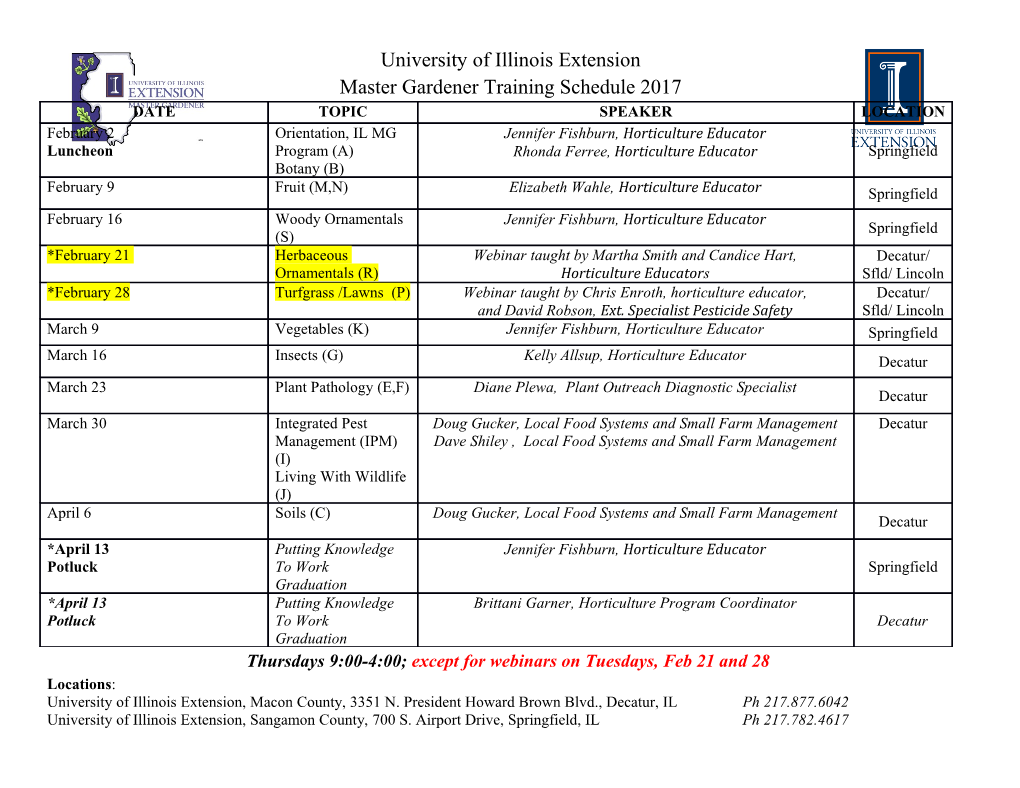
PRINCIPLES OF CIRCUIT SIMULAITON LectureLecture 9.9. LinearLinear Solver:Solver: LULU SolverSolver andand SparseSparse MatrixMatrix Guoyong Shi, PhD [email protected] School of Microelectronics Shanghai Jiao Tong University Fall 2010 2010-11-15 Slide 1 OutlineOutline Part 1: • Gaussian Elimination • LU Factorization • Pivoting • Doolittle method and Crout’s Method • Summary Part 2: Sparse Matrix 2010-11-15 Lecture 9 slide 2 MotivationMotivation • Either in Sparse Tableau Analysis (STA) or in Modified Nodal Analysis (MNA), we have to solve linear system of equations: Ax = b ⎛⎞A 00⎛⎞i ⎛0 ⎞ ⎜⎟ 00IAv−=T ⎜⎟ ⎜ ⎟ ⎜⎟⎜⎟ ⎜ ⎟ ⎜⎟⎜⎟ ⎜ ⎟ ⎡⎤11 1 KKiv0 ⎝⎠ e ⎝ S ⎠ GG ⎝⎠⎢⎥++22 −− RR13 R 3⎛⎞e1 ⎛⎞0 ⎢⎥⎜⎟= ⎜⎟ ⎢⎥111⎝⎠e2 ⎝⎠I S 5 ⎢⎥−+ ⎣ RRR343⎦ 2010-11-15 Lecture 9 slide 3 MotivationMotivation • Even in nonlinear circuit analysis, after "linearization", again one has to solve a system of linear equations: Ax = b. • Many other engineering problems require solving a system of linear equations. • Typically, matrix size is of 1000s to millions. • This needs to be solved 1000 to million times for one simulation cycle. • That's why we'd like to have very efficient linear solvers! 2010-11-15 Lecture 9 slide 4 ProblemProblem DescriptionDescription Problem: Solve Ax = b A: nxn (real, non-singular), x: nx1, b: nx1 Methods: – Direct Methods (this lecture) Gaussian Elimination, LU Decomposition, Crout – Indirect, Iterative Methods (another lecture) Gauss-Jacobi, Gauss-Seidel, Successive Over Relaxation (SOR), Krylov 2010-11-15 