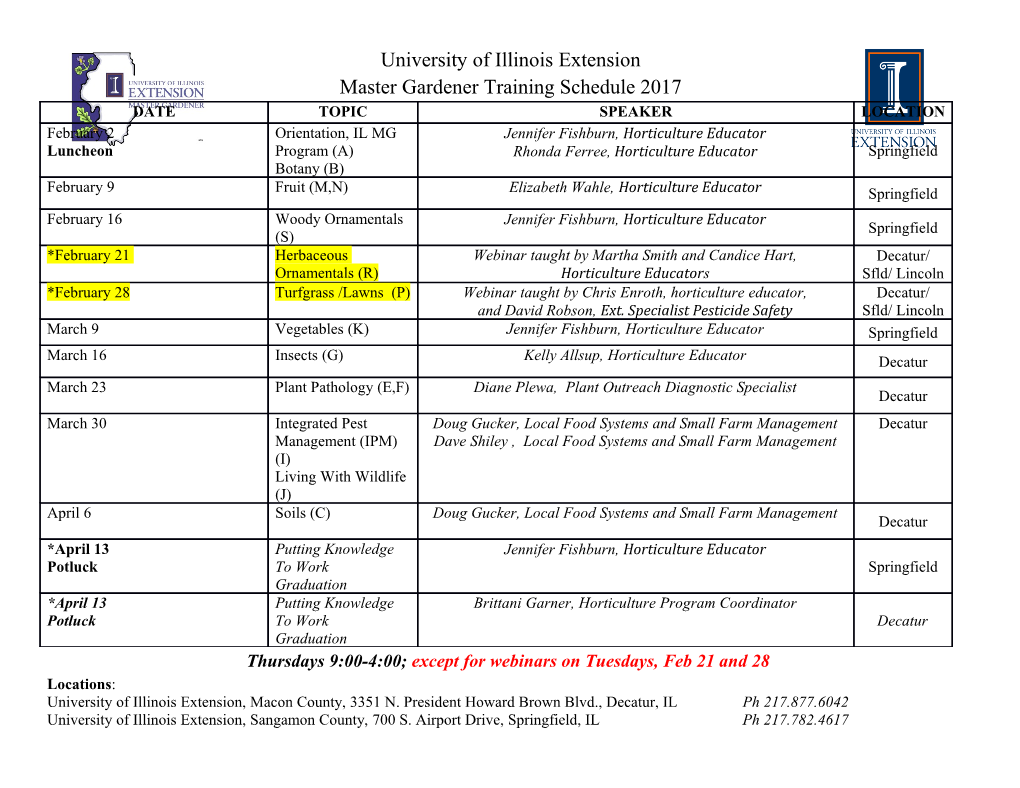
How to build a MC generator Tomasz Golan University of Wroclaw NuSTEC, Fermilab 2017 Monte Carlo method Buffon’s needle problem Suppose we have a floor made of parallel strips of wood, each the same width, and we drop a needle onto the floor. What is the probability that the needle will lie across a line between two strips? Georges-Louis Leclerc, blue are good Comte de Buffon 18th century red are bad Monte Carlo without computers If needle length (l) < lines width (t): MC experiment was performed by Mario Lazzarini in 1901 by throwing 3408 2l P = needles: tπ which can be used to estimate π: 2l 3408 355 π = · = = 3.14159292 2l t #red 113 π = · tP Tomasz Golan MC generators @ NuSTEC 3 / 40 From Solitaire to Monte Carlo method ■ Stanis law Ulam was a Polish mathematician ■ He invented the Monte Carlo method while playing solitaire ■ The method was used in Los Alamos, performed by ENIAC computer ■ What is a probability of success in solitaire? ◆ Too complex for an analytical calculations ◆ Lets try N = 100 times and count wins ◆ With N we are getting closer to→ correct ∞ result Tomasz Golan MC generators @ NuSTEC 4 / 40 Newton-Pepys problem Monte Carlo method Which of the following three Buffon’s needle problem From Solitaire to MC propositions has the greatest Newton-Pepys problem PRNG chance of success? Hit-or-miss method MC integration results Optimization of MC Crude method A Six fair dice are tossed Methods comparison Random from PDF independently and at least CDF CDF discrete one “6” appears. CDF continuous Acceptance-rejection B Twelve fair dice are tossed Quasi-elastic scattering independently and at least two “6”s appear. C Eighteen fair dice are tossed independently and at least three “6”s appear. Tomasz Golan MC generators @ NuSTEC 5 / 40 Newton-Pepys problem: analytical attempt ■ First, lets go back to high school and calculate this analytically Monte Carlo method Buffon’s needle problem From Solitaire to MC ■ 1 Newton-Pepys problem Let p = 6 be the probability of rolling 6 PRNG Hit-or-miss method MC integration results ■ The probability of not rolling 6 is (1 p) Optimization of MC Crude method − Methods comparison Random from PDF A six attempts, at least one six CDF CDF discrete CDF continuous 6 Acceptance-rejection PA = 1 (1 p) 0.6651 Quasi-elastic scattering − − ≈ B twelve attempts, at least two sixes 12 12 11 PB = 1 (1 p) p(1 p) 0.6187 − − − 1 − ≈ C eighteen attempts, at least three sixes 18 18 17 18 2 16 PC = 1 (1 p) p(1 p) p (1 p) 0.5973 − − − 1 − − 2 − ≈ Tomasz Golan MC generators @ NuSTEC 6 / 40 Newton-Pepys problem: MC attempt def throw (nSixes): ■ MC attempt is just “performing the n = 0 experiment”, so we will be rolling for _ in range (6 * nSixes): dices if random.randint (1, 6) == 6: n += 1 return n >= nSixes ■ Roll 6n times and check if number of def MC (nSixes, nAttempts): sixes is greater or equal n n = 0 for _ in range (nAttempts): ■ Repeat N times and your probability n += throw (nSixes) is given by: return float (n) / nAttempts number of successes P = if __name__ == "__main__": N for i in range (1, 4): print MC (i, 1000) Tomasz Golan MC generators @ NuSTEC 7 / 40 Newton-Pepys problem: summary ■ Your MC result depends on N Monte Carlo