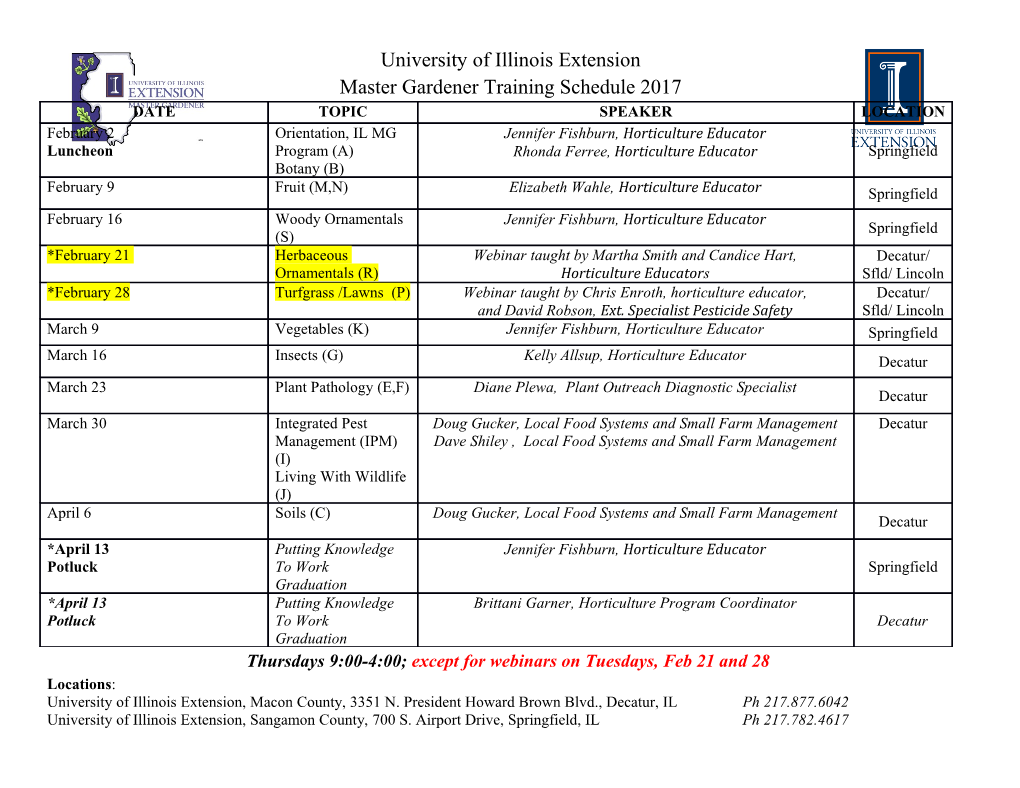
Locating Multiple Change-Points Using a Combination of Methods JOHAN ANDER SSON Master of Science Thesis Stockholm, Sweden 2014 Locating Multiple Change-Points Using a Combination of Methods JOHAN ANDERSSON Master’s Thesis in Mathematical Statistics (30 ECTS credits) Master Programme in Industrial Engineering and Management (120 credits) Royal Institute of Technology year 2014 Supervisor at KTH was Camilla Landén Examiner was Camilla Landén TRITA-MAT-E 2014:30 ISRN-KTH/MAT/E--14/30--SE Royal Institute of Technology School of Engineering Sciences KTH SCI SE-100 44 Stockholm, Sweden URL: www.kth.se/sci Abstract The aim of this study is to find a method that is able to locate multiple change-points in a time series with unknown properties. The methods that are investigated are the CUSUM and CUSUM of squares test, the CUSUM test with OLS residuals, the Mann-Whitney test and Quandt’s log likelihood ratio. Since all methods are detecting single change-points, the binary segmentation technique is used to find multiple change-points. The study shows that the CUSUM test with OLS residuals, Mann- Whitney test and Quandt’s log likelihood ratio work well on most samples while the CUSUM and CUSUM of squares are not able to detect the location of the change-points. Furthermore the study shows that the binary segmentation technique works well with all methods and is able to detect multiple change-points in most circumstances. The study also shows that the results can, most of the time, be improved by using a combination of the methods. i ii Sammanfattning Syftet med studien är att hitta en metod som identifierar tidpunkterna för strukturella brott i en tidsserie med okända egenskaper. De metoder som undersöks är CUSUM och CUSUM av kvadrater, CUSUM test med OLS-residualer, Mann-Whitney-test samt Quandts log likelihood ratio. Eftersom alla metoder identifierar enbart en brytpunkt används binära uppdelningstekniken för att hitta multipla brytpunkter. Studien visar att CUSUM-test med OLS-residualer, Mann-Whitney-test och Quandt’s log likelihood ratio fungerar bra för de flesta stickproven medan CUSUM och CUSUM av kvadrater inte hittar tidpunkten för brytpunkterna. Vidare så visar studien att binära uppdelningstekniken fungerar bra med alla metoder och kan identifiera multipla brytpunkter i de flesta fallen. Studien visar också att resultaten för det mesta kan förbättras genom att använda en kombination av metoderna. iii iv Acknowledgements I would like to thank my supervisors, Camilla Landén at KTH and Jovan Zamac at Handelsbanken. Camilla, thank you for your support and advice throughout the process. Jovan, thank you for your suggestions, feedback and constant support. I would also like to thank Erik Svensson at Handelsbanken. Thank you for your support. v vi Contents 1 Introduction ..................................................................................................................................... 1 1.1 Background .............................................................................................................................. 1 1.2 Purpose .................................................................................................................................... 2 1.3 Outline ..................................................................................................................................... 2 2 Literature ......................................................................................................................................... 3 2.1 The change-point problem ...................................................................................................... 3 2.2 Change-point detection methods ........................................................................................... 4 3 Methodology ................................................................................................................................... 7 3.1 Binary segmentation technique .............................................................................................. 7 3.2 CUSUM and CUSUMSQ............................................................................................................ 8 3.3 OLSCUSUM ............................................................................................................................ 10 3.4 Quandt’s log likelihood ratio ................................................................................................. 11 3.5 Mann-Whitney ...................................................................................................................... 12 3.6 Ensemble method ................................................................................................................. 13 3.7 Combined method ................................................................................................................. 