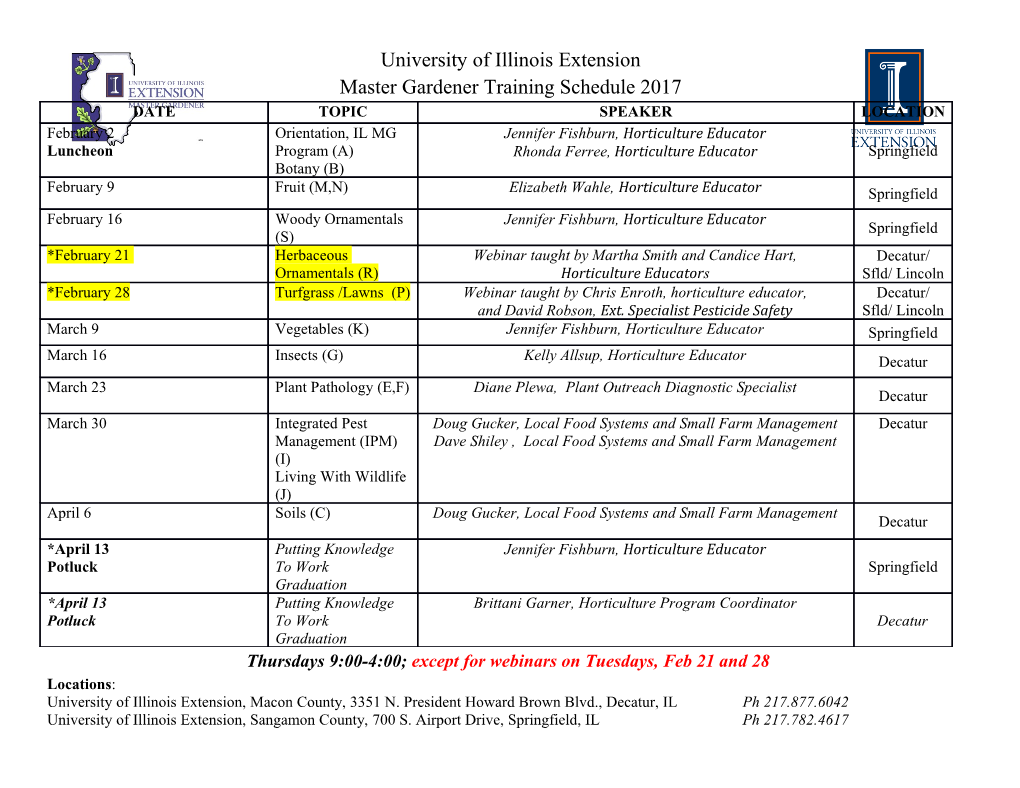
Fundamentals of Chemometrics and Modeling Dr. Tom Dearing CPAC, University of Washington Outline • Fundamentals of Chemometrics – Introduction to Chemometrics – Measurements – The Data Analysis Procedure • Basic Modeling – Principal Component Analysis – Scores and Loadings • Advanced Modeling – Partial Least Squares – Latent Variables – Scores and Loadings – Calibration and Validation – Prediction • Case Study Section 1 Through the looking glass….. Chemometrics • Chemometrics is: The science of extracting information from measurements made on chemical systems with the use of mathematical and statistical procedures. • Keywords and phrases: data analysis, data processing, univariate, multivariate, variance, modeling, scores, loadings, calibration and validations, predictions, real time decision making. Near IR Tablet Data Measurements 7 6.5 • Measurements come in many 6 different forms. 5.5 – Spectroscopic 5 Signal Signal Intensity • Near IR, Fluorescence, Raman. 4.5 – Chromatographic 4 • Gas Chromatography, HPLC. 3.5 3 600 800 1000 1200 1400 1600 1800 2000 – Physical Wavenumber cm-1 • Temperature, Pressure, Flow rate, Melting Points, Viscosity, Concentrations. • All measurements yield data. • NIR data set containing 255 spectra measured at 650 (counts) Intensity different wavenumbers has 165750 data points!! Wavelength (nm) Two Types Of Data • Univariate • Multivariate – One variable to measure – Multiple variables – One variable to predict – Multiple predictions – Typically select one – Typically use entire wavelength and monitor spectra. change of absorbance – Allows investigation into over time. the relationship – Wavelength must not between variables. have contributions or – Allows revealing of overlapping from other latent variation within a peaks. set of spectra. Multivariate Analysis • Analysis performed on multiple sets of measurements, wavelengths, samples and data sets. • Analysis of variance and dependence between variables in crucial to multivariate analysis. The Chemometrics Process • All chemometrics begin with taking a measurement and collecting 5. Understanding data. • Mathematical and statistical methods are employed to extract 4. Knowledge relevant information from the data. • The information is related to the chemical process to extract 3. Information knowledge about a system. • Finally, the knowledge provided 2. Data allows comprehension and understanding of a system. • Understanding facilitates decision 1. Measurement making. Converting Data to Information • Advances in measurement science means rate of data collection is extremely fast. • Large amounts of data produced. • Data rich, information poor. • Chemometrics used to remove redundant data, reduce variation not relating to the analytical signal and build models. Data Analysis Flow Chart OUTLIER INPUT PREPROCESSING REMOVAL DATA ANALYSIS OUTPUT Input • Most overlooked stage of data analysis. • Most critical stage of all. • Data must be converted or transferred into the analysis software. • Proprietary collection software make this task difficult. • However, some analysis software have excellent data importing functionality Outliers – Problems and Removal • Removing outliers is a delicate procedure. • Grubbs test used to detect outliers. • Frequently