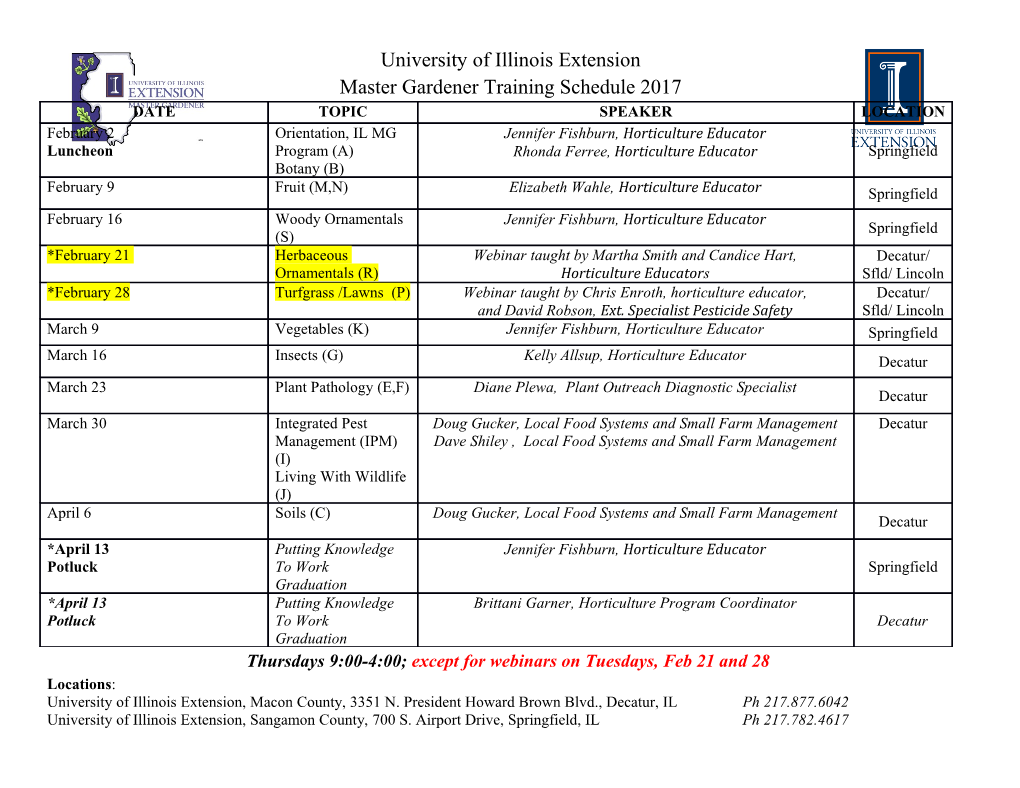
Convolutional operators in the time-frequency domain Vincent Lostanlen To cite this version: Vincent Lostanlen. Convolutional operators in the time-frequency domain. Signal and Image Process- ing. Université Paris sciences et lettres, 2017. English. NNT : 2017PSLEE012. tel-01559667 HAL Id: tel-01559667 https://tel.archives-ouvertes.fr/tel-01559667 Submitted on 10 Jul 2017 HAL is a multi-disciplinary open access L’archive ouverte pluridisciplinaire HAL, est archive for the deposit and dissemination of sci- destinée au dépôt et à la diffusion de documents entific research documents, whether they are pub- scientifiques de niveau recherche, publiés ou non, lished or not. The documents may come from émanant des établissements d’enseignement et de teaching and research institutions in France or recherche français ou étrangers, des laboratoires abroad, or from public or private research centers. publics ou privés. THÈSE DE DOCTORAT de l’Université de recherche Paris Sciences et Lettres PSL Research University Préparée à l’École normale supérieure Convolutional Operators in the Time-frequency Domain Opérateurs convolutionnels dans le plan temps-fréquence École doctorale n°386 SCIENCES MATHÉMATIQUES DE PARIS CENTRE Spécialité INFORMATIQUE COMPOSITION DU JURY : M. GLOTIN Hervé LSIS, AMU, Université de Toulon, ENSAM, CNRS, président du jury M. PEETERS Geoffroy STMS, Ircam, Université Pierre et Marie Curie, CNRS, rapporteur M. RICHARD Gaël LTCI, TELECOM ParisTech, Université Paris-Saclay, CNRS, rapporteur M. MALLAT Stéphane Soutenue par VINCENT LOSTANLEN DI, École normale supérieure, CNRS, le 2 février 2017 membre du jury h M. LAGRANGE Mathieu IRCCyN, École centrale de Nantes, CNRS, membre du jury Dirigée par Stéphane MALLAT M. SHAMMA Shihab LSP, École normale supérieure, CNRS, h membre du jury ! CONVOLUTIONAL OPERATORS IN THE TIME-FREQUENCY DOMAIN VINCENT LOSTANLEN Département d’informatique École normale supérieure Vincent Lostanlen: Convolutional operators in the time-frequency domain. This work is supported by the ERC InvariantClass grant 320959. In memoriam Jean-Claude Risset, 1938-2016. ABSTRACT In the realm of machine listening, audio classification is the problem of automatically retrieving the source of a sound according to a pre- defined taxonomy. This dissertation addresses audio classification by designing signal representations which satisfy appropriate invariants while preserving inter-class variability. First, we study time-frequency scattering, a representation which extracts modulations at various scales and rates in a similar way to idealized models of spectrotempo- ral receptive fields in auditory neuroscience. We report state-of-the- art results in the classification of urban and environmental sounds, thus outperforming short-term audio descriptors and deep convolu- tional networks. Secondly, we introduce spiral scattering, a represen- tation which combines wavelet convolutions along time, along log- frequency, and across octaves, thus following the geometry of the Shepard pitch spiral which makes one full turn at every octave. We study voiced sounds as a nonstationary source-filter model where both the source and the filter are transposed in frequency through