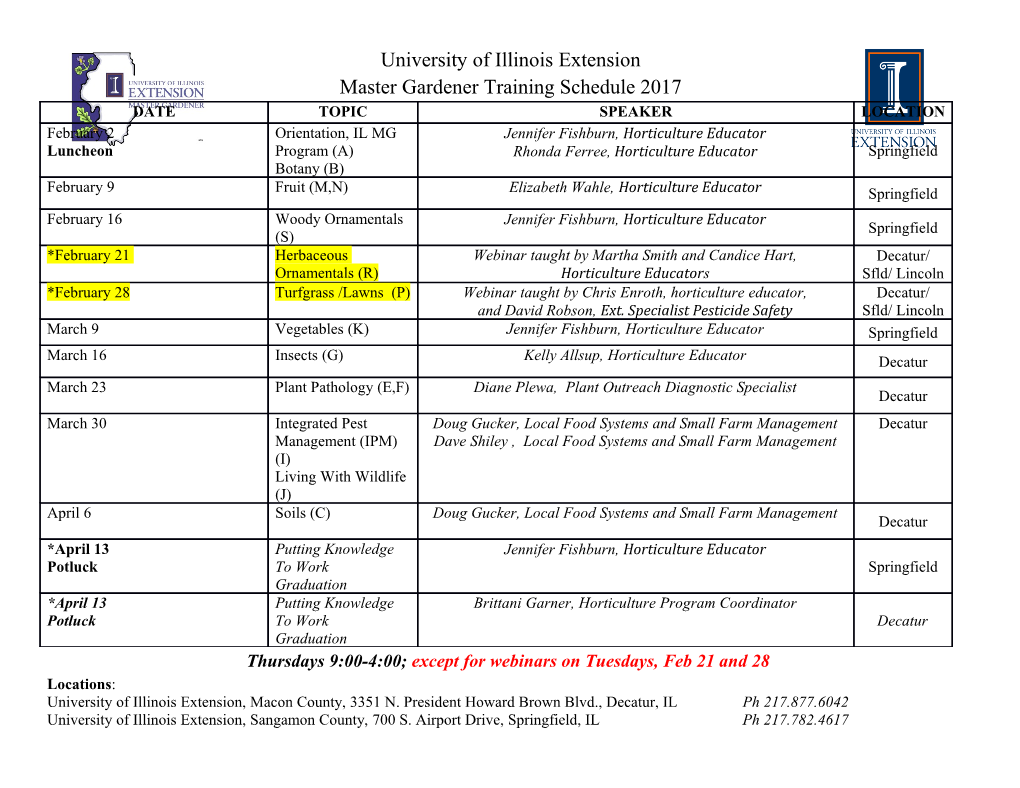
Exact Sparse Approximation Problems via Mixed-Integer Programming: Formulations and Computational Performance Sébastien Bourguignon, Jordan Ninin, Hervé Carfantan, Marcel Mongeau To cite this version: Sébastien Bourguignon, Jordan Ninin, Hervé Carfantan, Marcel Mongeau. Exact Sparse Approxi- mation Problems via Mixed-Integer Programming: Formulations and Computational Performance. IEEE Transactions on Signal Processing, Institute of Electrical and Electronics Engineers, 2016, 64 (6), pp.1405-1419. 10.1109/TSP.2015.2496367. hal-01254856 HAL Id: hal-01254856 https://hal.archives-ouvertes.fr/hal-01254856 Submitted on 12 Jan 2016 HAL is a multi-disciplinary open access L’archive ouverte pluridisciplinaire HAL, est archive for the deposit and dissemination of sci- destinée au dépôt et à la diffusion de documents entific research documents, whether they are pub- scientifiques de niveau recherche, publiés ou non, lished or not. The documents may come from émanant des établissements d’enseignement et de teaching and research institutions in France or recherche français ou étrangers, des laboratoires abroad, or from public or private research centers. publics ou privés. 1 Exact Sparse Approximation Problems via Mixed-Integer Programming: Formulations and Computational Performance Sebastien´ Bourguignon, Jordan Ninin, Herve´ Carfantan, and Marcel Mongeau, Member, IEEE Abstract—Sparse approximation addresses the problem of too difficult in practical large-scale instances. Indeed, the approximately fitting a linear model with a solution having as few Q brute-force approach that amounts to exploring all the K non-zero components as possible. While most sparse estimation possible combinations, is computationally prohibitive. In the algorithms rely on suboptimal formulations, this work studies the abundant literature on sparse approximation, much work has performance of exact optimization of `0-norm-based problems through Mixed-Integer Programs (MIPs). Nine different sparse been dedicated to the relaxation approach that replaces the `0- optimization problems are formulated based on `1, `2 or `1 “norm” sparsity measure, x 0 := Card i xi = 0 , with the data misfit measures, and involving whether constrained or P k k f j 6 g `1 norm x 1 := i xi . Many specific convex optimization penalized formulations. For each problem, MIP reformulations algorithmsk havek beenj proposedj in the past decade, see for allow exact optimization, with optimality proof, for moderate-size yet difficult sparse estimation problems. Algorithmic efficiency of example [1], [2]. In addition, conditions were established all formulations is evaluated on sparse deconvolution problems. for which both the `0 and the relaxed `1 problems yield This study promotes error-constrained minimization of the `0 the same solution support (the set of non-zero components). norm as the most efficient choice when associated with `1 and These mostly rely on a low sparsity level assumption and `1 misfits, while the `2 misfit is more efficiently optimized on structural hypotheses on the matrix H, such as low with sparsity-constrained and sparsity-penalized problems. Then, correlation of its columns (see [1] and references therein). exact `0-norm optimization is shown to outperform classical methods in terms of solution quality, both for over- and under- Alternatively, greedy algorithms build a sparse solution by determined problems. Finally, numerical simulations emphasize iteratively adding non-zero components to an initially empty- the relevance of the different `p fitting possibilities as a function support solution [3], [4], [5]. More complex forward-backward of the noise statistical distribution. Such exact approaches are methods [6], [7] may show better performance in practice but shown to be an efficient alternative, in moderate dimension, to classical (suboptimal) sparse approximation algorithms with with higher computation time. Tree-search-based methods also `2 data misfit. They also provide an algorithmic solution to try to improve the classical greedy algorithms using heuristics less common sparse optimization problems based on `1 and to reduce the complexity of exhaustive combinatorial explo- `1 misfits. For each formulation, simulated test problems are ration (see e.g., [8] and references therein). Other support proposed where optima have been successfully computed. Data exploration strategies maintain the desired sparsity level at and optimal solutions are made available as potential benchmarks for evaluating other sparse approximation methods. each iteration, and perform local combinatorial exploration steps [9]. Optimality proofs for all such strategies also rely Index Terms—sparse approximation, `0-norm-based problems, on very restrictive hypotheses [10], [11]. More “` -oriented” optimization, mixed-integer programming, deconvolution 