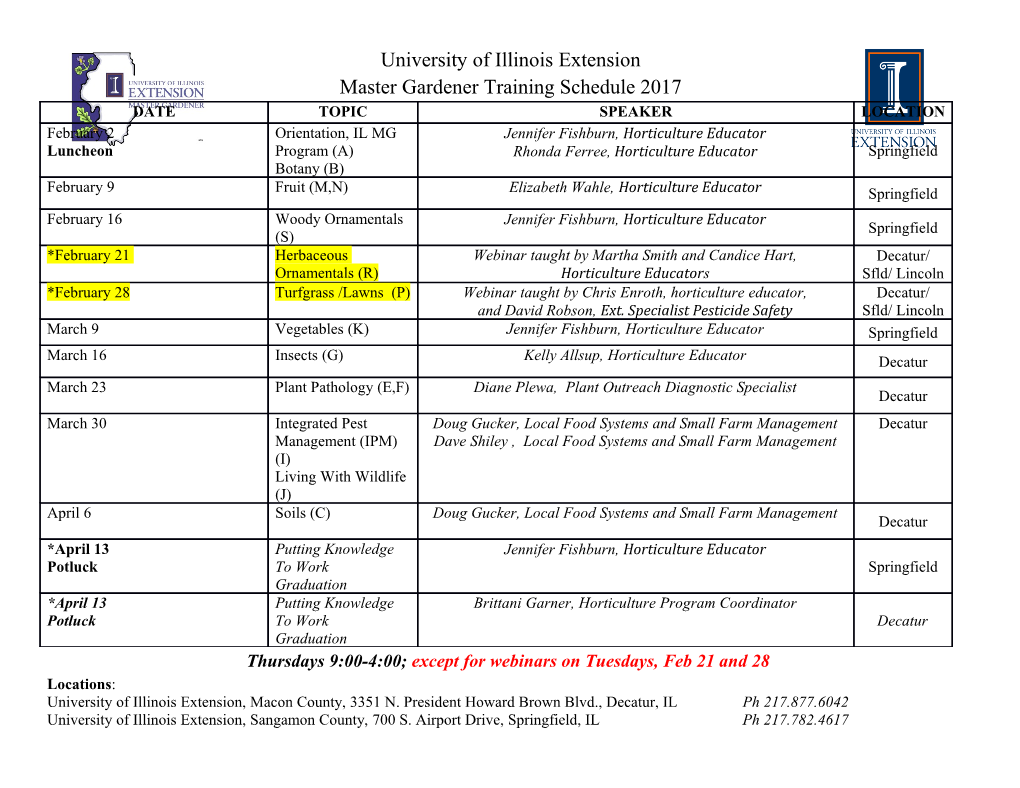
Technische Universität München Lehrstuhl für Finanzmathematik Incorporating parameter risk into derivatives prices – bid-ask pricing and calibration Karl Friedrich Bannör Vollständiger Abdruck der von der Fakultät für Mathematik der Technischen Universität München zur Erlangung des akademischen Grades eines Doktors der Naturwissenschaften (Dr. rer. nat.) genehmigten Dissertation. Vorsitzende: Univ.-Prof. Claudia Czado, Ph.D. Prüfer der Dissertation: 1. Univ.-Prof. Dr. Matthias Scherer 2. Univ.-Prof. Dr. Ralf Korn, Technische Universität Kaiserslautern 3. Lecturer Dr. Christoph Reisinger, University of Oxford/Vereinigtes Königreich Die Dissertation wurde am 16. Mai 2013 bei der Technischen Universität München eingereicht und durch die Fakultät für Mathematik am 26. Juli 2013 angenommen. To my beloved, all-time supporting wife Bianka, who made this work possible. Abstract In this thesis, a new methodology using convex risk measures is developed to incorporate parameter risk into prices of financial derivatives, provided that a distribution on the parameter space is given. In this context, weak continuity properties of convex risk measures w.r.t. the underlying probability measure on the parameter space are analyzed. Parameter risk arising from time series estimation is discussed in extensive numerical case studies and large-sample approximations for certain parameter risk-captured prices are stated. A technique to induce a parameter distribution in case of calibration to market prices is presented, allowing to conduct a comparison of parameter risk in different financial market models and of different exotic options. For the calibration to quoted bid-ask prices, a non-parametric calibration approach to broad classes of distortion risk measures is developed and a calibration to quoted bid-ask prices – comparing the non- parametric approach to given parametric suggestions – is assessed. Zusammenfassung In dieser Doktorarbeit wird eine neue Methodik basierend auf konvexen Risikomaßen entwickelt, um Parameterrisiko in den Preisen von Finanzderivaten zu berücksichtigen. In diesem Kontext werden schwache Stetigkeitseigenschaften von konvexen Risikomaßen als Funktion des unterliegenden Wahrscheinlichkeitsmaßes auf dem Parameterraum un- tersucht. Es wird Parameterrisiko resultierend aus Zeitreihenschätzung in numerischen Fallstudien untersucht und Approximationen für parameterrisikoadjustierte Preise im Falle von großen Stichproben angegeben. Im Falle der Parameterschätzung durch Kalib- rierung an Marktpreise wird eine Technik entwickelt, ein Wahrscheinlichkeitsmaß auf dem Parameterraum zu konstruieren, um einen Vergleich des Parameterrisikos in verschiede- nen Finanzmarktmodellen sowie verschiedenen exotischen Optionen durchzuführen. Zur Kalibrierung an marktquotierte Bid-Ask-Preise wird ein nichtparametrischer Ansatz zur Kalibrierung an Verzerrungsrisikomaße entwickelt und eine Kalibrierung an mark- tquotierte Bid-Ask-Preise zum Vergleich mit existierenden parametrischen Methoden durchgeführt. 