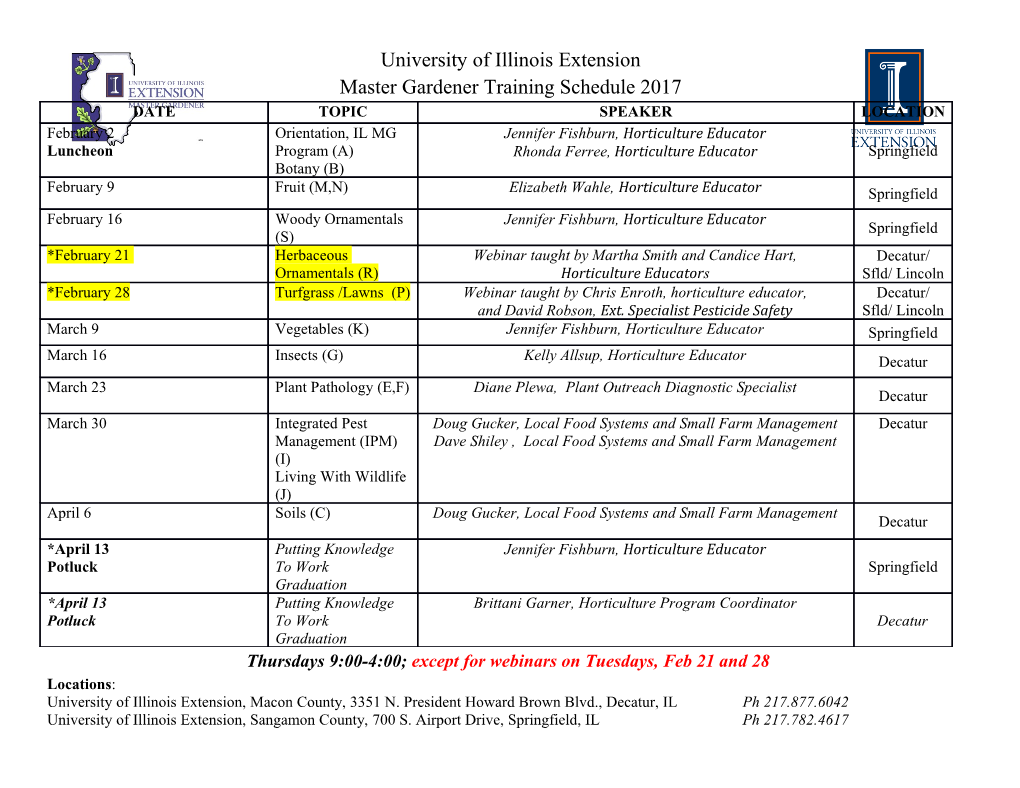
Bias in Machine Learning - What is it Good for? Thomas Hellstrom¨ and Virginia Dignum and Suna Bensch 1,2 Abstract. In public media as well as in scientific publications, the is related to several of the AI-HLEG recommendations for building term bias is used in conjunction with machine learning in many dif- Human-Centered AI systems. ferent contexts, and with many different meanings. This paper pro- Machine learning is a wide research field with several distinct ap- poses a taxonomy of these different meanings, terminology, and def- proaches. In this paper we focus on inductive learning, which is a initions by surveying the, primarily scientific, literature on machine corner stone in machine learning. Even with this specific focus, the learning. In some cases, we suggest extensions and modifications to amount of relevant research is vast, and the aim of the survey is not promote a clear terminology and completeness. The survey is fol- to provide an overview of all published work, but rather to cover the lowed by an analysis and discussion on how different types of biases wide range of different usages of the term bias. are connected and depend on each other. We conclude that there is This paper is organized as follows. Section 2 briefly summarizes a complex relation between bias occurring in the machine learning related earlier work. In Section 3 we survey various sources of bias, pipeline that leads to a model, and the eventual bias of the model as it appears in the different steps in the machine learning process. (which is typically related to social discrimination). The former bias Section 4 contains a survey of various ways of defining bias in the may or may not influence the latter, in a sometimes bad, and some- model that is the outcome of the machine learning process. In Sec- time good way. tion 5 we provide a taxonomy of bias, and discuss the different types of found biases and how they relate to each other. Section 6 concludes the paper. 1 INTRODUCTION Media, as well as scientific publications, frequently report on ‘Bias in 2 RELATED WORK Machine Learning’, and how systems based on AI or machine learn- ing are ‘sexist’ 3 or ‘discriminatory’ 4 [10,37]. In the field of machine A number of reviews, with varying focuses related to bias have been learning, the term bias has an established historical meaning that, at published recently. Barocas and Selbst [3] give as good overview least on the surface, totally differs from how the term is used in typ- of various kinds of biases in data generation and preparation for ical news reporting. Furthermore, even within machine learning, the machine learning. Loftus et al. [31] review a number of both non term is used in very many different contexts and with very many dif- causal and causal notions on fairness, which is closely related to bias. ferent meanings. Definitions are not always given, and if they are, the Suresh and Guttag [45] identify a number of sources of bias in the relation to other usages of the word is not always clear. Furthermore, machine learning pipeline. Olteanu et al. [35] investigate bias and us- definitions sometimes overlap or contradict each other [8]. age of data from a social science perspective. Our analysis is comple- The main contribution of this paper is a proposed taxonomy of mentary to the work cited above, by focusing on bias in conjunction the various meanings of the term bias in conjunction with machine with machine learning, and by examining a wider range of biases. learning. When needed, we suggest extensions and modifications to We also provide a novel analysis and discussion on the connections promote a clear terminology and completeness. We argue that this and dependencies between the different types of biases. is more than a matter of definitions of terms. Terminology shapes how we identify and approach problems, and furthermore how we communicate with others. This is particularly important in multidis- 3 SOURCES OF BIAS ciplinary work, such as application-oriented machine learning. Our survey of sources of bias is organized in sections corresponding The taxonomy is based on a survey of published research in sev- to the major steps in the machine learning process (see Figure 1). In eral areas, and is followed by a discussion on how different types of Section 3.1 we describe bias as a major concept in the learning step. biases are connected and depend on each other. In Section 3.2 we focus on our biased world, which is the source Since humans are involved in both the creation of bias, and in of information for the learning process. Section 3.3 describes the the application of, potentially biased, systems, the presented work plethora of biases related terms used in the data generation process. 