time, and show that spiral scattering disentangles and linearizes these transpositions. In practice, spiral scattering reaches state-of-the-art re- sults in musical instrument classification of solo recordings. Aside from audio classification, time-frequency scattering and spiral scat- tering can be used as summary statistics for audio texture synthe- sis. We find that, unlike the previously existing temporal scattering transform, time-frequency scattering is able to capture the coherence of spectrotemporal patterns, such as those arising in bioacoustics or speech, up to a scale of about 500 ms. Based on this analysis-synthesis framework, an artistic collaboration with composer Florian Hecker has led to the creation of five computer music pieces. v PUBLICATIONS 1. Lostanlen, V and S Mallat (2015). “Transformée en scattering sur la spirale temps-chroma-octave”. In: Actes du GRETSI. 2. Andén, J, V Lostanlen, and S Mallat (2015). “Joint Time-frequency Scattering for Audio Classification”. In: Proceedings of the IEEE Conference on Machine Learning for Signal Processing (MLSP). Re- ceived a Best Paper award. 3. Lostanlen, V and S Mallat (2015). “Wavelet Scattering on the Pitch Spiral”. In: Proceedings of the International Conference on Dig- ital Audio Effects (DAF-x). 4. Lostanlen, V and C Cella (2016). “Deep Convolutional Networks on the Shepard Pitch Spiral for Musical Instrument Recogni- tion”. In: Proceedings of the International Society on Music Informa- tion Retrieval (ISMIR). 5. Lostanlen, V, G Lafay, J Andén, and M Lagrange (2017). “Audi- tory Scene Similarity Retrieval and Classification with Relevance- based Quantization of Scattering Features”. To appear in IEEE/ACM Transactions on Audio, Speech, and Language Processing (TASLP), Special Issue on Sound Scene and Event Analysis. vii ACKNOWLEDGMENTS First and foremost, I thank Stéphane Mallat, my advisor, for being a day-to-day supporter of the “try it nonlinear and diagonal” method (Mallat, 2008, preface), which goes well beyond the realm of applied mathematics. For accepting to be part of my PhD committee, I thank Hervé Glotin, Mathieu Lagrange, Geoffroy Peeters, Gaël Richard, and Shi- hab Shamma. For fostering my interest in science from an early age, I thank Christophe Billy, Thomas Chénel, Bernard Gauthier, Sébastien Guf- froy, Raphaëla López, Étienne Mahé, Joaquin Martinez, and Anna Poveda. For teaching me the fundamentals of audio signal processing, I thank Roland Badeau, Bertrand David, Slim Essid, and Jérémie Jakubow- icz, who were my professors at TELECOM ParisTech. For supervising me as an intern in information geometry at Inria, and providing me a pleasant foretaste of scientific research, I thank Arshia Cont and Arnaud Dessein. For introducing me to the theory of the time-frequency scattering transform, and helping me putting it into practice, I thank Joakim Andén, who, more than a co-author, has been my mentor throughout these years of doctoral studies. I also thank Joan Bruna, who was the first in applying the scattering transform to audio texture synthesis, and was kind enough to explain his approach to me. I thank Carmine-Emanuele Cella who co-authored an ISMIR paper with me on deep convolutional networks on the pitch spiral; and