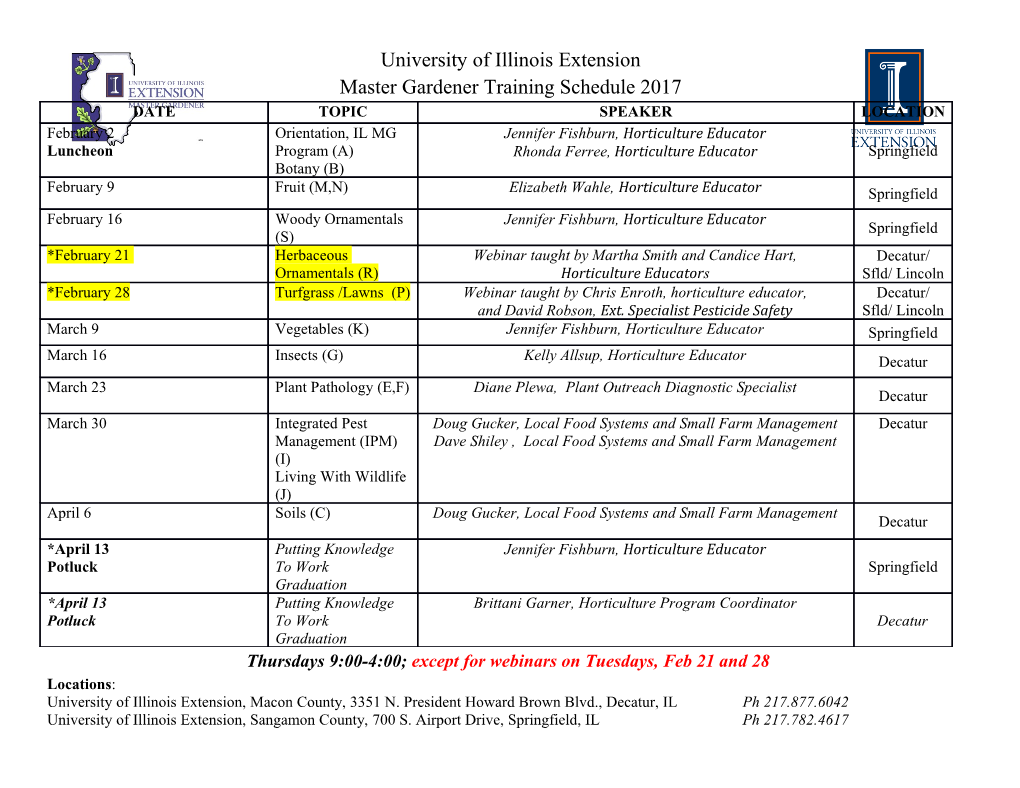
UC Irvine UC Irvine Electronic Theses and Dissertations Title Statistical Methods for the Forensic Analysis of User-Event Data Permalink https://escholarship.org/uc/item/8s22s5kb Author Galbraith, Christopher Michael Publication Date 2020 License https://creativecommons.org/licenses/by/4.0/ 4.0 Peer reviewed|Thesis/dissertation eScholarship.org Powered by the California Digital Library University of California UNIVERSITY OF CALIFORNIA, IRVINE Statistical Methods for the Forensic Analysis of User-Event Data DISSERTATION submitted in partial satisfaction of the requirements for the degree of DOCTOR OF PHILOSOPHY in Statistics by Christopher Galbraith Dissertation Committee: Chancellor’s Professor Padhraic Smyth, Chair Chancellor’s Professor Hal S. Stern Associate Professor Veronica Berrocal 2020 c 2020 Christopher Galbraith DEDICATION To my parents, Lynn & Larry ii TABLE OF CONTENTS Page LIST OF FIGURES vi LIST OF TABLES x LIST OF ALGORITHMS xi ACKNOWLEDGMENTS xii VITA xiv ABSTRACT OF THE DISSERTATION xvi 1 Introduction 1 1.1 Outline & Contributions . 4 2 Computing Strength of Evidence with the Likelihood Ratio 8 2.1 Evidence Types . 8 2.1.1 Biological (DNA) Evidence . 9 2.1.2 Trace Evidence . 10 2.1.3 Pattern Evidence . 11 2.2 Formal Problem Statement . 12 2.2.1 Source Propositions . 12 2.3 The Likelihood Ratio . 15 2.3.1 The LR as a Bayesian Method . 17 2.3.2 Estimation . 18 2.3.3 Interpretation . 21 2.4 Population Data . 22 2.4.1 Reference Data . 24 2.4.2 Validation Data . 24 2.4.3 Leave-pairs-out Cross-validation . 25 2.5 Empirical Classification Performance . 25 2.6 Information-theoretic Evaluation . 27 2.6.1 Uncertainty and Information . 28 2.6.2 Choosing the Target Distribution . 32 2.6.3 Strictly Proper Scoring Rules & Bayes Risk . 33 iii 2.6.4 Empirical Cross-Entropy (ECE) . 37 2.6.5 Log Likelihood Ratio Cost . 38 2.6.6 The ECE Plot . 39 2.7 Empirical Calibration . 41 2.7.1 Calibration in General . 42 2.7.2 Using LR Values to Calibrate Posterior Probabilities . 43 2.7.3 Isotonic Regression & the PAV Algorithm . 43 2.7.4 Obtaining Calibrated LR Values . 45 2.7.5 Assessing the Calibrated LR Values . 45 2.8 Discussion . 48 2.8.1 Contributions . 49 3 Score-Based Approaches for Computing the Strength of Evidence 50 3.1 The Score-based Likelihood Ratio . 51 3.1.1 Choosing an Appropriate Score Function . 53 3.1.2 Estimation . 54 3.2 The Coincidental Match Probability . 58 3.2.1 Estimation . 60 3.2.2 Interpretation . 62 3.3 Examples with Known Distributions . 63 3.3.1 Likelihood Ratio . 64 3.3.2 Score-based Likelihood Ratio . 65 3.3.3 Coincidental Match Probability . 66 3.3.4 Comparison . 67 3.4 Discussion . 71 3.4.1 Contributions . 72 4 Spatial Event Data 73 4.1 Motivating Example . 74 4.2 Related Work . 76 4.3 Forensic Question of Interest . 77 4.4 Computing the Likelihood Ratio . 80 4.4.1 Adaptive Bandwidth Kernel Density Estimators . 83 4.4.2 Choosing the Mixture Parameter . 84 4.5 Score Functions for Geolocation Data . 87 4.5.1 Nearest Neighbor Distances . 88 4.5.2 Earth Mover’s Distance . 89 4.5.3 Geoparcel Data . 91 4.5.4 Weighting Events . 92 4.6 Score-based Techniques . 94 4.6.1 Score-based Likelihood Ratio . 94 4.6.2 Coincidental Match Probability . 95 4.7 Case Study—Twitter Data . 95 4.7.1 Event Data . 95 4.7.2 Geoparcel Data . 97 iv 4.7.3 Results . 98 4.7.4 Error Analysis . 104 4.7.5 Discussion of Twitter Results . 107 4.8 Case Study—Gowalla Data . 108 4.8.1 Data . 108 4.8.2 Results . 111 4.8.3 Discussion of Gowalla Results . 