13 3.8 Data ....................................................................................................................................... 14 3.8.1 Real life data .................................................................................................................. 14 3.8.2 Generated data.............................................................................................................. 14 3.9 Evaluation of the methods .................................................................................................... 15 4 Results ........................................................................................................................................... 16 4.1 The distributions of the residuals .......................................................................................... 16 4.2 Evaluating the individual methods ........................................................................................ 18 4.2.1 Normal distribution ....................................................................................................... 18 4.2.2 Student’s t-distribution ................................................................................................. 26 4.2.3 Cauchy distribution........................................................................................................ 27 4.2.4 Uniform distribution ...................................................................................................... 29 4.2.5 AR(1)-process ................................................................................................................ 30 4.3 Evaluation of the combined method ..................................................................................... 32 4.3.1 Normal distribution ....................................................................................................... 32 4.3.2 Cauchy distribution........................................................................................................ 33 4.3.3 AR(1)-process ................................................................................................................ 35 4.4 Methods applied to real data ................................................................................................ 38 5 Discussion ...................................................................................................................................... 41 vii 5.1 Binary segmentation ............................................................................................................. 41 5.2 CUSUM and CUSUMSQ.......................................................................................................... 41 5.3 OLSCUSUM ............................................................................................................................ 42 5.4 Quandt’s log likelihood ratio ................................................................................................. 42 5.5 Mann-Whitney ...................................................................................................................... 43 5.6 Ensemble method ................................................................................................................. 43 5.7 Combined method ................................................................................................................. 43 5.8 Methods applied to real life data .......................................................................................... 44 6 Conclusions .................................................................................................................................... 46 6.1 Suggestions for further research ........................................................................................... 46 Bibliography ........................................................................................................................................... 48 Appendix A – Probabilities of overlapping subsamples ........................................................................ 49 Appendix B – Autocorrelation plots for real life data ........................................................................... 52 viii List of figures Figure 1 – Illustration of the binary segmentation technique ................................................................ 7 Figure 2 – A time series with a break and the statistic with upper- and lower confidence bounds ..................................................................................................................................................... 9 Figure 3 – A time series with a break and the statistic
Details
-
File Typepdf
-
Upload Time-
-
Content LanguagesEnglish
-
Upload UserAnonymous/Not logged-in
-
File Pages70 Page
-
File Size-