method Buffon’s needle problem From Solitaire to MC ■ Newton-Pepys problem Results for N = 100: PRNG Hit-or-miss method MC integration results Optimization of MC true Crude method PA = 0.71, 0.68, 0.76, 0.65, 0.68 PA = 0.6651 Methods comparison true Random from PDF PB = 0.70, 0.56, 0.60, 0.63, 0.69 P = 0.6187 CDF B CDF discrete true CDF continuous PC = 0.62, 0.62, 0.53, 0.57, 0.62 PC = 0.5973 Acceptance-rejection Quasi-elastic scattering ■ Results for N = 106: PA = 0.6655, 0.6648, 0.6653, 0.6662, 0.6653 PB = 0.6188, 0.6191, 0.6191, 0.6190, 0.6182 PC = 0.5975, 0.5979, 0.5972, 0.5978, 0.5973 ■ Your MC results also depends on the way how random numbers were generated Tomasz Golan MC generators @ NuSTEC 8 / 40 Pseudorandom number generator ■ PRNG is an algorithm for generating a sequence of “random” Monte Carlo method Buffon’s needle problem numbers From Solitaire to MC Newton-Pepys problem PRNG ■ Hit-or-miss method Example: middle-square method (used in ENIAC) MC integration results Optimization of MC ◆ Crude method take n-digit number as your seed Methods comparison Random from PDF CDF ◆ square it to get 2n-digit number (add leading zeroes if CDF discrete CDF continuous necessary) Acceptance-rejection Quasi-elastic scattering ◆ n middle digits are the result and the seed for next number ■ Middle-square method for n = 4 and base seed = 1111: 11112 = 01234321 2343 → 23432 = 05489649 4896 . → . 11112 = 01234321 2343 → Tomasz Golan MC generators @ NuSTEC 9 / 40 Pseudorandom number generator ■ Nowadays, more sophisticated PRNGs exist, but they also Monte Carlo method Buffon’s needle problem suffer on some common problems: From Solitaire to MC Newton-Pepys problem ◆ PRNG periodicity / different periodicity for different base seed Hit-or-miss method MC integration results Optimization of MC ◆ Crude method nonuniformity of number distributions Methods comparison Random from PDF CDF ◆ correlation of successive numbers CDF discrete CDF continuous Acceptance-rejection Quasi-elastic scattering Mathematics and Computers in Simulations 46 (1998) 485-505 Tomasz Golan MC generators @ NuSTEC 10 / 40 MC integration (hit-or-miss method) Lets do the following integration using MC method: Monte Carlo method Buffon’s needle problem From Solitaire to MC 1 1 2 1 Newton-Pepys problem 1 1 x 1 PRNG f(x)dx = x dx = = Hit-or-miss method Z0 Z0 2 2 2 0 4 MC integration results Optimization of MC Crude method y Methods comparison Random from PDF ■ take a random point from CDF CDF discrete the [0, 1] [0, 1] square CDF continuous × 1 Acceptance-rejection ■ 1 Quasi-elastic scattering compare it to your f(x) f(x) = 2 x ■ repeat N times ■ count n points below the function ■ you results is given by 1 x 1 n n f(x)dx = P = Z0 · N N Tomasz Golan MC generators @ NuSTEC 11 / 40 N = 100 (hit-or-miss) N = 10000 (hit-or-miss) 0.5 0.5 0.4 0.4 0.3 0.3 0.2 0.2 MC result MC result 0.1 0.1 0 0 0 200 400 600 800 1000 0 200 400 600 800 1000 Run number Run number N = 1000 (hit-or-miss) N = 100000 (hit-or-miss) 0.5 0.5 0.4 0.4 0.3 0.3 0.2 0.2 MC result MC result 0.1 0.1 0 0 0 200 400 600 800 1000 0 200 400 600 800 1000 Run number Run number Optimization of MC y y Monte Carlo method Buffon’s needle problem From Solitaire to MC Newton-Pepys problem 1 PRNG f(x) = 1 