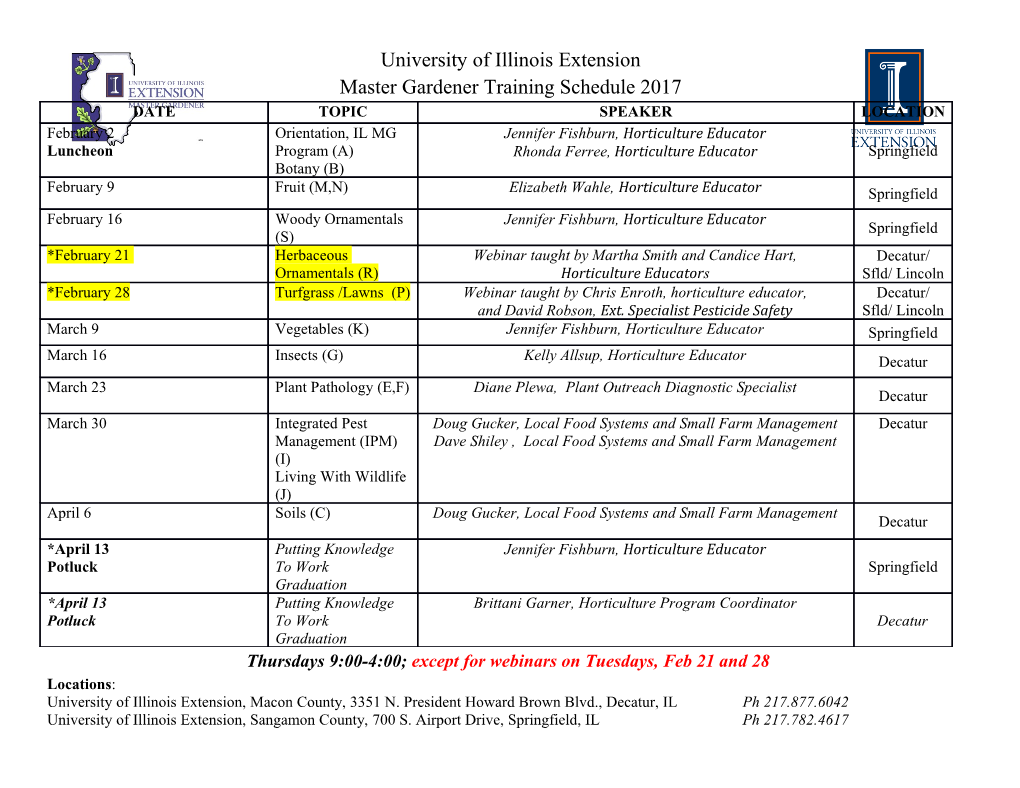
DISCOVERING AND VISUALIZING PROTOTYPICAL ARTISTS BY WEB-BASED CO-OCCURRENCE ANALYSIS 1 2 1 1 2 Markus Schedl ; Peter Knees Gerhard Widmer ; [email protected] [email protected] [email protected] 1 Department of Computational Perception Johannes Kepler University (JKU) A-4040 Linz, Austria 2 Austrian Research Institute for Artificial Intelligence (ÖFAI) A-1010 Vienna, Austria ABSTRACT Furthermore, prototypical artists are very useful for vi- sualizing music repositories since they are usually well- Detecting artists that can be considered as prototypes for known. Thus, also unexperienced music listeners are able particular genres or styles of music is an interesting task. to assign them to a particular genre or style of music and In this paper, we present an approach that ranks artists ac- can use them as reference points to discover similar but cording to their prototypicality. To calculate such a rank- less known artists. One possible way of visualizing proto- ing, we use asymmetric similarity matrices obtained via typical artists and the relations to their most similar neigh- co-occurrence analysis of artist names on web pages. We bors will be shown in this paper. demonstrate our approach on a data set containing 224 The approach presented here can be used to define artists from 14 genres and evaluate the results using the complete rankings based on the prototypicality of artists. rank correlation between the prototypicality ranking and Such rankings enable further applications. For example, a ranking obtained by page counts of search queries to together with genre information, they can serve as a mea- Google that contain artist and genre. High positive rank sure of the degree of artist membership in a particular correlations are achieved for nearly all genres of the data genre, thus defining to which extent an artist produces mu- set. Furthermore, we elaborate a visualization method that sic of a certain style or genre. illustrates similarities between artists using the prototypes Prototypicality is strongly related to the topic of sim- of all genres as reference points. On the whole, we show ilarity measurement. In fact, we exploit information on how to create a prototypicality ranking and use it, together co-occurrences of artist names on web pages to estimate with a similarity matrix, to visualize a music repository. conditional probabilities for an artist to be found on web pages of other artists. These probabilities give an asym- Keywords: prototypical artist detection, visualization, metric similarity matrix which is used for the calculation asymmetric artist similarity, web mining, co-occurrence of a prototypicality ranking. analysis Using the World Wide Web for information retrieval and data mining offers the advantage of incorporating 1 INTRODUCTION the knowledge and opinions of a large number of dif- ferent people. Thus, the Internet reflects a kind of cul- Finding artists that define a music genre or style, or at least tural knowledge that we extract and use for estimating are very typical for it, is a challenging and interesting task. artist similarity and, subsequently, for prototype detec- Information on prototypical artists may be used in various tion. However, web-based information retrieval and data areas of application. For example, music information sys- mining techniques also face some problems: First, they 1 tems like the “All Music Guide” or the “Desdichado Mu- obviously depend on the existence of web pages dealing 2 sic Information System” as well as online music stores, with the requested topic. If such web pages cannot be 3 e.g. “Amazon” , could benefit considerably. For instance, found, e.g. because the query for the search engine can- information on prototypes could be exploited to support not be defined adequately or comprises ambiguous words, their users in finding music more efficiently. web-based data mining does not yield valuable results. 1http://www.allmusic.com For example, a search for music-related web pages that 2http://www.music-i-s.com offer information about artists like “Bush”, “Kiss”, or 3http://www.amazon.com “Porn” will most probably result in a large number of web pages not dealing with these artists.4 Nevertheless, we al- ready showed that web-based co-occurrence analysis can Permission to make digital or hard copies of all or part of this be used successfully for artist similarity measurement and work for personal or classroom use is granted without fee pro- artist-to-genre classification (Schedl et al., 2005). In this vided that copies are not made or distributed for profit or com- paper, we make use of the asymmetric similarity measure mercial advantage and that copies bear this notice and the full given by the probability estimation and show how to use citation on the first page. 4To overcome this problem, we restrict the search by adding c 2005 Queen Mary, University of London music-related keywords to the queries. 