Lecture 9 slide 5 GaussianGaussian EliminationElimination ---- ExampleExample ⎧25xy+= ⎨ ⎩xy+=24 1 ⎛215⎞ − ⎛215⎞ ⎧x = 2 2 ⎜ 33⎟ ⎨ ⎜ 124⎟ ⎜0 ⎟ ⎩y = 1 ⎝ ⎠ ⎝ 22⎠ ⎛⎞⎛⎞10 21 ⎛⎞21 ⎜⎟⎜⎟ ⎜⎟= 13LU factorization ⎝⎠12 ⎜⎟⎜⎟10 ⎝⎠⎝⎠22 2010-11-15 Lecture 9 slide 6 UseUse ofof LULU FactorizationFactorization L U ⎛⎞⎛⎞10 21 ⎛21 ⎞⎛⎞xx⎜⎟⎜⎟⎛⎞ ⎛5⎞ ⎜ ⎟⎜⎟==13⎜⎟ ⎜⎟ ⎝12 ⎠⎝⎠yy⎜⎟⎜⎟10 ⎝⎠ ⎝4⎠ ⎝⎠⎝⎠22 Define ⎛⎞21 ⎛⎞ux⎜⎟ ⎛⎞ Solving the L-system: ⎜⎟:= 3 ⎜⎟ ⎝⎠vy⎜⎟0 ⎝⎠ ⎝⎠2 ⎛⎞10 ⎛⎞5 ⎛⎞u ⎛⎞5 ⎛⎞u ⎜⎟1 = ⎜⎟ ⎜⎟⎜⎟v ⎜⎟4 ⎜⎟= 3 1 ⎝⎠ ⎝⎠ ⎝⎠v ⎜⎟ ⎝⎠2 Solve ⎝⎠2 L Triangle systems are easy to solve (by back-substitution.) 2010-11-15 Lecture 9 slide 7 UseUse ofof LULU FactorizationFactorization ⎛⎞10 ⎛⎞u ⎛⎞5 ⎛⎞5 ⎜⎟ ⎛⎞u ⎜⎟ 1 ⎜⎟= ⎜⎟ ⎜⎟= 3 ⎜⎟1 ⎝⎠v ⎝⎠4 ⎝⎠v ⎜⎟ ⎝⎠2 ⎝⎠2 U U ⎛⎞21 ⎛ 5⎞ ⎛⎞21 ⎜⎟⎛⎞x ⎜⎟ ⎛⎞xu ⎛⎞ 33⎜⎟= ⎜⎟ ⎜⎟0 y ⎜⎟ 3 ⎜⎟= ⎜⎟ ⎝⎠ ⎜⎟0 ⎝⎠y ⎝v ⎠ ⎝⎠22 ⎝⎠ ⎝⎠2 Solving the U-system: ⎛⎞x ⎛2⎞ ⎜⎟= ⎜⎟ ⎝⎠y ⎝1⎠ 2010-11-15 Lecture 9 slide 8 LULU FactorizationFactorization A= LU= U The task of L & U L factorization is to find the elements in matrices L and U. Ax=== L( Ux) Ly b 1. Let y = Ux. 2. Solve y from Ly = b 3. Solve x from Ux = y 2010-11-15 Lecture 9 slide 9 AdvantagesAdvantages ofof LULU FactorizationFactorization • When solving Ax = b for multiple b, but the same A, then we only LU-factorize A only once. • In circuit simulation, entries of A may change, but structure of A does not alter. – This factor can used to speed up repeated LU- factorization. – Implemented as symbolic factorization in the “sparse1.3” solver in Spice 3f4. 