requires knowledge about the process being examined. • False outliers, samples at extremes of the system that appear infrequently within the data. – These are NOT REMOVED • True outliers, samples or variable that is statistically different from the other samples. – These ARE REMOVED Near IR Tablet Data Preprocessing 7 6.5 • Preprocessing 6 – Main goal of the preprocessing 5.5 stage is to remove variation 5 Signal Signal Intensity within the data that does not 4.5 pertain to the analytical 4 information. 3.5 3 600 800 1000 1200 1400 1600 1800 2000 Wavenumber cm-1 MEAN • Typical preprocessing methods CENTRING – Baseline Correction Mean Centred NIR Spectra – Mean Centering 0.8 0.6 – Normalization 0.4 0.2 – Orthogonal Signal Correction 0 -0.2 Mean Centred Signal Intensity Centred Mean – Multiplicative Scatter Correction -0.4 -0.6 – Savitsky-Golay Derivatisation -0.8 600 800 1000 1200 1400 1600 1800 Wavenumber cm-1 Data Analysis Scores Plot • Many different methods for 3 performing multivariate data 2 1 analysis. 0 -1 • Principal Component Analysis Scores PC on 2 (12.88%) – Section 2 -2 -3 -15 -10 -5 0 5 10 • Scores on PC 1 (81.38%) Partial Least Squares 60 – Section 3 50 • MCR 40 30 • Neural Networks 20 10 0 4.65 4.7 4.75 4.8 4.85 4.9 4.95 5 5.05 5.1 5.15 Output • Qualitative • Quantitative – Classification models. – Prediction models – Does a sample belong to – What is the a group or not?? concentration of the – Calibration and sample?? Validations – Calibration and – Classifications Validations – Classification error – Predictions – Number of samples – Calibration and classified correctly Prediction Errors – RMSEC and RMSEP Error • Many different methods of calculating errors. • Method used is critical as model quality determined by the error. • Procedure used can heavily influence model errors. (Discussed later in PCA section). • The choice of error metric depends on many different factors • Top Three – What are you showing? – What is the range of data? – How many samples do you have? Summary • Chemometrics is a method of extracting relevant information from complex chemical data. • Multivariate data allows analysis robust investigation of overlapping signals. • Multivariate analysis allows investigation of the relationship between variables. • The chemometrics process yields understanding and comprehension of the process under investigation. Summary • Data analysis is a multistep procedure involving many algorithms and many different paths to go down. • The end results of data analysis are commonly a model that could provide qualitative or quantitative information. • MatLab and PLS_Toolbox are software packages used to perform chemometrics analysis. Section 2 Principal Component Analysis P.C.A. PCA • Method of reducing a set of data into three new sets of variables – Principal Components (PC’s) – Scores – Loadings • Using these three new variables latent variation can be developed and examined. • Incredibly important for investigating the relationships between samples and variables PCA • NIR spectra run through a PCA routine without any form of preprocessing. • Scores produced show apparent variation in concentration. • Loadings illustrate the mean spectra, suggesting that preprocessing should be used. Near IR Tablet Data Samples/Scores Plot