Gré- goire Lafay, who contributed with me to a TASLP paper on relevance- based quantization of scattering features. I thank composer Florian Hecker for his relentless enthusiasm in using my software for computer music applications. I also thank Bob Sturm for putting us in contact. For their cordial invitations to give talks, I thank Lucian Alecu, Moreno Andreatta, Elaine Chew, Anne-Fleur Multon (from TrENS- missions student radio station), Ophélie Rouby, Simon Thomas (from Union PSL association), and Bruno Torrésani. For the small talk and the long-winded conversations, I thank my colleagues from École normale supérieure: Mathieu Andreux, Tomás Angles, Mickaël Arbel, Mia Xu Chen, Xiuyan Cheng, Ivan Dokmanic, Michael Eickenberg, Sira Ferradans, Matthew Hirn, Michel Kapoko, Bangalore Ravi Kiran, Chris Miller, Edouard Oyallon, Laurent Sifre, Paul Simon, Gilles Wainrib, Irène Waldspurger, Guy Wolf, Zhuoran Yang, Tomás Yany, and Sixin Zhang. ix I thank the PhD students from other labs who interacted with me: Thomas Andrillon, Pablo Arias, Victor Bisot, Stanislas Chambon, Ke- unwoo Choi, Léopold Crestel, Philippe Cuvillier, Pierre Donat-Bouillud, Simon Durand, Edgar Hemery, Clément Laroche, Simon Leglaive, Juan Ulloa, and Neil Zeghidour. I was invited to review the work of four interns: Randall Balestriero, Alice Cohen-Hadria, Christian El Hajj, and Florian Menguy. I wish them fair winds and following seas in their scientific careers. For their alacrity in solving computer-related problems, even when the problem lies between the chair and the keyboard, I thank Jacques Beigbeder and the rest of the SPI (which stands for “Service des Prodi- ges Informatiques”) at École normale supérieure. At the time of this writing, I am a postdoctoral researcher affili- ated to the Cornell Lab of Ornithology, working at the Center for Urban Science and Progress (CUSP) as well as the Music and Audio Research Lab (MARL) of New York University. I thank Juan Pablo Bello, Andrew Farnsworth, and Justin Salamon, for hiring me into this exciting position. More broadly, I thank Rachel Bittner, Mark Cartwright, Andrea Genovese, Eric Humphrey, Peter Li, Jong Wook Kim, Brian McFee, Charlie Mydlarz, and Claire Pelofi, for their welcome. I thank Iain Davies, Marshall Iliff, Heather Wolf, and the rest of the Information Department at the Cornell Lab for introducing me to the world of birdwatching and wildlife conservation. Finally, I thank my family and friends for their unfailing support. My most heartfelft thoughts go to my parents, Isabelle and Michel Lostanlen, and to my girlfriend, Claire Vernade. x CONTENTS 1introduction 1 1.1 Current challenges 3 1.1.1 Beyond short-term audio descriptors 3 1.1.2 Beyond weak-sense stationarity 4 1.1.3 Beyond local correlations in frequency 5 1.2 Contributions 6 1.2.1 Theoretical analysis of invariants 7 1.2.2 Qualitative appraisal of re-synthesized textures 7 1.2.3 Supervised classification 8 1.3 Outline 8 1.3.1 Time-frequency analysis of signal transforma- tions 8 1.3.2 Time-frequency scattering 8 1.3.3 Spiral scattering 9 2time-frequency analysis of signal transforma- tions
Details
-
File Typepdf
-
Upload Time-
-
Content LanguagesEnglish
-
Upload UserAnonymous/Not logged-in
-
File Pages199 Page
-
File Size-