge number of call records. These can incredibly vast number of transactions. Each can be be condensed and presented via highly accurate, appended with a latitude/longitude coordinate pair, near real-time coverage maps. This type of visual and operational rules can help determine when to flag analysis helps firms to improve customer service, records as potentially fraudulent. This process enables reduce churn, market more effectively and gain financial services firms to provide better safeguards to market share. consumers and their privacy, while helping to reduce losses due to fraud. Page 4 The benefits of a native optimized approach Innovative, modular technology Many geospatial technology providers supply solutions The Pitney Bowes Location Intelligence for Big Data that connect to big data platforms, then transfer data from solution is comprised of location technology software these distributed platforms into their own GIS server-based development kits (SDKs) that allow companies to technology. The disadvantage of this “connector” approach GeoEnrich datasets and spatially aggregate results, is that it doesn’t leverage the processing power of the condensing big data into a consumable output. It also distributed platform (Hadoop, Spark, etc.). Instead, the includes APIs and data. actual geospatial operations occur in a single server or a small server cluster, which limits your ability to process • Our Java-based SDKs can be transferred into any big data large data sets. environment, such as Hadoop or Spark, so companies are not limited by their technology choices in a transient and Pitney Bowes takes a different, big-data-ready approach. evolving field. To maximize the capabilities of distributed processing environments, we enable geospatial operations to run • We offer 350+ datasets that can be used to add spatial natively within a variety of distributed platforms. context, serve as a container for aggregation, and be analyzed and visualized using our web-based mapping Let’s look first at the technology we provide; then at the technologies. process steps that enable it to work natively in a big data environment. Examples of performance achieved via the Pitney Bowes native and optimized technology strategy These are actual numbers achieved in our clients’ use cases. We are continually improving the technology and can achieve much better performance using newer technologies like Spark. US Parcel Centroid geocoding of 106 Geocoding 30 minutes 5 nodes Hadoop cluster million addresses 1 billion mobile points spatially joined “Find the Nearest” spatial join 36 minutes 20 nodes EMR on AWS to 12 million points of interest Aggregated 19 billion mobile call Point-in- polygon processing 30 minutes records to 950 million polygons 56 node Hadoop cluster Pitney Bowes Location Intelligence for Big Data capabilities Location Intelligence SDK Global Geocoding API • Takes spatial primitives (points, lines and polygons) and applies a • Geocoding turns a street address, place or point of interest geometric function (“contains”, “combine”, “intersects” etc.) using into a latitude, longitude co-ordinate pair. additional spatial and aspatial data • Reverse geocoding take the co-ordinate and gives a street • Enables creation of spatial query such as “Aggregate point data address, or administrative boundary. within this polygon” or “Find the nearest point to this line” • Can be used to GeoEnrich a dataset by appending additional Routing SDK attributes using customer data, third-party data or any of the 350+ • Takes a known location (e.g. retail store) and uses the road datasets in the Pitney Bowes Global Data Catalog network to derive information such as equal drive times (isochrones) around that point, or the shortest path to • U.S. customers may also choose to utilize the pre-enriched Pitney that point. Bowes Master Location Database (MLD) assets for U.S. postal addresses to further improve processing speeds and location accuracy for operational workflows Location Intelligence for Big Data A Pitney Bowes technical white paper Page 5 Putting our technology to work The diagram to the right uses the Global Geocoding Jar API to illustrate this architecture as well as how it can MR/Yarn File Application be used in various big data related processes. It shows Hadoop NameNode how Pitney Bowes integrates geocoding capabilities Geocoding natively into Hadoop. SDK The key components of the solution are the Global Geocoding API (GGA) and geocoding dictionary files. • GGA is a collection of Jar files that can be used in writing Java based MapReduce, Yarn or Spark Geocoding Geocoding Geocoding applications. Dictionary Dictionary Dictionary • The geocoding dictionary data files can be pre- installed in all data nodes of the Hadoop cluster or Data Node Data Node Data Node distributed into the cluster dynamically before use. Input Data Output Data Running geocoding within Hadoop Making it more accessible Listed below is an example of how Pitney Bowes can run While a MapReduce batch job works well for users with a geocoding as a Hadoop MapReduce batch job in command data-engineering background, it is not user-friendly for line. Note that the user can set up different geocoding other data analysts. To make geocoding in Hadoop more parameters in the config.xml, such as the dictionary to use accessible, we’ve developed a HIVE geocoding UDF so any and the fields to return. Both forward geocoding and user with a SQL background can use it in Hadoop. Most reverse geocoding is supported. Pitney Bowes Location Intelligence capabilities can be deployed in Hadoop or Spark using an approach similar to the geocoding example above. [jun@osboxes ~]$: hadoop jar Geocoding_Hadoop.jar com.pb.mr.GeocodingDriver -input /addressdatafolder -output /geocoderesult -appConfig Geocode_config.xml HIVE> select geocode (street, city, state, zip, ‘USA’) from customersAddTable; Page 6 Optimizing geospatial data processing Breaking down the Pitney Bowes approach in Hadoop and Spark Data preparation is critical to a highly performant spatial Geospatial data processing is a fundamental step in process. This requires enhancements to both the spatial almost all location related big data applications. For data partition at the cluster level and spatial data example, to analyze users’ mobile records with GPS processing in node level. locations, ancilliary data is added to provide context, such as the individual’s address or a nearby point of Cluster-level data partitioning dictates how large interest. To enable this GeoEnrichment processes at datasets are divided so they can be efficiently processed scale, a set of highly efficient
Details
-
File Typepdf
-
Upload Time-
-
Content LanguagesEnglish
-
Upload UserAnonymous/Not logged-in
-
File Pages11 Page
-
File Size-