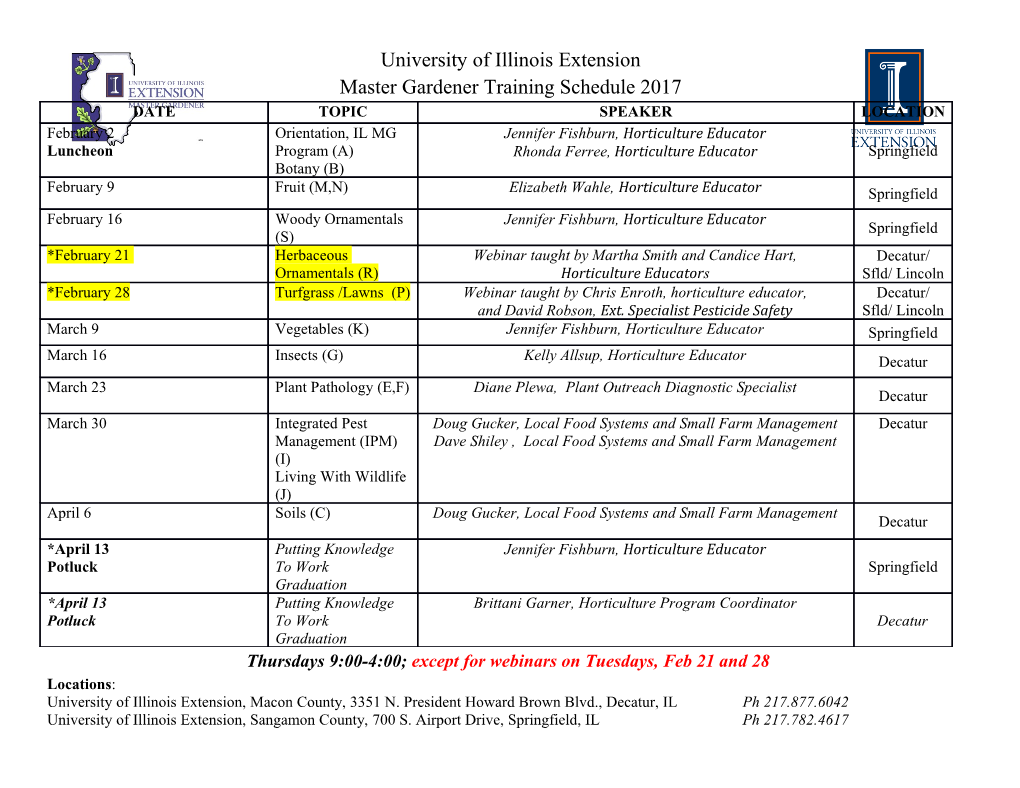
City University of New York (CUNY) CUNY Academic Works All Dissertations, Theses, and Capstone Projects Dissertations, Theses, and Capstone Projects 2-2019 The Ability of Polynomial and Piecewise Regression Models to Fit Polynomial, Piecewise, and Hybrid Functional Forms A. Zapata The Graduate Center, City University of New York How does access to this work benefit ou?y Let us know! More information about this work at: https://academicworks.cuny.edu/gc_etds/3048 Discover additional works at: https://academicworks.cuny.edu This work is made publicly available by the City University of New York (CUNY). Contact: [email protected] THE ABILITY OF POLYNOMIAL AND PIECEWISE REGRESSION MODELS TO FIT POLYNOMIAL, PIECEWISE, AND HYBRID FUNCTIONAL FORMS by A. ZAPATA A dissertation submitted to the Graduate Faculty in Educational Psychology in partial fulfillment of the requirements for the degree of Doctor of Philosophy, The City University of New York 2019 ii © 2019 A. ZAPATA All Rights Reserved iii The Ability of Polynomial and Piecewise Regression Models to Fit Polynomial, Piecewise, and Hybrid Functional Forms by A. Zapata This manuscript has been read and accepted for the Graduate Faculty in Educational Psychology in satisfaction of the dissertation requirement for the degree of Doctor of Philosophy. Date Keith Markus, Ph.D. Chair of Examining Committee Date Bruce Homer, Ph.D. Executive Officer Supervisory Committee: Sophia Catsambis, Ph.D. Keith Markus, Ph.D. David Rindskopf, Ph.D. THE CITY UNIVERSITY OF NEW YORK iv ABSTRACT The Ability of Polynomial and Piecewise Regression Models to Fit Polynomial, Piecewise, and Hybrid Functional Forms by A. Zapata Advisor: Keith Markus, Ph.D. The present simulation study evaluated and compared the performance of piecewise regression to one of the more recognizable methods utilized to model nonlinear relationships within the confines of OLS, polynomial regression. This investigation examined (a) the comparative performance of piecewise and polynomial regression under various experimental conditions (e.g., fitting datasets with different underlying structure, different sample sizes, and varying number slope change-points), and (b) their utility and ease for empirically identifying the point(s) where a regression line changes trajectory. The research design, which followed a mixed factorial design, included four independent variables: sample size, underlying data- generating model type, type of regression analyses (i.e., polynomial, piecewise with known knots, piecewise with unknown knots) and number of significant change-points. Seven outcome variables were used to evaluate and compare model performance: the square root of the mean squared error (RMSE), RMSE omitting the 10% most extreme residuals, RMSE for only the 10% omitted, R-squared values, estimated change-point location(s), estimated Y-hat when x was at the change-point, and the empirical standard error. Findings indicated that under conditions of v misspecification, polynomial and piecewise were incapable of correctly estimating the location of the true change-points and the Y-hats when x was at the estimated change-point locations. Misspecified models, in general, had inferior RMSE and R2 outcomes than their correctly specified counterparts. Sample size was a good discriminatory element, as larger ones helped better expose a misspecified model fit. Keywords: Piecewise regression, polynomial regression, segmented regression, nonlinear, knots, change-point, spline linear regression vi TABLE OF CONTENTS List of Tables .................................................................................................................................. x List of Figures ............................................................................................................................... xii CHAPTER 1: INTRODUCTION & LITERATURE REVIEW ..................................................... 1 Introduction ..................................................................................................................................... 1 Study objective and rationale .............................................................................................. 3 Governing research question............................................................................................... 4 Summary of chapters .......................................................................................................... 4 Review of the Literature ................................................................................................................. 5 Familiarity of multiple linear regression............................................................................. 5 Multiple regression’s presence in doctoral training ................................................... 