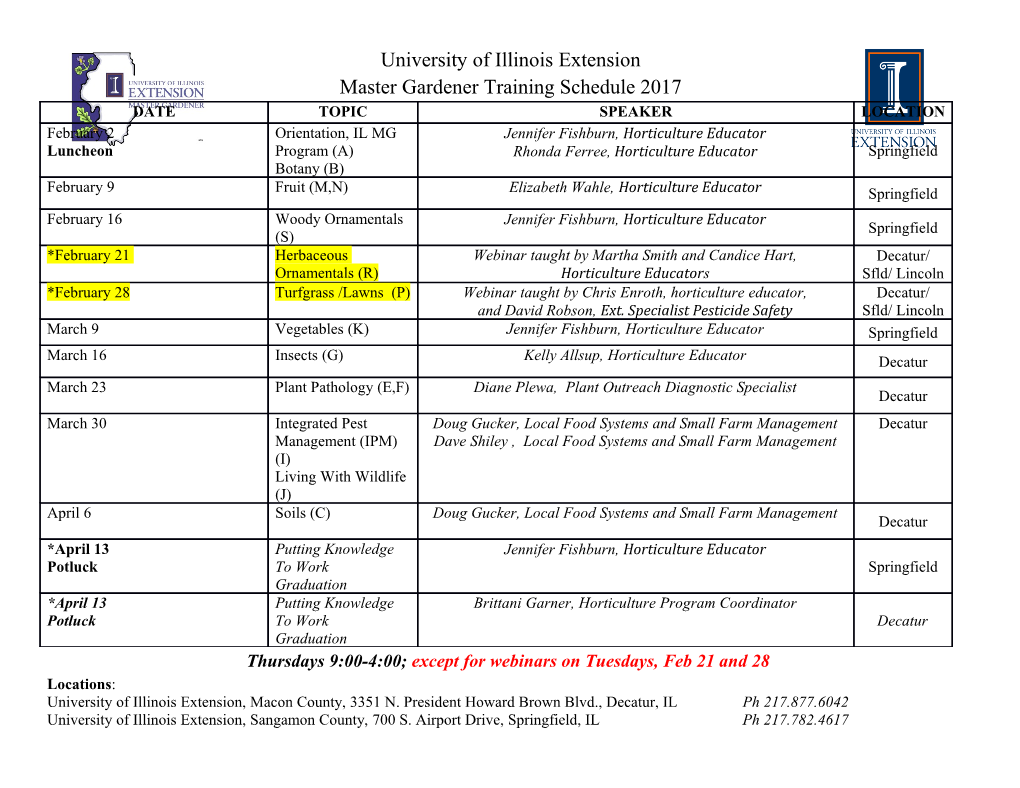
IOP Conference Series: Earth and Environmental Science PAPER • OPEN ACCESS Related content - Between the mean and the median: the Lp Robustness of location estimators under t- estimator Francesca Pennecchi and Luca Callegaro distributions: a literature review - Generalized shrunken type-GM estimator and its application C Z Ma and Y L Du To cite this article: C Sumarni et al 2017 IOP Conf. Ser.: Earth Environ. Sci. 58 012015 - On the Lp estimation of a quantity from a set of observations R Willink View the article online for updates and enhancements. This content was downloaded from IP address 170.106.33.19 on 25/09/2021 at 16:42 ISS IOP Publishing IOP Conf. Series: Earth and Environmental Science 58 (2017) 012015 doi:10.1088/1755-1315/58/1/012015 International Conference on Recent Trends in Physics 2016 (ICRTP2016) IOP Publishing Journal of Physics: Conference Series 755 (2016) 011001 doi:10.1088/1742-6596/755/1/011001 Robustness of location estimators under t-distributions: a literature review C Sumarni1,2, K Sadik1, K A Notodiputro1 and B Sartono1 1 Department of Statistics, Bogor Agriculture University, INDONESIA. 2 Statistics Indonesia, Bali Province. Email: [email protected], [email protected], [email protected], [email protected] Abstract. The assumption of normality is commonly used in estimation of parameters in statistical modelling, but this assumption is very sensitive to outliers. The t-distribution is more robust than the normal distribution since the t-distributions have longer tails. The robustness measures of location estimators under t-distributions are reviewed and discussed in this paper. For the purpose of illustration we use the onion yield data which includes outliers as a case study and showed that the t model produces better fit than the normal model. 1. Introduction Estimation of parameters in a survey often assumes that the data is a random sample from a normally distributed population. Suppose that sample data are recorded n units ( ), and are assumed as random samples from normal distribution, 2 (1) yNi iid ()μ,σ In statistical modeling it is also common to assume normality of the error terms. The model can be written as (2) and called the normal model. T 2 or yNii= x β+,εεσ i where i ()0, (2) 2 yNi iid ()μ(β ),σ Assuming normal distribution implies that the estimates become inaccurate when there are outliers in the data. We may assume robust distribution of the error terms to solve this problem. The t- distribution is useful for statistical modelling when data contains outliers. Lange et al. [1] has successfully demonstrated that the estimation results were robust to outliers in linear models if the 2 assumptions of normality has been replaced by assuming a t-distribution, εσi tv(0, , ) . The t-model can be written as 2 yti iid ()μ(β ),σ , v (3) Content from this work may be used under the terms of the Creative Commons Attribution 3.0 licence. Any further distribution of this work must maintain attribution to the author(s) and the title of the work, journal citation and