5 Acknowledgements At first, I want to thank my supervisor Prof. Dr. Matthias Scherer for his continuous support during all the time. He always had an open door for questions and fruitful discussions about the thesis, came up with useful ideas, and helped me with his kind and patient nature to make my step into the research community. I think that this thesis would not have been possible without him. I also want to thank Prof. Dr. Rudi Zagst for providing a possibility to finance my PhD studies with work at the Chair of Mathematical Finance as well as giving me the opportunity to present my research to a broader audience at various international conferences. Furthermore, I want to thank Prof. Dr. Rüdiger Kiesel for inviting me to Essen for a joint research project, which allowed me to gain further insight into electricity markets. During the time of my PhD studies, I could always stock up my creativity and exchange ideas with my appreciated colleagues, among them I found wonderful friends. So, I thank my roommates Daniela Selch and Thorsten Schulz for bearing me as their officemates, Lexuri Fernández and Peter Hieber for nice distraction during creative breaks, German Bernhart, Maximilian Gass, Dr. Asma Khedher, Mirco Mahlstedt, Franz Ramsauer, and Steffen Schenk for great table football matches, PD Dr. Aleksey Min for telling me great stories, and Tim Friederich, Prof. Dr. Kathrin Glau, Julia-Stefanie Kraus, Mikhail Krayzler, Maximilian Mair, Daniela Neykova, and Natalia Shenkman for a good time together at the Chair of Mathematical Finance. Since they have provided an excellent environment for building the foundation of my education during my childhood, I want to thank my parents Monika and Karl Heinz Hofmann. Last, but not least, I want to thank my dearly-loved wife Bianka Bannör: Due to all her support in my private life and her sacrifice, I was able to write this thesis. 6 Contents 1 Introduction 9 1.1 The principles of mathematical modeling in finance . ........ 9 1.2 Model and parameter risk and uncertainty . ..... 11 1.3 Literature overview . 15 1.4 Contributions .................................. 18 2 Mathematical preliminaries 23 2.1 Theory of convex risk measures . 23 2.2 Choquet integration theory . 29 3 Model and parameter risk – a convex risk measure ansatz 37 3.1 Model and parameter uncertainty in derivatives pricing ........... 37 3.2 Capturing model and parameter risk by using convex risk measures . 43 3.3 Examples: AVaR- and entropic-driven risk-captured prices . 48 3.4 Market-implied distributions . ..... 52 4 Convergencepropertiesofrisk-capturedprices 55 4.1 Convergence results of risk-capturing functionals . ........... 55 4.2 Counterexamples ................................ 60 4.3 Outlook ..................................... 60 5 Application: Bid-askpricesimpliedbyestimationrisk 63 5.1 Estimation risk-captured prices for consistent estimators .......... 63 5.2 Asymptotics of risk-capturing functionals . ........ 65 5.3 Case study: Estimation risk for Margrabe options in a two-dimensional Black-Scholes market . 68 5.4 The parameter risk-captured valuation of a gas power plant ........ 74 6 Application: Parameter risk induced by calibration to market prices 89 6.1 Calibration to market prices . 91 7 Contents 6.2 Parameter risk from calibration to market prices . ........ 92 6.3 Case study: Comparing parameter risk of different models and exotic options 99 6.3.1 Parameter risk in different models . 