0 approaches were proposed, e.g., by successive continuous approximations of the `0 norm [12], by descent-based Iterative I. INTRODUCTION Hard Thresholding (IHT) [13], [14] and by penalty decompo- A. Sparse estimation for inverse problems sition methods [15]. However, without additional assumptions on H, one can only prove that the solution found is a local The problem of sparse representation of data y RN in 2 minimum of the optimization problem. Moreover, for IHT, a dictionary H RN RQ consists in finding a solution 2 × optimality conditions suffer from the same restrictions as the x RQ to the system y = Hx with the fewest non-zero components,2 i.e., with the lowest sparsity level. In sparse aforementioned greedy methods [13], [14]. In many inverse problems, the model y Hx results approximation, in order to account for noise and model errors, ' the equality constraint is relaxed through the minimization from the discretization of an intrinsically continuous physical of the data misfit measure y Hx , where generally model. A typical example is sparse deconvolution, where Hx k − k k·k models the convolution of a spike train (in one-dimensional stands for the standard Euclidean norm in RN . Such sparsest representation and approximation problems are essentially signals) or of point sources (in imaging problems) by the combinatorial. Finding the best K-sparse solution (the so- impulse response of the acquisition device [7], [16], [17]. A lution with K non-zero components) is usually considered similar problem concerns nonlinear parameter identification, where parameters are discretized on arbitrarily thin grids [7], This work was partially supported by the French Groupement de Recherche [18], [19] and estimation amounts to finding a sparse solution ISIS (Information, Signal, Image, viSion) through its “young researchers” to a linear problem of high dimension. In such cases, the program. One of the authors of this work has been supported by French National Research Agency (ANR) through JCJC program (project ATOMIC columns of H can be highly correlated, so no optimality guar- ◦ n ANR 12-JS02-009-01). anty can be obtained for greedy and `1-norm-based methods. 2 Similar problems also arise for variable selection in machine with binary variables appears in [25], but binary variables are learning and statistics [6], where the set of features (the replaced by continuous variables in [0; 1] in order to yield columns of H) is not designed to satisfy any recovery property. a convex problem, which is obviously not equivalent to the The aforementioned problems essentially focus on the cor- original one. In [26], some exact and approximate reformula- rect estimation of the support of x. In deconvolution, for tions of `0-based problems are surveyed. The authors deplore example, support identification corresponds to detection and the inherent combinatorial difficulty of such MIP problems localization of the sources. Since the true sparsity measure but no practical result is provided. Finally, in [27], noise- is indeed the `0 norm, a global optimum of `0-based for- free sparse representation problems are formulated as MIP. mulations is more likely to yield exact support identification Here, we address the noisy case, which opens perspectives to than approximate solutions. Consequently, our interest focuses different possible formulations of the optimization problem. on optimization methods for `0-norm-based criteria provid- Establishing MIP reformulations for such problems, studying ing optimality guarantees. Such exact approaches are usually their computational efficiency, investigating properties of opti- discarded, based on the argument that sparse optimization mal solutions and comparing them with the results of standard problems are NP hard [20]. It is also commonly considered methods are the core of this paper. that exact optimization amounts to combinatorial exploration of all possible supports, which is nothing but a worst-case- scenario argument. Note however that, in order to reduce the C. Objectives of the paper number of explored supports, Tropp and Wright [1] mentioned This paper shows that different possible reformulations the possible use of cutting-plane methods, which are one of of sparse approximation problems can be tackled by MIP the basic elements of resolution of the mixed-integer programs solvers. Sparse approximation is intrinsically a bi-objective explored in the present paper. optimization problem, where both the sparsity measure and Here, we focus on sparse optimization occurring in certain the data misfit measure are optimized. In inverse problems, it inverse problems with moderate size yet with a complexity suf- is usually formulated through the optimization
Details
-
File Typepdf
-
Upload Time-
-
Content LanguagesEnglish
-
Upload UserAnonymous/Not logged-in
-
File Pages16 Page
-
File Size-