DOI. Published under licence by IOP Publishing Ltd 1 ISS IOP Publishing IOP Conf. Series: Earth and Environmental Science 58 (2017) 012015 doi:10.1088/1755-1315/58/1/012015 where tv((),,)μ β σ 2 denotes the univariate t-distribution with location parameter μ()β where β is a vector of coefficient’s regression, scale parameter σ 2 and v degrees of freedom. Lange et al. [1] has not shown explicit measures of the robustness of location estimators under t- distributions. In this paper, this robustness is reviewed by referring to the idea that the robustness of an estimator can be measured from the influence functions (IF), the asymptotic relative efficiency (ARE) and the breakdown point [2]. In this case, we focus on the influence functions and the asymptotic relative efficiency. We also study the influence of outliers toward modelling by illustrating the model of solid pesticides effects on the onion yield. 2. Location estimation under t-distributions We assume in a robust estimation that are random samples from t-distributed population which density function (pdf) is defined by (4). −(1)/2v+ ⎛⎞2 Γ((v + 1) / 2) 1 ⎛⎞yi − μ fy()i = ⎜1+ ⎟−∞≤≤∞, y (4) 2 ⎜⎟v ⎜⎟ πσvvΓ(/2)⎝⎠⎝⎠σ Note that, If and , (4) is a density of univariate Student’t distributions with degrees of freedom (), otherwise to be noncentral t-distributions with location parameter , scale parameter and shape parameter degrees of freedom [3]. Denote logarithm functions of (4) as , ⎛⎞2 112 (1)1v + ⎛⎞yi − μ lvi = ln()Γ ((+ 1)/2)−−−Γ−⎜ ln()πσ v ln ln() ( v /2) ln1+ ⎟ (5) 22() 2⎜⎟v ⎜⎟ ⎝⎠⎝⎠σ or it can be written as 2 (1)v + ⎛⎞ 1⎛⎞y − μ l = constant−⎜ ln 1+ i ⎟ (6) i 2 ⎜⎟v ⎜⎟ ⎝⎠⎝⎠σ The first derivative of respect to is ∂−lyiiv +1 ⎛⎞μ = (7) ∂μ 22⎜⎟ vy+ ()()/i − μ σ ⎝⎠σ If σ 2 is assumed known and v is fixed, we would get the maximum likelihood estimator (MLE) of by finding the solution of this equation, n ∂l ∑ i = 0 i=1 ∂μ n v +1 ⎛⎞y − μ i = 0 ∑ 22⎜⎟ i=1 vy+ ()()/i − μ σ ⎝⎠σ v +1 n ⎛⎞y − μ If we denote w , then w i 0 i = 2 i ⎜⎟2 = ∑ σ vy+ ()()/i − μ σ i=1 ⎝⎠ The MLE of μ under t-distributions is ⎛⎞nn ˆ wy w μTiii= ⎜⎟∑∑ (8) ⎝⎠ii==11 2 ISS IOP Publishing IOP Conf. Series: Earth and Environmental Science 58 (2017) 012015 doi:10.1088/1755-1315/58/1/012015 This is a weighted mean with depending on the sample, so the location estimator under t-distributions is one of an M-estimator [2]. Since is a function of parameters (), equation (8) has no closed form solution. The EM algorithm can be used to get the solution (for more detailed see Lange et al. [1]). 3. The measure of robustness under t-distributions Robustness of an estimator can be measured quantitatively and qualitatively. The quantitative robustness can be measured by a breakdown point and the qualitative ones can be seen from efficiency and stability. 