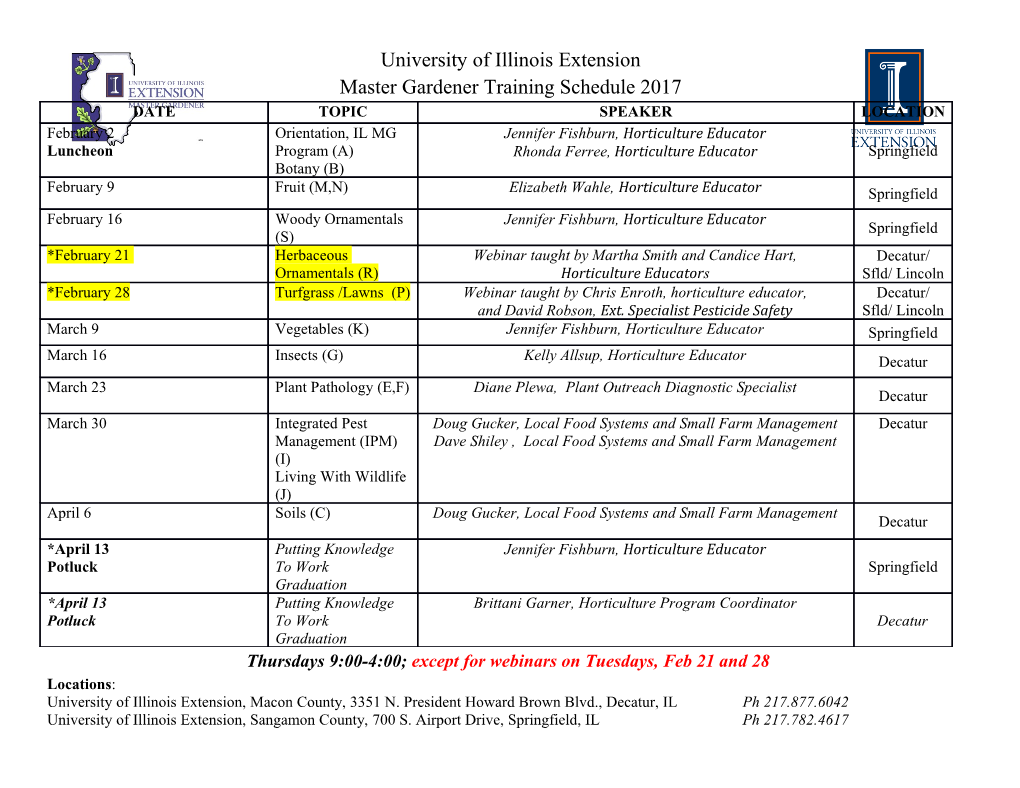
PiCasso: A Lightweight Edge Computing Platform Adisorn Lertsinsrubtavee, Anwaar Ali, Carlos Molina-Jimenez, Arjuna Sathiaseelan and Jon Crowcroft Computer Laboratory, University of Cambridge, UK Email: fi[email protected] Abstract—Recent trends show that deploying low cost devices deploy multiple instances of a given service opportunistically with lightweight virtualisation services is an attractive alternative to ensure that it complies with service requirements. The core for supporting the computational requirements at the network of PiCasso is the orchrestration engine that deploys services edge. Examples include inherently supporting the computational needs for local applications like smart homes and applications on the basis of the service specifications and the status of with stringent Quality of Service (QoS) requirements which are the resources of the hosting devices. Although PiCasso is still naturally hard to satisfy by traditional cloud infrastructures or under development, this paper offers insights into the building supporting multi-access edge computing requirements of network of service deployment platforms (its main contribution). We in the box type solutions. The implementation of such platform demonstrate that the effort involves the execution of practical demands precise knowledge of several key system parameters, including the load that a service can tolerate and the number experiments to yield results to identified the parameters that of service instances that a device can host. In this paper, we the orchrestration engine needs to take into account. introduce PiCasso, a platform for lightweight service orchestra- tion at the edges, and discuss the benchmarking results aimed II. RELATED WORK at identifying the critical parameters that PiCasso needs to take Several works [3]–[6] have explored the benefits of into consideration. lightweight service deployment through a deployment scheme I. INTRODUCTION similar to ours i.e., based on microservices (e.g., Docker Latest advances in lightweight OS virtualisation technolo- containers) running in low cost hardware substrate. The insight gies such as Docker and Unikernels allow service providers of these studies is that pushing services from centralised clouds to deploy and replicate their services on demand at the edge to the edge can potentially improve end users’ experience, of the network i.e. for supporting edge/fog computing. The in particular, for latency sensitive applications. However, the motivation for this approach is to improve the QoS (latency policies to deploy the service instances are not discussed. Our in particular), provide high level of security (provided by the work addresses this issue, by the use of an intelligent orches- strict isolation capabilities of technologies such as Unikernels) tration engine that decides when/where to deploy a service and provide privacy. An edge cloud can be built on the basis of instance to meet its requirements. A deployment policy that several hardware technologies (e.g., rack of servers), however takes into account startup latencies (instantiation) is discussed clouds built by clusters of single-board devices (e.g., Rasp- in [7]. According to this work a service is deployed when the berry Pi, Cubie boards etc) are drawing significant attention overall latency incurred is more than the life expectancy of for several reasons: these devices are cheap, consume low the application itself. A limitation of this solution is that it energy and (if optimised) have enough resources to support considers only the deployment cost and overlooks the status applications of practical interest such as those used in smart of the resources of underlying hardware. This issue is a major cities [1]. The aim of optimisation is to maximize the use of concern in our work. The authors in [8] point out that the running devices so that we can deliver the service with as less load inflicted on the hardware can significantly impact the as possible of them without compromising the necessary QoS network performance in centralised clouds. In our work, we requirements of applications. are concerned about similar issues but in resource constrained To overcome this challenge, the service provider can benefit clouds deployed at the edge. from flexibility of a lightweight service deployment infras- Related to PiCasso are container orchestration technologies tructure [2] that provides on-demand computing capacity and such as Docker Swarm, Mesos and Kubernetes that are used enables elastic service provisioning. For instance, a service to orchestrate and manage service instances in both cen- image can be migrated from the service repository and au- tralised and edge clouds [9]–[11]. Although they provide some tomatically instantiated on the edge device after receiving a automation and load