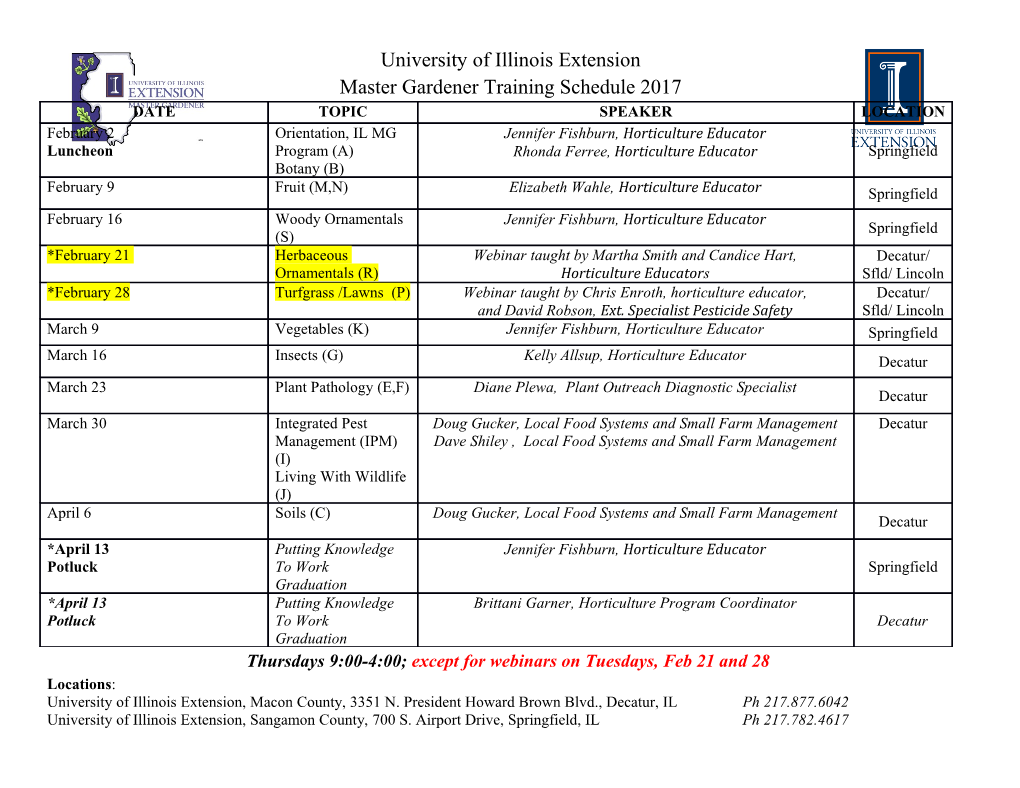
Rheumatol Int (2015) 35:973–979 Rheumatology DOI 10.1007/s00296-014-3178-9 INTERNATIONAL ORIGINAL ARTICLE - GENES AND DISEASE Predicting the potential ankylosing spondylitis-related genes utilizing bioinformatics approaches Hao Zhao · Dan Wang · Deyu Fu · Luan Xue Received: 25 August 2014 / Accepted: 11 November 2014 / Published online: 29 November 2014 © Springer-Verlag Berlin Heidelberg 2014 Abstract Given that ankylosing spondylitis (AS) occurs down-regulated genes. These DEGs were significantly in approximately 5 out of 1,000 adults of European descent enriched in phosphorylation (p 1.21E 05) and positive = − and the unclear pathogenesis, the aim of the research was regulation of gene expression (p 1.25E 03). Further- = − to further predict the molecular mechanism of this dis- more, one module was screened out from the up-regulated ease. The Affymetrix chip data GSE25101 were available network, which contained 39 nodes and 205 edges. More- from Gene Expression Omnibus database. First of all, dif- over, the nodes in the module were significantly enriched ferentially expressed genes (DEGs) were identified by in ribosomal protein (RPL17, ribosomal protein L17 and Limma package in R. Moreover, DAVID was used to per- MRPL22, mitochondrial ribosomal protein L22) and pro- form gene set enrichment analysis of DEGs. In addition, teasome (PSMA6, proteasome subunit, alpha type 6, miRanda, miRDB, miRWalk, RNA22 and TargetScan were PSMA4)-related domains. Our findings that might explore applied to predict microRNA-target associations. Mean- the potential pathogenesis of AS and RPL17, MRPL22, while, STRING 9.0 was utilized to collect protein–protein PSMA6 and PSMA4 have the potential to be the biomark- interactions (PPIs) with confidence score >0.4. Then, the ers for the disease. PPI networks for up- and down-regulated genes were con- structed, and the clustering analysis was undergone using Keywords Ankylosing spondylitis · Differentially ClusterONE. Finally, protein-domain enrichment analy- expressed gene · MicroRNA · Protein–protein interaction sis of modules was conducted using DAVID. Total 145 network · Functional analysis DEGs were identified, including 103 up-regulated and 42 Introduction H. Zhao (*) Department of Arthritis Emergency, Guanghua Integrative Medicine Hospital, Changning District, Shanghai, China Ankylosing spondylitis (AS) has been found to be a com- e-mail: zhh‑[email protected] mon inflammatory rheumatic disease predominantly of the axial skeleton, causing severe inflammatory back H. Zhao pain, inducing structural and functional impairments Institute of Arthritis Research, Shanghai Academy of Chinese Medical Sciences, 540 Xinhua Road, Shanghai 200052, China and decreasing patients’ quality of life [1]. The disease is characterized by the inflammation of the spine and sacro- D. Wang · L. Xue iliac joints, which will then causing pain and stiffness and Department of Rheumatology, Yueyang Hospital of Integrated ultimately new bone formation and leading to progressive Traditional Chinese and Western Medicine, Shanghai University of Traditional Chinese Medicine, Shanghai 200437, China joint ankylosis [2]. However, the disease status, including terms of