3.1. The Influence Function (IF) The stability of robust estimators can be seen from the influence function. The influence function can be computed according to the function. The influence function of an M-estimate (maximum likelihood type estimates) is defined as [2]. ψ ()y;μ IF(.) = (9) −∂∂Ey⎡⎤⎣⎦()()/;μ ψ μ If the function of an M-estimator is computed from the first derivative of logarithm function of pdf, ∂ ψ ()yfy;ln()μ = ()− , then the M-estimator is the usual MLE [4]. ∂μ The location estimator under the t-distributions is a form of M-estimators with the ψ function defined as (10). vy+1 − μ y; ⎛⎞ ψ ()μ = 22⎜⎟ (10) vy+ ()()/− μ σ ⎝⎠σ The computing of the first derivative of the ψ function (10) can be seen in Appendix and the expectation is 2 ⎡⎤()v +1 ⎡⎤⎛⎞y − μ ⎢⎥⎢⎥− v ⎡⎤2 ⎜⎟ ∂ ⎡⎤vy+1 ⎛⎞− μ ⎢⎥σ ⎢⎥⎝⎠σ EyE/;⎢⎥⎢⎥E⎣⎦ ⎣⎦⎡⎤()()∂∂μ ψ μ ==22⎜⎟ ⎢⎥2 ⎢⎥∂μ ⎢⎥vy()/⎝⎠σ 2 ⎣⎦⎣⎦+ ()− μ σ ⎢⎥vy+ ()()/− μ σ ⎢⎥() ⎣⎦ ⎡⎤⎡⎤2 1 ⎛⎞y − μ ⎢⎥⎢⎥⎛⎞v ⎢⎥⎜⎟⎜⎟− ()v +1 ⎢⎥v ⎜⎟⎝⎠σ = E ⎢⎥⎢⎥⎝⎠ ⎢⎥v 22⎢⎥2 σ ⎛⎞vy+ ()/− μ σ ⎢⎥⎢⎥⎜⎟() ⎢⎥⎢⎥⎜⎟v ⎣⎦⎣⎦⎝⎠ y − μ Let z = , Lange et al. (1989) has computed that the integration yields, σ ⎛⎞⎛⎞vv 2 −m ⎜⎟⎜⎟+ m −1 ⎡⎤⎛⎞z ⎝⎠⎝⎠22 E ⎢⎥⎜⎟1+= and ⎢⎥⎝⎠v ⎛⎞⎛⎞vv++11 ⎣⎦⎜⎟⎜⎟+ m −1 ⎝⎠⎝⎠22 3 ISS IOP Publishing IOP Conf. Series: Earth and Environmental Science 58 (2017) 012015 doi:10.1088/1755-1315/58/1/012015 ⎡⎤⎛⎞z2 11 22−−21⎢⎥⎜⎟+ − 2 2−2 ⎡⎤zz⎛⎞ v ⎡⎛⎞⎛⎞z z ⎤ ⎢⎥⎝⎠ . So we get EEE⎢⎥⎜⎟11+=2 =+ ⎢⎜⎟⎜⎟− 1+ ⎥ vv ⎢⎥2 v v ⎣⎦⎢⎥⎝⎠ ⎛⎞z ⎣⎢⎝⎠⎝⎠ ⎦⎥ ⎢⎥⎜⎟1+ ⎣⎦⎢⎥⎝⎠v ⎡⎤⎡⎤2 ⎢⎥⎢⎥z 22 −1 ⎡⎤⎡⎤22−−2 ⎢⎥()vv++11⎢⎥v ()⎛⎞⎛⎞zz () v+ 1⎛⎞z EyE/; E⎢⎥1 E⎢⎥1 ⎡⎤⎣⎦()()∂∂μ ψ μ ==+⎢⎥22⎢⎥ 2⎜⎟⎜⎟− 2 ⎜⎟+ vvσσ2 ⎢⎥vv ⎢⎥ v σv ⎢⎥⎢⎥⎛⎞z ⎣⎦⎝⎠⎝⎠⎣⎦ ⎝⎠ ⎜⎟1+ ⎢⎥⎢⎥v ⎣⎦⎣⎦⎝⎠ ()vv++11⎡⎤⎡⎤v ()v()v+2 = 22⎢⎥⎢⎥− vvσσ⎣⎦⎣⎦⎢⎥⎢⎥()()vv++13()() vv ++ 13 1 ()v + 2 = − σσ22vv++33 () () ()v +1 = − σ 2 ()v + 3 and ()v +1 −∂∂Ey⎡⎤()()/;μ ψ μ = 2 (11) ⎣⎦σ ()v + 3 Thus the influence function of an M-estimator under t-distributions is v + 3 IF ˆ y ()μμT = 2 ()− (12) vy+ ()()− μ / σ How to see that μˆT (location estimator under t-distribution) is more robust than μˆ N , the location estimator under normal distribution ( )? To answer this, we must know the ψ function and the influence function under normal distribution. The same way as before, we get under normal distribution the ψ function is y − μ ψ *;()y μ = (13) σ 2 and the influence function IF() is IF()μˆN = y − μ (14) The influence function of location estimators under normal distribution is a trend line and unbounded. The increasingly an observed value ( ) will produce a higher value of the influence function ( ) and vice versa. It means that the data’s outlier will influence highly on the estimates. Meanwhile, the influence function of location estimators under t-distributions is a bounded function (see figure 1). That is since any outlier data is down weighted by v +1 wi = 2 (15) vy+ ()()/i − μ σ 4 ISS IOP Publishing IOP Conf. Series: Earth and Environmental Science 58 (2017) 012015 doi:10.1088/1755-1315/58/1/012015 where is a shape parameter, then influence of such data is reduced. An outlier will not affect the estimate significantly. That is why the location estimator under t-distributions is more robust than under the normal distribution. t-IF normal-IF -3 -2 -1 0 1 2 3 -3 -2 -1 0 1 2 3 -20 -10 0 10 20 -20 -10 0 10 20 y y Figure 1.
Details
-
File Typepdf
-
Upload Time-
-
Content LanguagesEnglish
-
Upload UserAnonymous/Not logged-in
-
File Pages12 Page
-
File Size-