114 4.9 Discussion . 115 4.9.1 Contributions . 117 5 Temporal Event Data 119 5.1 Motivating Example . 120 5.2 Forensic Question of Interest . 121 5.3 Related Work . 124 5.4 Score Functions for Temporal Event Data . 126 5.4.1 Marked Point Process Indices . 127 5.4.2 Inter-Event Times . 129 5.5 Quantifying Strength of Evidence . 129 5.5.1 Population-based Approach . 130 5.5.2 Resampling Approach . 131 5.6 Case Study—Simulated Data . 135 5.6.1 Simulating Temporal Marked Point Processes . 135 5.6.2 Results . 137 5.6.3 Discussion of Simulation Results . 142 5.7 Case Study—Student Web Browsing Data . 143 5.7.1 Data . 143 5.7.2 Population-based Results . 144 5.7.3 Resampling Results . 148 5.7.4 Discussion of Student Web Browsing Results . 149 5.8 Case Study—LANL Authentication Data . 150 5.8.1 Data . 150 5.8.2 Results . 151 5.9 Discussion . 152 5.9.1 Contributions . 153 6 Discussion on Future Directions 154 Bibliography 159 Appendix A Spatial Results—Twitter Data 169 Appendix B Spatial Results—Gowalla Data 176 Appendix C Signal-to-Noise Ratio Calculation 179 v LIST OF FIGURES Page 1.1 Example of temporal evidence from two individuals (i and j) taken from the case study of Chapter 5. A and B events generated by the same individual tend to cluster temporally, with less clustering in time for A and B events from different users. 4 2.1 Prior entropy UP (Hs) (logarithm base 2) as a function of the prior probability P (Hs)........................................ 29 2.2 Logarithmic scoring rule (base 2) as a loss function. 34 2.3 Empirical cross-entropy (ECE) plot for case study data from Chapter 4. Cllr values are the ECE evaluated at prior log odds of 0 and are given in the legend. Lower values are indicative of better performance on the validation data. 40 2.4 Empirical cross-entropy (ECE) plot of Figure 2.3 including the PAV calibrated LR values. Cllr values are the ECE evaluated at prior log odds of 0 and are given in the legend. Lower values are indicative of better performance on the validation data. 46 3.1 Hypothetical illustration of the conditional densities of the score function ∆ under the hypotheses that the samples are from the same source (Hs, dashed line) and that the samples are from different sources (Hd, solid line). The score-based likelihood ratio SLR∆ is the ratio of the conditional density func- tions g evaluated at δ. .............................. 52 3.2 Hypothetical illustration of the densities of the score function ∆ under the hypotheses that the samples are from the same source (Hs, dashed line) and that the samples are from different sources (Hd, solid line). The coincidental match probability CMP∆ is the shaded tail region of g(∆(A; B)jHd;I). 61 3.3 Behavior of the various methods for evaluating evidence under known distri- butional forms. The value B = b was fixed to the mean of the same-source distribution, µB = 0, to eliminate one source of variability in the analysis. Columns represent a selection of values for the parameters µP , σ!, and σβ. (Top row) Distribution of A under Hs and Hd; (middle row) behavior of the LR and SLR as a function of A = a; (bottom row) behavior of the CMP as a function of A = a.................................. 68 3.4 Contour plots of the various evidence evaluation methods for the example with known distributions where µB = 0, µP = −4, σ! = 0:5, and σβ = 1.
Details
-
File Typepdf
-
Upload Time-
-
Content LanguagesEnglish
-
Upload UserAnonymous/Not logged-in
-
File Pages199 Page
-
File Size-