1 Umea˚ University, Sweden, email: fthomas.hellstrom,virginia.dignum, [email protected] 3.1 Bias in learning 2 This research is partly funded by the Swedish funding agency VINNOVA, project number 2019-01930. N 3 In inductive learning, the aim is to use a data set f(xi; yi)gi=1 to find BBC News, Oct. 10, 2018. Accessed July. 29, 2020. ∗ ∗ 4 Reuters Technology News, Oct. 10, 2018. Accessed July 29, 2020. a function f (x) such that f (xi) approximates yi in a good way. Copyright © 2020 for this paper by its authors. Use permitted under Creative Each xi is a vector of features, while y is denoted the output, target Commons License Attribution 4.0 International (CC BY 4.0). variable or label. An often-discussed case is when machine learning is used to build decision support systems that outputs recommenda- The learning step involves more possible sources of bias. One ex- tions, for example on whether to accept or deny loan applications in a ample is denoted uncertainty bias [19], and has to do with the prob- bank. The features may in this case be income, property magnitude, ability values that are often computed together with each produced family status, credit history, gender, and criminal record for a loan classification in a machine learning algorithm5. The probability rep- applicant, while the output y is a recommendation, 0 or 1. resents uncertainty, and typically has to be above a set threshold for a Without further restrictions, infinitely many functions perfectly classification to be considered. For example, a decision support sys- match any given data set, but most of them are typically useless since tem for bank loan applications may reject an application although it is they simply memorize the given data set but generalize poorly for classified as ‘approve’, because the probability is below the thresh- other data from the same application. Therefore, the search for a good old. This threshold is usually manually set, and may create a bias f ∗ has to be limited to a certain space Ω of functions. For example, against underrepresented demographic groups, since less data nor- the function may be assumed to be linear, which is the assumption in mally leads to higher uncertainty. linear regression. While this may be sufficient in some cases, more complex function spaces, such as high-order polynomials, or artifi- cial neural networks are often chosen. Each specific function in Ω is 3.2 A Biased World typically defined by a set of numerical weights. This preference of certain functions over others was denoted bias The word ‘bias’ has an established normative meaning in legal lan- by Tom Mitchell in his paper from 1980 with the title The Need for guage, where it refers to ‘judgement based on preconceived notions Biases in Learning Generalizations [34], and is a central concept in or prejudices, as opposed to the impartial evaluation of facts’ [8]. statistical learning theory. The expression inductive bias (also known The world around us is often described as biased in this sense, and as learning bias) is used to distinguish it from other types of biases. since most machine learning techniques simply mimic large amounts In general, inductive learning can be expressed as the minimiza- of observations of the world, it should come as no surprise that the tion problem resulting systems also express the same bias. ∗ historical bias f = arg min L(f); (1) This bias of the world is sometimes denoted . His- f2Ω torical bias has to do with data that in itself has unwanted properties that are regarded as biased: ‘Historical bias arises even if the data is where L(f) is a costfunction quantifying how well f matches the perfectly measured and sampled, if the world as it is or was leads data. The most common loss function is defined as a model to produce outcomes that are not wanted’ [45]. The bias of N the world obviously has many dimensions, each one describing some X 2 L(f) = (f(xi) − yi) : (2) unwanted aspect of the world. i=1 Sometimes, the bias in the world is analyzed by looking at cor- relations between features, and between features and the label. The The process of solving the minimization problem in Eq. 1 is called N authors of [52] show that in a certain data set, the label cooking training, and uses a training set f(xi; yi)gi=1 to search through Ω for co-occurs unproportionally often with woman, as compared to man. the function f that minimizes the loss function in Eq. 2. Since most machine learning techniques depend on correlations, such All machine learning techniques for inductive learning (for exam- biases may propagate to learned models or classifiers. The authors ple neural networks, support vector machines, and K-nearest neigh- of [52] show examples of this, and present techniques to detect and bor), need some kind of inductive bias to work, and the choice of quantify bias related to correlations.
Details
-
File Typepdf
-
Upload Time-
-
Content LanguagesEnglish
-
Upload UserAnonymous/Not logged-in
-
File Pages8 Page
-
File Size-