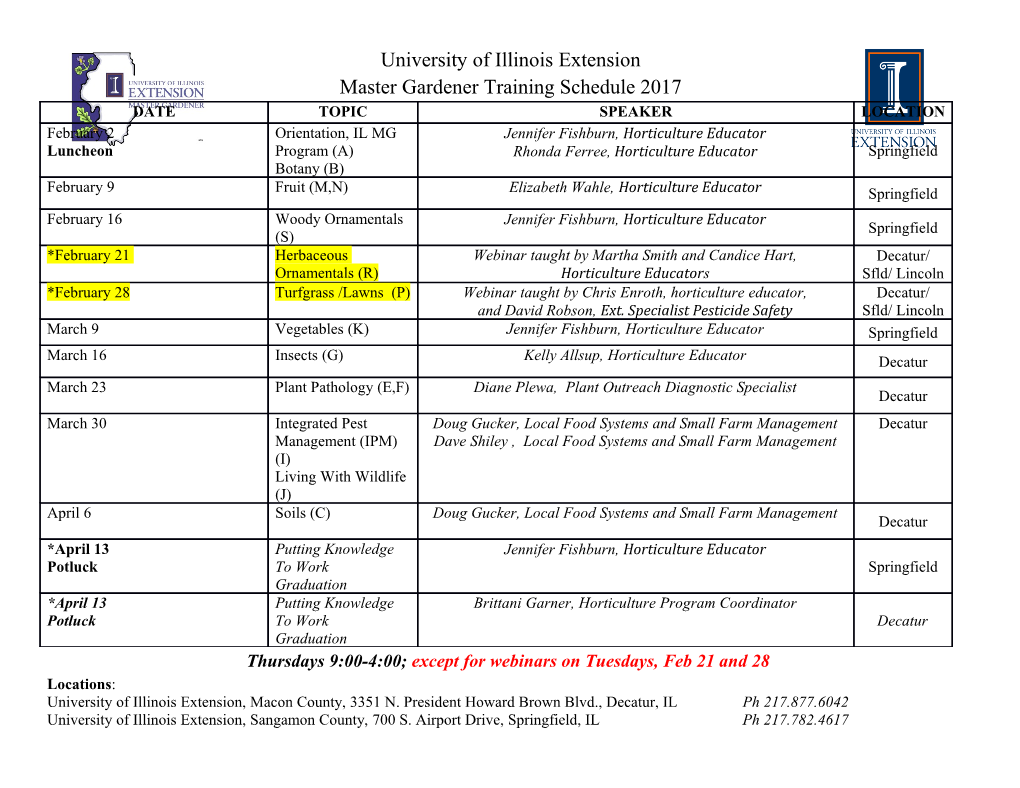
Harvard Data Science Review • Issue 2.4, Fall 2020 Post-Election Interview with Scott Tranter Scott Tranter, Liberty Vittert, Xiao-Li Meng Published on: Dec 21, 2020 License: Creative Commons Attribution 4.0 International License (CC-BY 4.0) Harvard Data Science Review • Issue 2.4, Fall 2020 Post-Election Interview with Scott Tranter 0:00 / 45:10 Listen to the interview and read the transcript below. Liberty Vittert: (LV): Hello and welcome to the Harvard Data Science Review's special theme on the 2020 U.S. Election. I'm Liberty Vittert, Media Feature Editor for the Harvard Data Science Review and I'm joined by my co-host Xiao-Li Meng, our Editor-in-Chief. Today we are speaking with Scott Tranter, the CEO and cofounder of 0ptimus Analytics, an adjunct professor at American University, and an adviser to Decision Desk HQ. Scott and his team predicted a Biden win in the Harvard Data Science Review in October 2020, and he is here today to discuss his correct prediction in the aftermath of this election. Xiao-Li Meng (XLM): Scott, it’s good to see you, and I'm particularly happy to see you very relaxed, after all of this. Scott Tranter (ST): Yeah. Can I just open up with the fact that my model was better than Liberty's off- the-cuff, whatever you wanted to say, prediction. LV: I think the audience must know. I feel like I need to come to terms with it. Scott and I made a bet about three years ago. I took Trump and he took the field as to who would win in 2020. And the deal was, that if I lost, I had to be Scott's donut truck chef for three months. And I lost, so I will be Scott's donut truck chef for three months. XLM: Liberty, you have to remember that Trump is still fighting for you. LV: So yeah, there's still hope! 2 Harvard Data Science Review • Issue 2.4, Fall 2020 Post-Election Interview with Scott Tranter XLM: Now I understand why he is still fighting. LV: Now you understand why, Xiao-Li, there could be election fraud! You never know. XLM: So, Scott, you still have to wait. ST: Yeah, I do. I have to wait ‘til it's all counted. I know, but I'm just I'm foreshadowing. LV: My favorite is that our producer was so excited about the fact I'm going to be a donut truck chef. It's the first time in all the episodes we've done that she is chimed in. ST: Oh, I look forward to discussing this on air. LV: Wait, we're already on air, Scott! Let's dive right in here to this. We have been operating on the premise that the pollsters did a much worse job in 2020 than they did in 2016, and you're saying that's incorrect, so I'd love to hear your thoughts on that. ST: I'm talking to people who do measurement for a business. So, how do you measure success of polling? How do you measure success of modeling if you follow all of us election forecasters on Twitter? This is a huge thing. We all argue about what's the right metric to measure and so we're trying to predict a political outcome. Most of the public views our models as a binary outcome: do we think Trump's going to win or Biden's going to win? They don't look at our model probabilities and factor that in. If we say, like our model did near the end, 80- 85% chance of a Biden win, everyone rounds that up to 100%, but that's not the case. Well, we understand that.One way to measure the efficacy of a model is, OK, we say 80- 85 percent, Biden's going to win. Biden wins. Therefore, great. Our model’s great. That's probably too simplistic for this group and certainly too simplistic given the complicatedness and the complexity of the model. 3 Harvard Data Science Review • Issue 2.4, Fall 2020 Post-Election Interview with Scott Tranter The other way to look at it is, OK, one of the outputs our model produces is how many electoral votes do we think the winner will get? Our model predicted 318 electoral votes for Joe Biden. Assuming Georgia goes the way we think it will, Joe Biden's going to end up with 306 electoral votes. I would say that's pretty close. The other way to measure it is you look at the individual state predictions. What was our prediction for Biden and Trump in North Carolina? What was our prediction in Iowa, and all that kind of stuff? So, all these different metrics go into this. The question is, what are the metrics that