Variables/Loadings Plot 7 8 0.055 6.5 6 0.05 6 4 0.045 5.5 PCA 2 5 0 0.04 Signal Signal Intensity 4.5 -2 0.035 Scores PC on 2 (0.05%) Loadings on PC on 1 (99.93%) Loadings 4 -4 0.03 3.5 -6 3 -8 0.025 600 800 1000 1200 1400 1600 1800 2000 118 120 122 124 126 128 130 132 134 136 100 200 300 400 500 600 -1 Wavenumber cm Scores on PC 1 (99.93%) Variable SPECTRAL DATA SCORES LOADINGS Principal Components • Each principal component calculated captures as much of the variation within the data as possible. • This variation is removed and a new principal component is determined. • The first PC describes the greatest source of variation within the data Scores • The scores are organized in a column fashion. • The first column denotes the scores relating to the variation captured on PC1. • Intra-sample relationships can be observed by plotting the scores from PC1 against PC2. • This can be expanded to the scores of the first three PC’s. Scores Samples/Scores Plot of aldat Samples/Scores Plot of aldat Samples/Scores Plot of aldat 400 300 150 300 200 100 200 100 50 100 0 0 0 -100 -50 -100 Scores PC on 2 (29.86%) Scores PC on 3 (11.16%) Scores PC on 1 (52.89%) -200 -100 -200 -300 -300 -150 -400 -400 -200 5 10 15 20 25 30 35 40 45 50 55 5 10 15 20 25 30 35 40 45 50 55 5 10 15 20 25 30 35 40 45 50 55 Sample Sample Sample Scores on PC1 Scores on PC2 Scores on PC3 Samples/Scores Plot of aldat 300 Samples/Scores Plot of aldat 150 Samples/Scores Plot of aldat 200 100 100 100 50 50 0 0 0 -50 -100 -50 -100 Scores PC on 2 (29.86%) -200 -150 Scores PC on 3 (11.16%) Scores PC on 3 (11.16%) -100 -200 -300 -150 200 0 -200 -400 -400 200 -400 -300 -200 -100 0 100 200 300 400 -200 -200 0 Scores on PC 1 (52.89%) -400 -300 -200 -100 0 100 200 300 400 Scores on PC 2 (29.86%) Scores on PC 1 (52.89%) Scores on PC 1 (52.89%) Scores of PC1 vs. Scores of PC1 vs. Scores of PC2 PC3 PC1 vs. PC2 vs PC3 Loadings • Illustrate the weight or importance of each variable within the original data. • From loadings it is possible to see the most significant variables. • Loadings can be used to track the process of a reaction e.g. monitor reactant consumption. • Deduce variables responsible for the clustering in the scores. Loadings Variables/Loadings Plot Variables/Loadings Plot 0.055 0.07 0.06 0.05 0.05 0.045 0.04 0.03 0.04 0.02 0.035 0.01 Loadings on PC on 1 (81.38%) Loadings Loadings on PC on 1 (99.93%) Loadings 0 0.03 -0.01 0.025 -0.02 100 200 300 400 500 600 100 200 300 400 500 600 Variable Variable NO PREPROCESSING MEAN CENTRING Variables/Loadings Plot 0.05 0.04 0.03 0.02 0.01 0 Loadings on PC on 1 (62.61%) Loadings -0.01 -0.02 -0.03 100 200 300 400 500 600 Variable AUTO SCALING Outlier Removal • PCA can be used in conjunction with confidence intervals to identify outliers within a set of data. Samples/Scores Plot Samples/Scores Plot 4 6 3 4 2 2 1 0 0 -1 -2 Scores PC on 2 (12.88%) Scores PC on 2 (12.88%) -2 -4 -3 -4 -6 -15 -10 -5 0 5 10 -15 -10 -5 0 5 10 15 Scores on PC 1 (81.38%) Scores on PC 1 (81.38%) 95% Confidence Interval 99.9% Confidence Interval Summary • PCA used to decompose the data into scores and loadings • Scores reveal information about between sample variation.
Details
-
File Typepdf
-
Upload Time-
-
Content LanguagesEnglish
-
Upload UserAnonymous/Not logged-in
-
File Pages65 Page
-
File Size-