6 Gravitating towards what we know ........................................................................... 8 Building on researchers’ linear regression knowledgebase ....................................... 9 Characterizing nonlinear relationships in multiple linear regression ............................... 10 Polynomial regression ....................................................................................................... 11 Basic structure of polynomial regression ................................................................. 11 Advantages of polynomial regression ...................................................................... 13 Critique of polynomial regression: The multicollinearity problem ......................... 13 Other critiques of polynomial regression ................................................................. 15 Polynomial regression, a global model .................................................................... 15 Exploring an alternative to polynomial regression ........................................................... 16 Piecewise Regression ........................................................................................................ 17 Piecewise regression and the family of splines ....................................................... 18 vii Piecewise regression: A regression technique by any other name .......................... 18 Basic structure of piecewise regression ................................................................... 20 The importance of line continuity ............................................................................ 23 Advantages of piecewise regression ........................................................................ 24 Limitations of piecewise regression ........................................................................ 25 Importance and timeliness for detecting change points .................................................... 26 Estimating location of critical change-points .......................................................... 27 Piecewise and polynomial regression in education research ............................................ 31 Polynomial regression in published education studies ............................................ 32 Piecewise regression in published education studies ............................................... 32 Making sense of the piecewise-polynomial literature search .................................. 33 Studies comparing piecewise and polynomial regression ................................................ 34 Chapter summary .............................................................................................................. 37 CHAPTER 2: METHOD .............................................................................................................. 38 Study overview ................................................................................................................. 38 Research questions ............................................................................................................ 38 Study design and overview of procedure .......................................................................... 40 Functions for generating simulated data and the replication process ............................... 41 Parameter values for the simulation functions ......................................................... 43 Determining piecewise parameters from polynomial parameters ........................... 44 Parameter values specified in the simulation functions ........................................... 45 Regression analyses: Models fitted to datasets ................................................................. 48 Evaluation criteria: Comparing the fitted models ............................................................. 50 viii CHAPTER 3: RESULTS .............................................................................................................. 51 Part I: Analysis of research questions focusing on model fit........................................................ 51 Q1 and Q2 Results ............................................................................................................ 52 Q3 Results ......................................................................................................................... 55 RMSE results: Fits to matching and opposing data structure .................................. 56 RMSE-trimmed and extreme: Fits to matching and opposing data structures ........ 60 R2 results: Fits to matching and opposing data structures ....................................... 60 Fits to data with a hybrid structure .........................................................................
Details
-
File Typepdf
-
Upload Time-
-
Content LanguagesEnglish
-
Upload UserAnonymous/Not logged-in
-
File Pages175 Page
-
File Size-