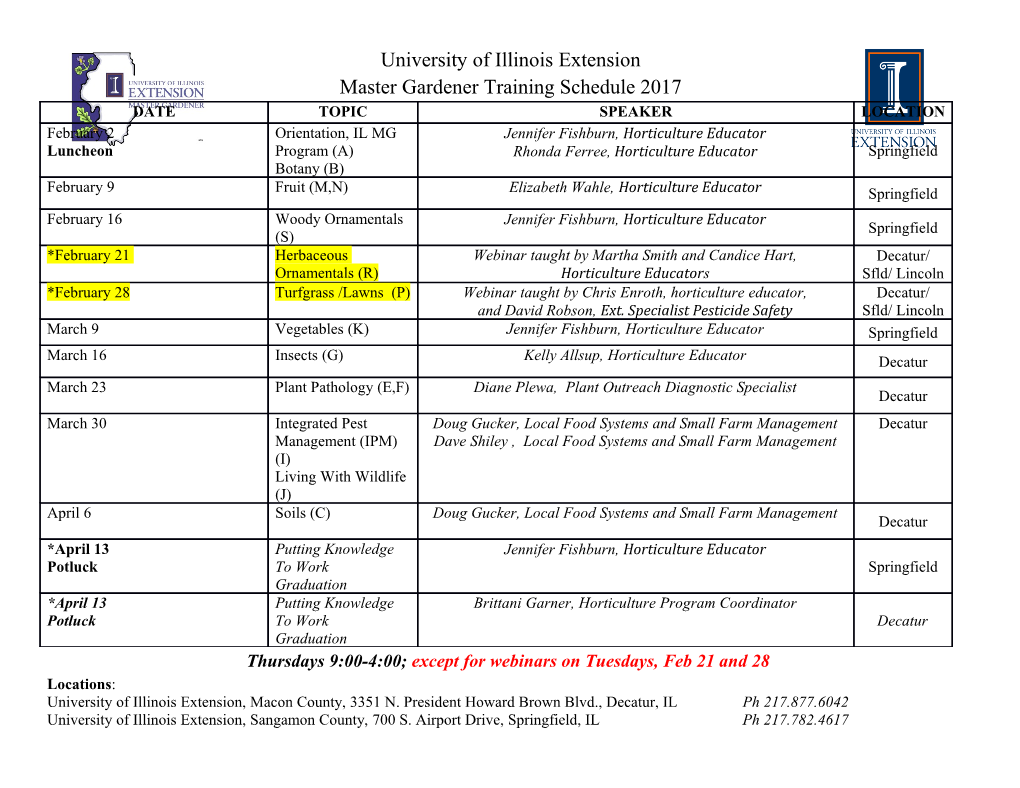
1 THE EFFECTIVENESS OF OPTION PRICING MODELS DURING FINANCIAL CRISES Camillo Lento* and Nikola Gradojevic** *Lakehead University, **Lakehead University and The Rimini Centre for Economic Analysis CHAPTER OUTLINE 1.1 Introduction 1 1.2 Methodology 4 1.3 Data 6 1.4 Results 7 1.5 Concluding Remarks 10 References 11 1.1 Introduction Options can play an important role in an investment strategy. For example, options can be used to limit an investor’s downside risk or be employed as part of a hedging strategy. Accordingly, the pricing of options is important for the overall efficiency of capital markets.1 The purpose of this chapter is to explore the effec- tiveness of the original Black and Scholes (1973) option pricing model (BS model) against a more complicated non-parametric neural network option pricing model with a hint (NN model). Specifically, this chapter compares the effectiveness of the BS model versus the NN model during periods of stable economic conditions and economic crisis conditions. Past literature suggests that the standard assumptions of the BS model are rarely satisfied. For instance, the well-docu- mented “volatility smile” and “volatility smirk” (Bakshi et al., 1997) pricing biases violate the BS model assumption of 1 Readers interested in a detailed survey of the literature on option pricing are encouraged to review Garcia et al. (2010) and Renault (2010). Rethinking Valuation and Pricing Models. http://dx.doi.org/10.1016/B978-0-12-415875-7.00001-4 Copyright Ó 2013 Elsevier Inc. All rights reserved. 1 2 Chapter 1 THE EFFECTIVENESS OF OPTION PRICING MODELS DURING FINANCIAL CRISES constant volatility. Additionally, stock returns have been shown to exhibit non-normality and jumps. Finally, biases also occur across option maturities, as options with less than three months to expiration tend to be overpriced by the BlackeScholes formula (Black, 1975). In order to address the biases of the BS model, research efforts have focused on developing parametric and non-parametric models. With regard to parametric models, the research has mainly focused on three models: The stochastic volatility (SV), stochastic volatility random jump (SVJ) and stochastic interest rate (SI) parametric models. All three models have been shown to be superior to the BS model in out-of-sample pricing and hedging exercises (Bakshi et al., 1997). Specifically, the SV model has been shown to have first-order importance over the BS model (Gencay and Gibson, 2009). The SVJ model further enhances the SV model for pricing short-term options, while the SI model extends the SVJ model in regards to the pricing of long-term options (Gencay and Gibson, 2009). Although parametric models appear to be a panacea with regard to relaxing the assumptions that underlie the BS model, while simultaneously improving pricing accuracy, these models exhibit some moneyness-related biases for short-term options. In addition, the pricing improvements produced by these para- metric models are generally not robust (Gencay and Gibson, 2009; Gradojevic et al., 2009). Accordingly, research also explores non-parametric models as an alternative, (Wu, 2005). The non- parametric approaches to option pricing have been used by Hutchinson et al. (1994), Garcia and Gencay (2000), Gencay and Altay-Salih (2003), Gencay and Gibson (2009), and Gradojevic et al. (2009). Non-parametric models, which lack the theoretical appeal of parametric models, are also known as data-driven approaches because they do not constrain the distribution of the underlying returns (Gradojevic et al., 2011). Non-parametric models are superior to parametric models at dealing with jumps, non- stationarity and negative skewness because they rely upon flexible function forms and adaptive learning capabilities (Agliardi and Agliardi, 2009; Yoshida, 2003). Generally, non- parametric models are based on a difficult tradeoff between rightness of fit and smoothness, which is controlled by the choice of parameters in the estimation procedure. This tradeoff may result in a lack of stability, impeding the out-of-sample performance of the model. Regardless, non-parametric models have been shown to be more effective than parametric models at relaxing BS model assumptions (Gencay and Gibson, Chapter 1 THE EFFECTIVENESS OF OPTION PRICING MODELS DURING FINANCIAL CRISES 3 2009; Gradojevic and Kukolj, 2011; Gradojevic et al., 2009). Accordingly, the BS model is compared against a non-para- metric option pricing model in this chapter. Given its currency, little research has been conducted on the effectiveness of option pricing during the 2008 financial crisis. However, the 1987 stock market crash has proved to be fertile grounds for research with regard to option pricing during periods of financial distress. For example, Bates (1991, 2000) identified an option pricing anomaly