disease activity, disease progression and prognosis D. Fu are difficult to define in AS [3]. Currently, the underlying Department of Cardiovascular Medicine, Yueyang Hospital molecular mechanism of the disease is still unclear. There- of Integrated Traditional Chinese and Western Medicine, Shanghai University of Traditional Chinese Medicine, fore, there is an urgent need to predict the pathogenesis of Shanghai 200437, China AS. 1 3 974 Rheumatol Int (2015) 35:973–979 MicroRNAs (miRNAs) are endogenous 22-nucleotide was used to conduct the multiple correction, and false dis- RNAs, some of which are discovered playing important covery rate (FDR) was obtained. Finally, FDR < 0.05 and regulatory roles in animals by targeting the messages of |log2FC| > 0.5 were set as thresholds to identify DEGs protein-coding genes for translational repression [4]. The between the two kinds of samples. alterations of miRNAs are involved in the initiation and progression of human cancer. MicroRNA-146a (miR-146a) Functional enrichment analysis and its target IL-1R-associated kinase (IRAK1) have been detected playing a role in psoriatic arthritis susceptibility In the present study, database for annotation, visualiza- [5]. Additionally, human leukocyte antigen (HLA)-B27 is tion and integrated discovery (DAVID) [14] was applied to discovered playing an important role in AS, and the evi- conduct gene ontology (GO) analysis of DEGs. GO terms dence came from linkage and association studies both in are significantly overrepresented in a set of genes from humans and in transgenic animal models [6, 7]. HLA mark- three aspects, including cellular component (CC), molecu- ers and linkage disequilibrium blocks near HLA-DPA1 lar function (MF) and biological process (BP) [15]. In our and HLA-DPB1 are statistically associated with AS [8]. work, the significant GO terms with p < 0.05 and the num- Additionally, endoplasmic reticulum aminopeptidase 1 ber of DEGs > 2 were selected for further analysis. (ERAP1), interactived with HLA-B27, is also important in the pathogenesis of AS [9]. Although several factors have Predicting miRNAs for DEGs been found related to the disease, the molecular mechanism has not been fully described. Thus, in the present study, we Total five miRNA-target prediction tools, including utilized several informatics approaches to further investi- miRanda [16], miRDB [17], miRWalk [18], RNA22 [19] gate the mechanism of AS. and TargetScan [20] were used to predict miRNAs that reg- In the current study, original chip data were downloaded ulate the identified DEGs. miRNAs, predicted by more than and then the differentially expressed genes (DEGs) were three times, were screened out for further analysis. identified between AS and normal controls. By analyzing the gene expression alterations and miRNA-target associa- Constructing protein–protein interaction network tions, we predicted the roles of genes in AS progression. Furthermore, the modules in PPI networks were ana- As a database of predicted functional associations between lyzed and the significantly enriched GO terms and protein proteins, Search Tool for the Retrieval of Interacting Genes domains were screened out. Based on these results, several (STRING) [21] was used