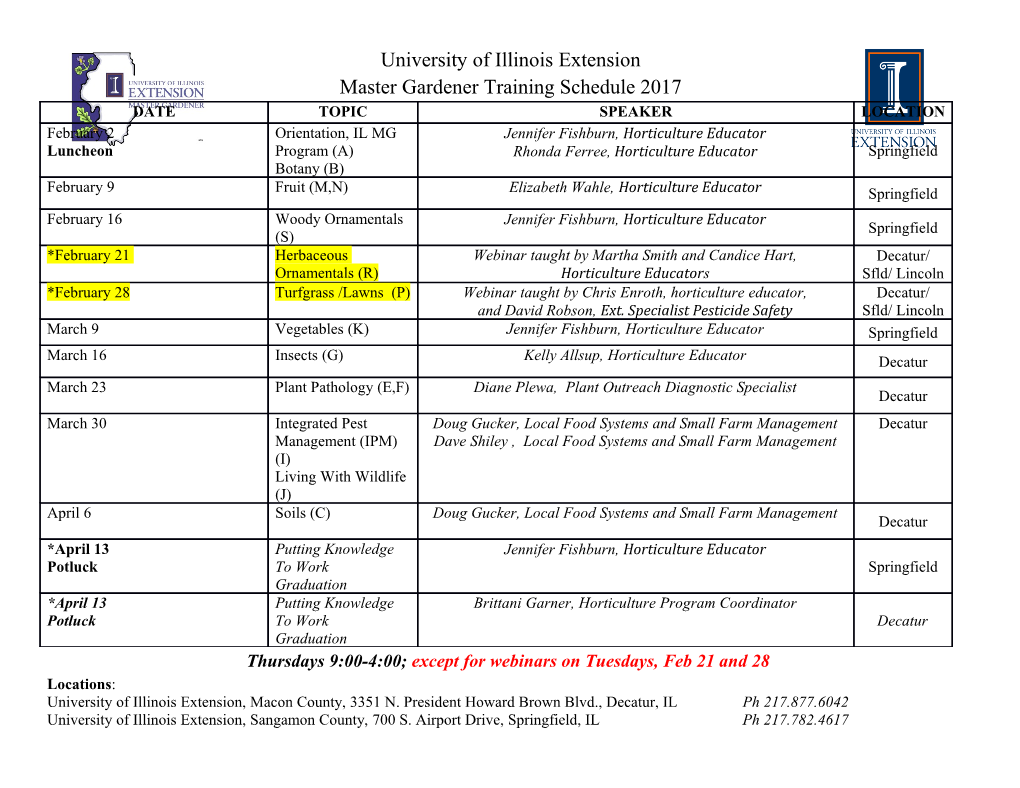
Learn About Multiple Regression With Dummy Variables in SPSS With Data From the General Social Survey (2012) © 2015 SAGE Publications, Ltd. All Rights Reserved. This PDF has been generated from SAGE Research Methods Datasets. SAGE SAGE Research Methods Datasets Part 2015 SAGE Publications, Ltd. All Rights Reserved. 1 Learn About Multiple Regression With Dummy Variables in SPSS With Data From the General Social Survey (2012) Student Guide Introduction This dataset example introduces readers to multiple regression with dummy variables. Multiple regression allows researchers to evaluate whether a continuous dependent variable is a linear function of two or more independent variables. When one (or more) of the independent variables is a categorical variable, the most common method of properly including them in the model is to code them as dummy variables. Dummy variables are dichotomous variables coded as 1 to indicate the presence of some attribute and as 0 to indicate the absence of that attribute. The multiple regression model is most commonly estimated via ordinary least squares (OLS), and is sometimes called OLS regression. This example describes multiple regression with dummy variables, discusses the assumptions underlying it, and shows how to estimate and interpret such models. We use a subset of data from the 2012 General Social Survey (http://www3.norc.org/GSS+Website/). It presents an analysis of whether a person’s weight is a linear function of a number of attributes, including whether or not the person is female and whether or not the person smokes cigarettes. Weight, and particularly being overweight, is associated with a number of negative Page 2 of 15 Learn About Multiple Regression With Dummy Variables in SPSS With Data From the General Social Survey (2012) SAGE SAGE Research Methods Datasets Part 2015 SAGE Publications, Ltd. All Rights Reserved. 1 health outcomes. Thus, results from an analysis like this could therefore have implications for individual behavior and public health policy. What Is Multiple Regression With Dummy Variables? Multiple regression expresses a dependent, or response, variable as a linear function of two or more independent variables. Readers looking for a general introduction to multiple regression should refer to the appropriate examples in Sage Research Methods. This example focuses specifically on including dummy variables among the independent variables in a multiple regression model. Many times, an independent variable of interest is categorical. "Gender" might be coded as Male or Female; "Region" might be coded as South, Northeast, Midwest, and West. When there is no obvious order to the categories or when there are three or more categories and differences between them are not all assumed to be equal, such variables need to be coded as dummy variables for inclusion into a regression model. The number of dummy variables you will need to capture a categorical variable will be one less than the number of categories. Thus, for gender, we only need one dummy variable, maybe coded "1" for Female and "0" for Male. For region, we would need three, which might look like this: • northeast: coded "1" if from the Northeast and "0" otherwise. • south: coded "1" if from the South and "0" otherwise. • midwest: coded "1" if from the Midwest and"0" otherwise. We always need one less than the number of categories because the last one would be perfectly predicted by the others. For example, if we know that northeast, south, and midwest all equal zero, then the observation must be from the West. Multiple regression models are typically estimated via Ordinary Least Squares Page 3 of 15 Learn About Multiple Regression With Dummy Variables in SPSS With Data From the General Social Survey (2012) SAGE SAGE Research Methods Datasets Part 2015 SAGE Publications, Ltd. All Rights Reserved. 1 (OLS). OLS produces estimates of the intercept and slopes that minimize the sum of the squared differences between the observed values of the dependent variable and the values predicted based on the regression model. When computing formal statistical tests, it is customary to define the null hypothesis (H0) to be tested. In multiple regression, the standard null hypothesis is that each coefficient is equal to zero. The actual coefficient estimates will not be exactly equal to zero in any particular sample of data simply due to random chance in sampling. The t-tests conducted to test each coefficient are designed to help us determine if the coefficient estimates are different enough from zero to be declared statistically significant. "Different enough" is typically defined as a test statistic with a level of statistical significance, or p-value, of less than 0.05. This would lead us to reject the null hypothesis (H0) that a coefficient estimate equals zero. Estimating a Multiple Regression With Dummy Variables