2010-11-15 Lecture 9 slide 10 GaussianGaussian EliminationElimination • Gaussian elimination is a process of row transformation Ax = b aaa11 12 13 a1n b1 aaa21 22 23 a2n b2 aaa31 32 33 a3n b3 aaannn123 a nnbn Eliminate the lower triangular part 2010-11-15 Lecture 9 slide 11 GaussianGaussian EliminationElimination a11 ≠ 0 aa11 12 a13 a1n b1 aaa a b a 21 22 23 2n 2 − i 1 aa31 32 a33 a3n b3 a11 aann12an3 annbn aa11 12 a13 a1n b1 0 aa22 23 a2n b2 0 aa32 33 a3n b3 Entries updated 0 aann23 ann bn 2010-11-15 Lecture 9 slide 12 EliminatingEliminating 11stst ColumnColumn • Column elimination is equiv to row transformation ⎡ 100 0⎤ aaa a ⎡ 11 12 13 1n ⎤ b1 ⎢ a ⎥ ⎢ − 21 10 0⎥ ⎢ ⎥ a aaa21 22 23 a2n b ⎢ 11 ⎥ ⎢ ⎥ 2 ⎢ a ⎥ ⎢ − 31 01 0⎥ X ⎢aaa31 32 33 a3n ⎥ b3 ⎢ a 11 ⎥ ⎢ ⎥ ⎢ ⎥ ⎢ ⎥ ⎢ ⎥ ⎢ ⎥ a n 1 ⎢aaa a⎥ b ⎢ − 00 1⎥ ⎣ nnn123 nn⎦ n ⎣ a 11 ⎦ ⎡aa11 12 a 13 a1n ⎤ b1 ⎢ (2) (2) (2) ⎥ (2) 0 aa22 23 a2n b2 -1 (2) ⎢ ⎥ L A = A (2) 1 ⎢ 0 aa(2) (2) a(2) ⎥ b ⎢ 32 33 3n ⎥ 3 ⎢ ⎥ ⎢ (2) (2) (2) ⎥ (2) ⎣ 0 aann23 a nn⎦ bn 2010-11-15 Lecture 9 slide 13 EliminatingEliminating 22ndnd ColumnColumn ⎡ 100 0⎤ ⎡aa11 12 a 13 a1n ⎤ ⎢ 010 0⎥ ⎢ ⎥ ⎢ ⎥ 0 aa(2) (2) a(2) ⎢ (2) ⎥ ⎢ 22 23 2n ⎥ a 32 ⎢ − 10 ⎥ (2) (2) (2) a (2) X ⎢ ⎥ ⎢ 22 ⎥ 0 aa32 33 a3n ⎢ ⎥ ⎢ ⎥ ⎢ ⎥ ⎢ ⎥ ⎢ a (2) ⎥ n 2 ⎢ 001− (2) ⎥ ⎢ (2) (2) (2) ⎥ a ⎣ 22 ⎦ ⎣ 0 aann23 a nn⎦ (2) a22 ≠ 0 ⎡aa11 12 a 13 a1n ⎤ b1 ⎢ ⎥ 0 aa(2) (2) a(2) (2) ⎢ 22 23 2n ⎥ b2 L -1 A(2) = A(3) 2 ⎢ 00aa(3) (3) ⎥ b (3) ⎢ 33 3n ⎥ 3 ⎢ ⎥ ⎢ ⎥ 00aa(3) (3) (3) ⎣ nn3 n⎦ bn 2010-11-15 Lecture 9 slide 14 ContinueContinue onon EliminationElimination • Suppose all diagonals are nonzero aaa a ⎡ 11 12 13 1n ⎤ b1 ⎢ ⎥ aaa a b ⎢ 21 22 