make a good model? Which is different from the polling, which I'm sure we'll talk about in a second. But that's kind of where the big argument is among—I shouldn't say “argument,” but discussion— around folks, like I know you got Allan Lichtman’s model, I know you got G. Elliot's and Gelman's. What is a good model, and how do you measure those types of things? I'd be curious, Xiao-Li, what's your statistical measure for a good model in this case? If you are a teacher, what grade would you give us? XLM: That's a great point. Let me answer the question by using a question. That's the strategy of answering a question. ST: Very Socratic of you. XLM Because, for me, you are absolutely right. The problem is, whatever you say, 85 or even 75— people not only round off to 100, they think that's a landslide winning. That's the way the public is going to translate these numbers. So, the way I think what you talk about—what's important to the metric—I think what's a problem to me, most importantly, is how do you convey to the general public about how they consume these kinds of numbers? Will they understand it properly, as you intended? instead of interpreting that is a landslide, then they get very disappointed when it is not. That's always about setting the public's expectation right. That's a very important one. So that's part of the conversation I want to have. My question for you is, really, I think there's a huge communication issue, By now, there is a general perception, no matter how we think about it, that people were saying the polls failed in 2016 and have failed again in 2020. Even, as you said, there’s a much more accurate way to measure those things and, of course, it depends on which poll you're talking about. And it's not just the poll results, it's how you analyze these results and coming up with your conclusion. I would love to get your opinions about how we convey those things properly so we don't get this misinformation out there. 4 Harvard Data Science Review • Issue 2.4, Fall 2020 Post-Election Interview with Scott Tranter ST: I think, first off, we require stats class in sixth grade, ninth grade, and to graduate into being a human being and a functioning adult in society. LV: You know your audience, Scott. ST: And obviously I know that's not realistic, but you're talking to someone who optionally took stats in high school and only took one stats class in undergrad, and then took a whole lot more afterwards. So, it's not like I opted into it most like the audience. I opted into stats because it's something that interests me and it's where it is. But it allowed me to look at these things differently. I think most of the public understands numbers because, by and large, we have a decent public education system, but —and it's not their fault—they don't want to put the time into what an 85 percent probability really means. Or, if you're Nate Silver back in 2016, the weekend before the election, you roughly said, ‘Hey, Donald Trump has a 30 percent chance of winning.’ Well, guess what, 30 percent happens one in three times. There are people go to Vegas every day and make bets with odds worse than that. They’ll put thousands of dollars on the roulette table and a single number with worse odds on that. I think that's what people need to realize on this. The other thing I'd like to separate a little bit is the difference forecast models, which is what we did for you guys at Harvard Data Science Review, and polls. And a poll is, in a way, a sort of a model. But the polls are a key ingredient that all of our forecast models use—or at least ours and the Economists use going forward. You know the old axiom: bad data in, bad data out. Polling error is something we get asked about a lot. So, let's define ‘polling error.’ Polling error is if in 2016, Donald Trump won a state by two points, and the polling average had Donald Trump winning the state by one point, then the polling error is considered to be one point. And that would be considered pretty good, given that a poll has a margin of error depending on sample size of two or three or four points, you would call that a good poll.
Details
-
File Typepdf
-
Upload Time-
-
Content LanguagesEnglish
-
Upload UserAnonymous/Not logged-in
-
File Pages21 Page
-
File Size-