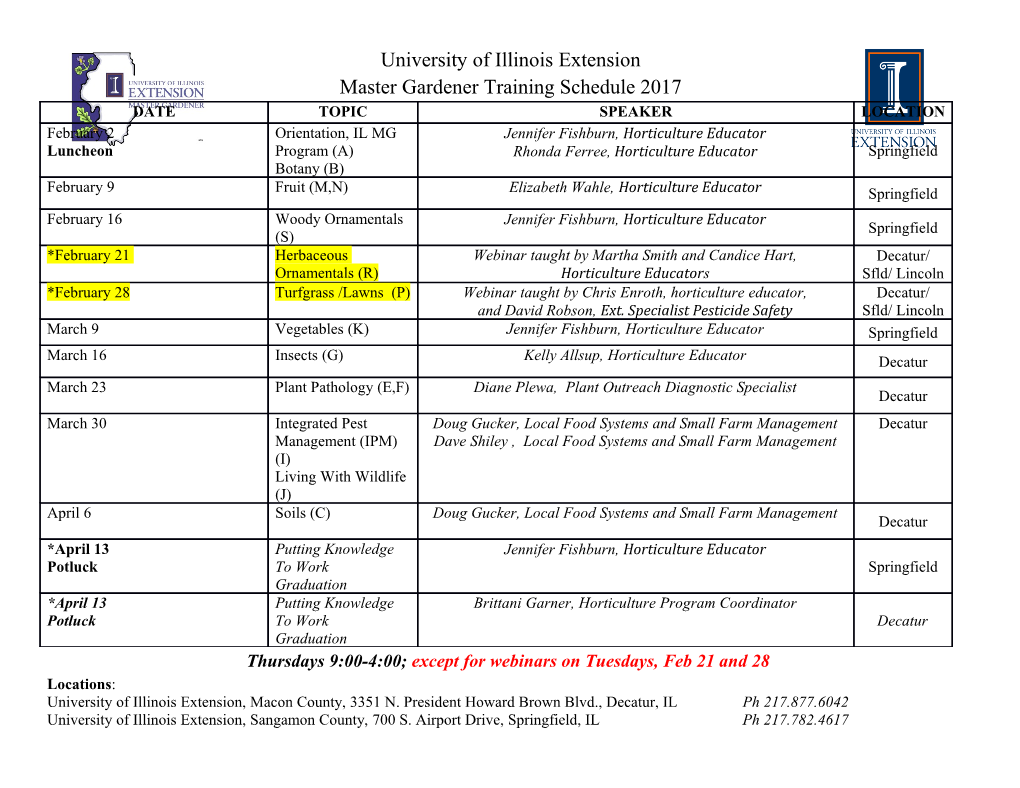
An Investigation of an Evolutionary Approach to the Opening of Go Graham Kendall, Razali Yaakob Philip Hingston School of Computer Science and IT School of Computer and Information Science University of Nottingham, UK Edith Cowan University, WA, Australia {gxk|rby}@cs.nott.ac.uk [email protected] Abstract – The game of Go can be divided into three stages; even told how it had performed in each individual game. the opening, the middle, and the end game. In this paper, The conclusion of Fogel and Chellapilla is that, without evolutionary neural networks, evolved via an evolutionary any expert knowledge, a program can learn to play a game strategy, are used to develop opening game playing strategies at an expert level using a co-evolutionary approach. for the game. Go is typically played on one of three different board sizes, i.e., 9x9, 13x13 and 19x19. A 19x19 board is the Additional information about this work can be found in standard size for tournament play but 9x9 and 13x13 boards [19, 20, 21, 22]. are usually used by less-experienced players or for faster Go is considered more complex than chess or checkers. games. This paper focuses on the opening, using a 13x13 One measure of this complexity is the number of positions board. A feed forward neural network player is played against that can be reached from the starting position which Bouzy a static player (Gondo), for the first 30 moves. Then Gondo and Cazenave estimate to be 10160 [23]. Chess and takes the part of both players to play out the remainder of the checkers are about 1050 and 1017, respectively [23]. game. Two experiments are presented which indicate that The size of the search space is the primary reason why a learning is taking place. good automated Go player is difficult to produce. To reduce the search space, the board can be scaled down I. INTRODUCTION either by reducing the board size or by dividing the board Go is an ancient game, which originated in China at into sub-boards (decomposition). These techniques can least 2000 years ago and was known originally as Weiqi reduce the computational effort required for playing the [1]. The name Go came from the shortened Japanese word game, which is one of the main constraints in producing an Igo. Automated Go programs began appearing in the 1970s effective automated Go player. The early work for small [2] and 1980s [3, 4, 5, 6]. Since that time, Go has been the boards can be found in [24, 25] and decomposition subject of research areas such as machine learning [7, 8, approaches are reported in [26]. 9], cognitive science [10], and evolutionary computing The game of Go can be divided into three general [11, 12], but automated Go programs are still far from the phases: the opening game, the middle game, and the standard of human experts [13]. endgame [27]. Researchers have applied a variety of The games of Go, chess, and checkers are similar, in techniques to the middle game, which is considered the that they are finite, perfect information games, played most difficult – yet important – phase [27]. However, between two players. One of the best-known chess Ishigure [28] states that the opening game is the most programs, Deep Blue [14], achieved world champion important phase. In order to make territory, the best place status when, in 1997, it beat Garry Kasparov by a score of to move is in a corner and the least valuable moves are 3.5-2.5. Utilising custom-built hardware [15], Deep Blue those in the centre of the board. The number of plays in the executed sophisticated search algorithms to analyse up to opening is not predetermined [28]. Aggressive player may 200 million positions per second. start to attack the opponent (thus entering the middle Chinook, a checkers program, developed by Jonathan game) just a few turns after the start of the game, whilst Schaeffer’s team at The University of Alberta won the other openings may last longer. world checkers title in 1994 [16, 17]. Chinook used an Playing just the opening is the approach we adopt here. opening, and endgame database and an extensive checkers In this paper, we are not attempting to reduce the size of knowledge base. the search space or incorporate any Go knowledge. The Chellapilla and Fogel [18], also considered checkers, objective of this research is to investigate a population of developing Blondie24 - a checkers player, which learned evolutionary neural networks, which play the opening of its strategy using a co-evolutionary approach. The current Go and compete to survive to the next generation. A board position in Blondie24 is evaluated using an artificial feedforward neural network player is played against a neural network with the weights of the network being static player, Gondo (written by Jeffrey Greenberg [29]). evolved via an evolutionary strategy. The output of the We play against Gondo for the first 30 turns (15 turns for network is used in a minimax search. Blondie24 was not each player). At that time Gondo takes the part of both provided with any domain knowledge. It received points players and plays out the remainder of the game to based on whether it won, lost, or drew games and was not determine the outcome. An evolutionary strategy is used to Differences in playing strength can be balanced by a evolve the networks. We use a 13x13 board. handicap system, which allows the Black player to place Previous authors have investigated evolving neural several stones on the board before the start of the game. networks to play Go. Richard et al. evolved neural Go is played between two players, Black and White. networks using the SANE1 (Symbiotic, Adaptive Neuro- The game starts with an empty board. Stones are placed Evolution [30]) method [27]. Board sizes of 5x5, 7x7, and on any empty intersection on the board with players 9x9 were used in evolving networks to play on small making alternate moves. Black plays first. Go players can boards against a simple computer opponent called Wally2. pass their turn at any time but consecutive passes ends the Each intersection on the board has two input units and one game. output unit. Any positive output indicates a good move, the A stone on an intersection has a set of liberties (empty larger the value, the better the move. If none of its outputs intersections next to a stone). Figure 1a shows a white is positive, the network will pass its turn. SANE required stone with four liberties. When the opponent places a stone 20 generations to defeat Wally on a 5x5 board, 50 on any neighbouring intersection, white will lose one generations on a 7x7 board, and on a 9x9 board, 260 liberty. In Figure 1b, black stones surround the white generations were needed. Donelly et al. also evolved stone, which means white has no liberties and is captured neural networks via self-play [31]. and removed from the board (Figure 1c). This territory Other researchers have used neural networks to learn to now belongs to black. play Go using machine learning methods such as supervised learning (training on moves from professional games) [32] and reinforcement learning [33, 34]. Yen et al. took a different approach to learning to play Go openings with Jimmy 4.0, which has been ranked 4 3 kyu when they adopted 100 questions from Go books to test the rank of their opening game [35]. Jimmy plays the (a) (b) (c) opening stage by trying to occupy the corners (called joseki - a series of standard moves in corners or sometimes Figure 1: (a) White stone has 4 liberties, (b) Black stones are on edges), extending the edges, and dealing with Moyo surrounding a white stone, (c) White stone has been removed and the black player gets 1 point of territory. (large potential territories). Pattern matching techniques are used to occupy the corners and generate the Moyo moves. In extending the edges, two techniques are used. Placing a stone that does not make a capture, and has no Pattern matching generates moves near the existing stones liberties, is illegal. For example, the white player cannot on the board. A “split” method places a stone equidistant place a stone in the centre of black territory in Figure 1c. between two stones, which are near opposite corners. Placing a stone that results in a repeating position seen For an excellent review of the state of the art in after the same player’s previous play is also illegal. This is computer Go programming, we recommend Müller [36] called the ko-rule. and as a starting point for further reading, Enzenberger’s Figure 2a shows a marked white stone that has only one online bibliography [37]. Other reviews focussing on AI liberty. Black captures the marked white stone by playing approaches may be found in [38, 39]. at position k. After the marked white stone has been removed from the board, the white player cannot place a II. RULES OF GO stone on r, Figure 2b, before the board position has changed (i.e. white must play on another intersection The aim of Go is to gain as much territory as possible. before being able to place a stone on r). Players will try to capture an opponent’s stones and also define areas as their own territory. At the end of the game, the winner will be identified by counting territories using one of two scoring methods; territory scoring or area scoring. Japanese rules use territory scoring. Surrounded k r territory is counted, plus the number of captured opponent stones.
Details
-
File Typepdf
-
Upload Time-
-
Content LanguagesEnglish
-
Upload UserAnonymous/Not logged-in
-
File Pages8 Page
-
File Size-