balancing functions, they still rely on service request from the end-user. With this approach, the manual intervention in the administration and orchestration of service provider can opportunistically aggregate idle resources the services. In our work, we aim at an intelligent orchestration from widely distributed computational devices demonstrate the engine capable of performing the managerial tasks much like huge potential of pool resources to build highly scalable, low Docker Swarm and other tools but in an automated fashion cost and easily deployable platforms. that is supported by the monitoring of resources. This paper contributes to cover the research gap. It intro- Frameworks such as MuSIC [12] and MapCloud [13] pro- duces PiCasso – a platform for deploying QoS-sensitive ser- posed the dynamic service allocation algorithms to improve vices in edge clouds built of single board devices. PiCasso can QoS while considering factors like application delay, device power consumption, user cost and user mobility patterns. Manager where the orchestration engine uses it for deciding Similarly, PiCasso aims to develop intelligent service orches- on deployments. tration algorithms but we consider other factors such as the 2) Service Execution allows the edge node to instantiate current workload of underlying hardwares and characteristics containers automatically. It provides an API that allows edge of different container applications. nodes to receive Docker images and json obj with deployment description from the Service Orchestrator. The following json III. PICASSO object is a deployment descriptor to instantiate a httpd-busybox 1 PiCasso is a platform for service deployment at the edge web server. of the network. Its architecture is shown in Fig. 1. The current deploy_descriptor={ implementation is developed in Python. It assumes a network ’imageName’: ’hypriot/rpi-busybox- httpd:latest’, provider-centric model where the provider is in full control of ’port_host’: ’80’ the communication infrastructure. PiCasso has the following ’port_container’: ’8083’} components: Without loss of generality, further parameters can be added Service Orchestrator to the json object to enable the deployment of more sophisti- Service Providers cated services. Service Repo 3) Service Proxy is an intermediary that seeks the service Monitoring Orchestration instance for clients. A user’ s request is intercepted at the Manager Engine local edge node and forwarded to a running container that serves a requested service. If the service is not available in Edge Node#1 Monitoring Service the local edge node, the user’s request will be forwarded to Agent Execution Edge Node#2 the closet edge node hosting the requested service. To improve S S1 S2 3 Sn Monitoring Service the performance and reliability, a particular service can be de- Agent Execution Service Proxy ployed in several containers. These containers (replica) can be S S S S 1 2 3 n deployed either in the same edge node or multiple edge nodes Service Proxy across the network edge. The Service Proxy also includes load balancing function where it distributes the users’ requests 2 Fig. 1: PiCasso’s architecture. across multiple containers. We intend to use HAProxy to develop the service proxy and integrate it with the edge node. A. Edge node B. Service Orchestrator An edge node is a single board computer such as a Rasp- berry Pi (RPi) or any device with storage, CPU and software The service orchestrator is a central entity of PiCasso which facilities for hosting (upon request of the service orchestrator) is responsible for making an informative decision to deploy the the execution of microservices. Each edge node is provided services. The design of service orchestrator is shown in Fig. 2. with Monitoring Agent, Service Execution and Service Proxy 1) Orchestration Engine (OE) implements the logic for functionalities. deployment of instances of services to meet specific QoS 1) Monitoring Agent is responsible for measuring the current requirements. OE has access to an algorithm repository that status of resources and the current demand imposed on the can execute to make decisions on deployment of instances services. The monitored data is formatted as json objects. The of services. For instance, an edge node can rapidly become code below shows the actual data collected from an edge node, exhausted when a number of user requests are placed against a called \SEG 1". particular service at the same time. This can significantly result {"softResources":{"OS": "Linux"}, in large response time perceived by the end-users. To mitigate "hardResources": {"mem": "1 GB", "disk": "16 GB", "cpu": "A 1.2GHz quad-core ARMv8 CPU"}, this problem, the orchestration engine
Details
-
File Typepdf
-
Upload Time-
-
Content LanguagesEnglish
-
Upload UserAnonymous/Not logged-in
-
File Pages7 Page
-
File Size-