x f(x) = 1 x Hit-or-miss method 2 2 MC integration results Optimization of MC Crude method 0.5 Methods comparison Random from PDF CDF CDF discrete CDF continuous Acceptance-rejection 1 x x Quasi-elastic scattering 1 ■ You want to avoid generating “red” points as they do not contribute to your integral ■ You can choose any rectangle as far as it contains maximum of f(x) in given range Tomasz Golan MC generators @ NuSTEC 13 / 40 Optimization of MC ■ Lets consider the following Monte Carlo method function: Buffon’s needle problem 0.2 From Solitaire to MC ) Newton-Pepys problem 2 PRNG Q Hit-or-miss method 1 ( MC integration results 2 F (Q ) = F 0.1 Optimization of MC 2 2 Crude method (1 + Q ) Methods comparison Random from PDF CDF more or less dipole form 0 CDF discrete CDF continuous factor 2 4 6 8 10 Acceptance-rejection Q2 Quasi-elastic scattering ■ Integrating this function over Q2 is highly inefficient 1 ■ However, one can integrate ) x by substitution to get ( F 0.5 better performance, e.g. 2 x = log10(Q ) 0 2 1 0 1 − − 2 don’t forget about Jacobian x = log10(Q ) Tomasz Golan MC generators @ NuSTEC 14 / 40 MC integration (crude method) Lets do the following integration using MC method once again: Monte Carlo method Buffon’s needle problem From Solitaire to MC 1 1 2 1 Newton-Pepys problem 1 1 x 1 PRNG f(x)dx = x dx = = Hit-or-miss method Z0 Z0 2 2 2 0 4 MC integration results Optimization of MC Crude method ■ One can approximate y Methods comparison Random from PDF integral CDF CDF discrete b N CDF continuous b a Acceptance-rejection f(x)dx − f(xi) 1 Quasi-elastic scattering Z ≈ N f(x) = x a Xi=1 2 where xi is a random number from [a, b] ■ It can be shown that crude method is more accurate x than hit-or-miss ■ We will skip the math and look at some comparisons Tomasz Golan MC generators @ NuSTEC 15 / 40 N = 100 (hit-or-miss) N = 100 (crude) 0.5 0.5 0.4 0.4 0.3 0.3 0.2 0.2 MC result MC result 0.1 0.1 0 0 0 200 400 600 800 1000 0 200 400 600 800 1000 Run number Run number N = 1000 (hit-or-miss) N = 1000 (crude) 0.5 0.5 0.4 0.4 0.3 0.3 0.2 0.2 MC result MC result 0.1 0.1 0 0 0 200 400 600 800 1000 0 200 400 600 800 1000 Run number Run number Random numbers from probability density function Monte Carlo method Buffon’s needle problem From Solitaire to MC Newton-Pepys problem PRNG Hit-or-miss method ■ MC integration results How to generate a random 3 Optimization of MC Crude method number from probability Methods comparison Random from PDF density function? 2 CDF CDF discrete y CDF continuous ■ Lets consider f(x) = 3x2 Acceptance-rejection 1 Quasi-elastic scattering ■ Which means that x = 1 should be thrown 2 times 0 √ 0 0.5 1 more often than x = 2 2 x Tomasz Golan MC generators @ NuSTEC 17 / 40 Cumulative distribution function ■ Cumulative distribution function of a random variable X: Monte Carlo method Buffon’s needle problem From Solitaire to MC Newton-Pepys problem F (x) = P (X x) PRNG ≤ Hit-or-miss method MC integration results Optimization of MC Crude method Note: 0 F (x) 1 for all x Methods comparison ≤ ≤ Random from PDF CDF ■ CDF discrete Discrete random variable X: CDF
Details
-
File Typepdf
-
Upload Time-
-
Content LanguagesEnglish
-
Upload UserAnonymous/Not logged-in
-
File Pages40 Page
-
File Size-