21 it for defining an artist prototypicality ranking. that “Metallica” serves as a prototype for the genre heavy The remainder of this paper is organized as follows. metal. Ellis et al. (2002) regard this asymmetry as a prob- Related work is briefly summarized in Section 2. In Sec- lem since it undermines a Euclidean model of similarity. tion 3, we present our approach to prototype detection and In fact, nearly all of the cited publications dealing with the performed evaluation, and we discuss the results. Sec- co-occurrence-based similarity measurement consider the tion 4 describes our “Continuous Similarity Ring (CSR)” asymmetry a shortcoming and perform operations to sym- visualization that is used to illustrate relations between metrize the similarity matrices. To contrast, in this paper, prototypical artists and their most similar neighbors. Fi- we describe a prototype detection approach that capital- nally, in Section 5, we summarize the work, draw conclu- izes on asymmetric similarity matrices. sions, and point out possible future research directions. 3 PROTOTYPE DETECTION 2 RELATED WORK In the following, we sketch how we use co-occurrence While we could not find previous work on prototype de- analysis to define an asymmetric similarity measure. To tection for music artists, there has been some work on co- this end, we apply the same technique as in (Schedl et al., occurrence analysis in music information retrieval. One of 2005). Based on this similarity measure, we then elabo- the first publication on MIR-related co-occurrence analy- rate our novel method for calculating the prototypicality sis is (Pachet et al., 2001), where playlists of radio sta- ranking. tions and databases of compilation CDs are used to de- tect co-occurrences between titles and between artists. In (Ellis et al., 2002; Whitman and Lawrence, 2002), first 3.1 Methodology attempts to exploit the cultural knowledge offered by the 3.1.1 Co-Occurrence Analysis World Wide Web can be found. User collections of the music sharing service “OpenNap” are analyzed to gain a Given a list of artist names, we use Google to estimate the similarity measure based on community metadata. The number of web pages containing each artist and each pair artist co-occurrences extracted from these collections are of artists. Since we are not interested in the content of the evaluated by comparison with direct subjective similarity found web pages, but only in their number, the search is judgments obtained via a web-based survey. In contrast restricted to display only the top-ranked page. In fact, the to this survey of non-professionals, Cano and Koppen- only information we use is the page count that is returned berger (2004) use expert opinions taken from the “All Mu- by Google. This raises performance and limits web traffic. sic Guide” to create a similarity network. To this end, the Addressing the issue of finding only music-related “similar artists” links of 400 artists are gathered. Further- web pages, we add additional keywords to the more, co-occurrences on playlists from “The Art of the Google search query. More precisely, we use the Mix”5 are extracted and visualized as a network contain- scheme “artist1” [“artist2”]+music+review to form ing more than 48.000 artists. queries. This scheme, already used in (Whitman and Zadel and Fujinaga (2004) also investigate co- Lawrence, 2002), proved to yield good results for occurrences of artist names on web pages. In contrast classification tasks (Knees et al., 2004; Schedl et al., to our work, Zadel and Fujinaga (2004) focus on the us- 2005). Furthermore, it performed slightly better than age of web services for creating clusters of similar music “artist1” [“artist2”]+music+genre+style in first experi- artists. Starting with a seed artist, the Amazon web ser- ments of prototype detection. vice “Listmania!” is used to obtain a list of potentially The outcome of the querying procedure is a symmet- related artists. Based on this list, co-occurrences are de- ric matrix C, where element cij gives the number of web rived by querying Google. Thereafter, the “relatedness” of pages containing the artist with index i together with the j each “Listmania!”-artist to the seed artist is calculated as one indexed by . The values of the diagonal elements the ratio between the combined page count and the mini- cii show the total number of web pages containing artist mum of the single page counts for both artists. In contrast i. Based on the page count matrix C, we then use rela- to our co-occurrence approach, the one used in (Zadel and tive frequencies to calculate a conditional probability ma- Fujinaga, 2004) does not yield complete similarity matri- trix P as follows. Given two events ai (artist with index ces. i is mentioned on web page) and aj (artist with index j In this paper, we use the same technique as described is mentioned on web page), we estimate the conditional in (Schedl et al., 2005) to obtain a similarity matrix based probability pij (the probability for artist j to be found on on co-occurrences.
Details
-
File Typepdf
-
Upload Time-
-
Content LanguagesEnglish
-
Upload UserAnonymous/Not logged-in
-
File Pages8 Page
-
File Size-