Model To make this example easier to follow, we will focus on estimating a model with just two independent variables, in this case, labeled X and D. Let’s further assume that X is a continuous variable while D is a dummy variable coded 1 if the observation has the characteristic associated with D and coded 0 if it does not. The multiple regression model with two independent variables can be defined as in Equation 1: (1) Yi = β0 + β1Xi + β2Di + εi Where: • Yi = individual values of the dependent variable • Xi = individual values of the continuous independent variable Page 4 of 15 Learn About Multiple Regression With Dummy Variables in SPSS With Data From the General Social Survey (2012) SAGE SAGE Research Methods Datasets Part 2015 SAGE Publications, Ltd. All Rights Reserved. 1 • Di = individual values of the dummy independent variable • β0 = the intercept, or constant, associated with the regression line • β1 = the coefficient operating on the continuous independent variable • β2 = the coefficient operating on the dummy independent variable • ε = the unmodeled random, or stochastic, component of the dependent variable; often called the error term or the residual of the model. Researchers have values for Yi, Xi, and Di in their datasets – they use OLS to estimate values for β0, β1, and β2. The coefficients β1 and β2 are often called partial slope coefficients, or partial regression coefficients, because they represent the unique independent effect of the corresponding independent variable on the dependent variable after accounting for, or controlling for, the effects of the other independent variables in the model. Equation 2 can be used to estimate the coefficient operating on the first independent variable. The same equation can be altered to estimate as well. (2) 2 x y × d − d y × x d ^ ∑ ( i)( i) ∑ ( i) ∑ ( i)( i) ∑ ( i)( i) β1 = 2 2 2 ∑ (xi) × ∑ (di) − ∑ (xi)(di) Where: ^ • β1 = the estimated value of the coefficient operating on X1 ¯ • yi = Yi − Y ¯ • Y = the sample mean of the dependent variable ¯ • xi = Xi − X ¯ • X = the sample mean of the continuous independent variable Page 5 of 15 Learn About Multiple Regression With Dummy Variables in SPSS With Data From the General Social Survey (2012) SAGE SAGE Research Methods Datasets Part 2015 SAGE Publications, Ltd. All Rights Reserved. 1 ¯ • di = Di − D ¯ • D = the sample mean of the dummy independent variable. The numerator of Equation 2 is based on the product of deviations in X from its mean and deviations of Y from its mean. The sum of these products will determine whether the slope is positive, negative, or near zero. The numerator also accounts for the shared association between D and Y as well as the correlation between D and X. The denominator in Equation 2 adjusts the estimate of βi to account for how much variability there is in X and D. The result is that β1, which is the marginal effect of X on Y, captures the unique or independent effect of X on Y after accounting for the presence of D. The same logic applies to computing the estimate of β2. Once both β1 and β2 are computed , Equation 3 can be used to compute the value for the intercept. (3) ^ ¯ ^ ¯ ^ ¯ β0 = Y − β1X − β2D Equation 3 is a simple way to estimate the intercept, β0 . We can use this formula because the OLS regression model is estimated such that it always goes through the point at which the means of X, D, and Y intersect. Note that the formulas presented here are identical to the formulas used for multiple regression generally. The presence of a dummy variable among the independent variables does not change the math of OLS. As noted above, β1 and β2 represent the marginal effect of X and D, respectively, on the expected value of the dependent variable. That means that when X increases by 1 unit, the expected value of Y will change by an amount equal to Page 6 of 15 Learn About Multiple Regression With Dummy Variables in SPSS With Data From the General Social Survey (2012) SAGE SAGE Research Methods Datasets Part 2015 SAGE Publications, Ltd. All Rights Reserved. 1 β1. Similarly, when D increases by 1 unit, the expected value of Y will change by an amount equal to β2. For the continuous independent variable X, a 1-unit increase represents some incremental increase in that variable. It might mean an increase of 1 dollar, 1 thousand dollars, 1 inch, 1 year, and so forth. In contrast, a 1-unit increase in the dummy variable D represents shifting from the absence of some attribute (D = 0) to the presence of that attribute (D = 1). Moving from 0 to 1 constitutes the entire range of D. As a result, the estimate of can be interpreted as the mean difference between observations where D = 0 and observations where D = 1 after accounting for the effects of the other independent variables in the model.
Details
-
File Typepdf
-
Upload Time-
-
Content LanguagesEnglish
-
Upload UserAnonymous/Not logged-in
-
File Pages15 Page
-
File Size-