just prior to the October 1987 crash. Specifically, out-of-the-money American put options on S&P 500 Index futures were unusually expensive relative to out-of-the- money calls. In a similar line of research, Gencay and Gradojevic (2010) used the skewness premium of European options to develop a framework to identify aggregate market fears to predict the 1987 market crash. This chapter expands the option pricing literature by comparing the accuracy of the BS model against NN models during the normal, pre-crisis economic conditions of 1987 and 2008 (the first quarter of each respective year) against the crisis conditions of 1987 and 2008 (the fourth quarter of each respective year). Therefore, this work also provides new and novel insights into the accuracy of option pricing models during the recent 2008 credit crisis. The results suggest that the more complicated NN models are more accurate during stable markets than the BS model. This result is consistent with the past literature that suggest non- parametric models are superior to the BS model (e.g. Gencay and Gibson, 2009; Gradojevic et al., 2009). However, the results during the periods of high volatility are counterintuitive as they suggest that the simpler BS model is superior to the NN model. These results suggest that a regime switch from stable economic conditions to periods of excessively volatile conditions impedes the estimation and the pricing ability of non-parametric models. In addition to the regime shift explanation, considerations should be given to the fact that the BS model is a pre-specified non- linearity and its structure (shape) does not depend on the esti- mation dataset. This lack of flexibility and adaptability appears to be beneficial when pricing options in crisis periods. It conclusion, it appears as if the learning ability and flexibility of non-para- metric models largely contributes to their poor performance relative to parametric models when markets are highly volatile and experience a regime shift. The results make a contribution that is relevant to academic and practitioners alike. With the recent financial crisis of 2007e2009 creating pitfalls for various asset valuation models, 4 Chapter 1 THE EFFECTIVENESS OF OPTION PRICING MODELS DURING FINANCIAL CRISES this chapter provides practical advice to investors and traders with regard to the most effective model for option pricing during times of economic turbulence. In addition, the results make a contribution to the theoretical literature that investigates the BS model versus its parametric and non-parametric counterparts by suggesting that the efficacy of the option pricing model depends on the economic conditions. The remainder of this chapter is organized as follows: Section 1.2 outlines the methodology, Section 1.3 discusses the data, Section 1.4 presents the results and Section 1.5 provides concluding remarks. 1.2 Methodology The option pricing formula is defined as in Hutchinson et al. (1994) and Garcia and Genc¸ay (2000): Ct ¼ f ðSt; K ; sÞ; ð1:1Þ where Ct is the call option price, St is the price of the underlying asset, K is the strike price and s is the time to maturity (number of days). Assuming the homogeneity of degree one of the pricing function f with respect to St and K, one can write the option pricing function as follows: 0 1 B C C B S C t B t C ¼ f B ; 1; s C ¼ f ðx1; x2Þ: ð1:2Þ |{z}K @ |{z}K |{z} A x Ct x1 2 We extend the model in Equation (1.2) with two additional inputsdthe implied volatility and the risk-free interest rate: S c ¼ f t ; s; ; r ¼ f ðx ; x ; x ; x Þð: Þ t K s1 1 2 3 4 1 3 We estimate Equation (1.3) non-parametrically using a feedfor- ward NN model with the “hint” from Garcia and Genc¸ay (2000). This model is an improvement on the standard feedforward NN methodology that provides superior pricing accuracy. Moreover, when Genc¸ay and Gibson (2009) compared the out-of-sample performance of the NN model to standard parametric approaches (SV, SVJ and SI models) for the S&P 500 Index, they found that the NN model with the generalized autoregressive conditional het- eroskedasticity GARCH(1,1) volatility dominates the parametric models over various moneyness and maturity ranges. The supe- riority of the NN model can be explained by its adaptive learning Chapter 1 THE EFFECTIVENESS OF OPTION PRICING MODELS DURING FINANCIAL CRISES 5 and the fact that it does not constrain the distribution of the underlying returns. The “hint” involves utilizing additional prior information about the properties of an unknown (pricing) function that is used to guide the learning process. This means breaking up the pricing function into four parts, controlled by x1, x2, x3 and x4. Each part contains a cumulative distribution function
Details
-
File Typepdf
-
Upload Time-
-
Content LanguagesEnglish
-
Upload UserAnonymous/Not logged-in
-
File Pages625 Page
-
File Size-