104 6.3.2 Parameter risk profiles of different exotics . 112 6.4 Outlook .....................................113 7 Calibration of risk-captured model prices to bid-ask marketprices 119 7.1 Introduction...................................119 7.2 The bid-ask calibration problem . 120 7.3 A non-parametric calibration scheme for bid-ask price . ..........125 7.3.1 Generalresults .............................125 7.3.2 Application to parameter risk-captured prices . .......134 7.4 Applicationtodata............................... 134 7.5 Calibration to discontinuous distortion functions . ...........137 7.6 Outlook .....................................144 8 Conclusion 149 8.1 Summary ....................................149 8.2 Critical reflection . 151 8.3 Outlook .....................................152 Bibliography 155 8 1 Introduction In this introductory chapter, we first give a short insight into mathematical modeling in finance and explain the cruciality of model and parameter risk and uncertainty in mathematical finance. We provide a literature overview on existing works on model and parameter risk and uncertainty in mathematical finance and briefly summarize the main contributions of this thesis. 1.1 The principles of mathematical modeling in finance Today, mathematical modeling plays an important role in many different areas like, e.g., geoscience, engineering, empirical social science, and, last but not least, finance. In physics and engineering, mathematical modeling of real-world phenomena goes back to, e.g., Sir Isaac Newton and even to the ancient Greeks. Contrasting, in finance, mathematical and particularly stochastic modeling is a rather recent trend, starting around 1900 with Louis Bachelier’s seminal PhD thesis (cf. (Bachelier, 1900)). When regarding the financial world instead of modeling phenomena from classical me- chanics (like, e.g., in engineering), one immediately recognizes that the whole system is much more complex in the sense that many different forces drive the market in many different ways. When describing the fall of a stone to the ground in a laboratory, there are undoubtedly many different forces apart from earth gravitation that actually have an influence (e.g. the aerodynamic resistance, the gravitation of different objects in the labo- ratory). But their magnitude is so small compared to the magnitude of earth gravitation that not considering them eventually does not matter too much for the model. Contrasting, when modeling financial markets (e.g. stock markets for the purpose of derivatives pricing), there are many different market participants that actually influence asset prices in many different ways. Hence, a model trying to capture the whole market microstructure with all action and interaction of market participants would be extremely complicated, modeling many different dimensions with myriads of parameter. Thus, 9 such an approach is challenging from a modeling and computational perspective. But, contrastingly, there are several other good reasons not to model the microstructure of financial markets. First, financial markets
Details
-
File Typepdf
-
Upload Time-
-
Content LanguagesEnglish
-
Upload UserAnonymous/Not logged-in
-
File Pages165 Page
-
File Size-