in the current research. Func- genes were detected playing important roles in AS initia- tional links between proteins are usually inferred from tion and progression. genomic associations between the genes that encode them: groups of genes that are required for the same function tend to show similar species coverage and are often located in Materials and methods close proximity on the genome. In the present study, the protein–protein interactions (PPIs) with confidence scores Affymetrix chip data more than 0.4 were selected for PPI network construc- tion. Moreover, the PPIs network was visualized using The Gene Expression Omnibus (GEO) database at National Cytoscape [22], which is a popular bioinformatics package Center for Biotechnology Information (NCBI) is currently for biological network visualization and data integration. the largest fully public gene expression resource. This database includes 214,268 samples and 4,500 platforms Clustering analysis for protein–protein interaction network [10]. The microarray dataset GSE25101 [11] were avail- able from GEO, which included 16 AS patients with active Clustering analysis is a method for detecting potentially disease and 16 gender- and age-matched healthy controls. overlapping protein complexes from PPI data. After The platform was GPL6947 Illumina HumanHT-12 V3.0 the PPI network was constructed, ClusterONE [23] in expression beadchip, containing 49576 probes. Cytoscape was utilized to perform clustering analysis for PPI network with minimum size 5 and minimum = Identifying DEGs density 0.05. Finally, modules with p < 1.0E 5 were = − selected for analysis. Then, DAVID was applied to conduct Given that the chip data were normalized, we utilized protein-domain analysis to the modules based on InterPro Limma package [12] in R (V.3.0.1) to screen out DEGs database [24], and the remarkable domains with p < 0.05 between AS and normal controls. Bayesian methods [13] were selected. 1 3 Rheumatol Int (2015) 35:973–979 975 Table 1 Top 10 terms for up- and down-regulated genes with p < 0.05, respectively DEGs Term ID Description Count p value Up genes GO:0006119 Oxidative phosphorylation 7 1.21E 05 − GO:0006414 Translational elongation 7 1.44E 05 − GO:0022900 Electron transport chain 7 2.88E 05 − GO:0006412 Translation 10 5.53E 05 − GO:0042773 ATP synthesis coupled electron transport 5 2.08E 04 − GO:0042775 Mitochondrial ATP synthesis coupled electron transport 5 2.08E 04 − GO:0006091 Generation of precursor metabolites and energy 9 2.25E 04 − GO:0022904 Respiratory electron transport chain 5 3.49E 04 − GO:0045333 Cellular respiration 5 1.67E 03 − GO:0015980 Energy derivation by oxidation of organic compounds 5 6.89E 03 − Down genes GO:0010628 Positive regulation of gene expression 7 1.25E 03 − GO:0010604 Positive regulation of macromolecule metabolic process 8 1.82E 03 − GO:0007155 Cell adhesion 7 3.21E 03 − GO:0022610 Biological adhesion 7 3.23E 03 − GO:0006357 Regulation of transcription from RNA polymerase II promoter 7 3.87E 03 − GO:0006968 Cellular defense response 3 7.51E 03 − GO:0045935 Positive regulation of nucleobase,
Details
-
File Typepdf
-
Upload Time-
-
Content LanguagesEnglish
-
Upload UserAnonymous/Not logged-in
-
File Pages7 Page
-
File Size-