23 2n ⎥ 2 L -1 L -1 ••• L -1 L -1 n n-1 2 1 ⎢aaa31 32 33 a3n ⎥ b3 ⎢ ⎥ ⎢ ⎥ b ⎣⎢aaannn123 a nn⎦⎥ n Upper triangular aa a a ⎡ 11 12 13 1n ⎤ b1 ⎢ ⎥ 0 aa(2) (2) a(2) (2) ⎢ 22 23 (n) 2n ⎥ b2 (n) (n) ⎢ 00aaA(3) (3) ⎥ b (3) A x = b ⎢ 33 3n ⎥ 3 ⎢ ⎥ ⎢ ()n ⎥ b ()n ⎣ 00 0 ann ⎦ n 2010-11-15 Lecture 9 slide 15 TriangularTriangular SystemSystem Gaussian elimination ends up with the following upper triangular system of equations ⎡⎤aa11 12 a 13 a1n ⎛⎞x ⎛ b1 ⎞ ⎢⎥1 ⎜ ⎟ 0 aa(2) (2) a(2) ⎜⎟x b(2) ⎢⎥22 23 2n ⎜⎟2 ⎜ 2 ⎟ ⎢⎥(3) (3) ⎜ (3) ⎟ 00aa33 3n ⎜⎟x 3 = b3 ⎢⎥⎜⎟⎜ ⎟ ⎢⎥ ⎜⎟ ⎜ ⎟ ⎢⎥()nn⎜⎟x ⎜ ()⎟ ⎣⎦00 0 abnn ⎝⎠n ⎝n ⎠ Solve this system from bottom up: xn, xn-1, ..., x1 2010-11-15 Lecture 9 slide 16 LULU FactorizationFactorization • Gaussian elimination leads to LU factorization -1 -1 -1 -1 ( Ln Ln-1 ••• L2 L1 ) A = U L = (L L ••• L L ) A = (L1 L2 ••• Ln-1 Ln) U = LU 1 2 n-1 n ⎡ 100 0⎤ ⎡⎤aa11 12 a 13 a1n ⎢ a ⎥ ⎢ 21 10 0⎥ ⎢⎥(2) (2) (2) ⎢ a 11 ⎥ 0 aa22 23 a2n ⎢ ⎥ ⎢⎥ aa(2) (3) (3) ⎢ 31 32 10 ⎥ ⎢⎥00aa ⎢ (2) ⎥ 33 3n a 11 a ⎢⎥ ⎢ 22 ⎥ ⎢ ⎥ ⎢⎥ ⎢ (2) ⎥ aa ⎢⎥()n ⎢ nn12 ⎥ 00 0 a (2) *1 ⎣⎦nn ⎣⎢ a 11 a 22 ⎦⎥ 2010-11-15 Lecture 9 slide 17 ComplexityComplexity ofof LULU a11 ≠ 0 aa11 12 a13 a1n a − 21 aaa a a11 21 22 23 2n a 31 − aa31 32 a33 a3n a11 a − n 1 aann12an3 ann a11 # of mul / div = (n-1)*n ≈ n2 n >> 1 n 1 ∑in23=+(1n)(2n+1)∼ O(n) i =1 6 2010-11-15 Lecture 9 slide 18 CostCost ofof BackBack--SubstitutionSubstitution ⎡⎤aa11 12 a 13 a1n ⎛⎞x ⎛ b1 ⎞ ⎢⎥1 ⎜ ⎟ 0 aa(2) (2) a(2) ⎜⎟x b(2) ⎢⎥22 23 2n ⎜⎟2 ⎜ 2 ⎟ ⎢⎥(3) (3) ⎜ (3) ⎟ 00aa33 3n ⎜⎟x 3 = b3 ⎢⎥⎜⎟⎜ ⎟ ⎢⎥ ⎜⎟ ⎜ ⎟ ⎢⎥()nn⎜⎟x ⎜ ()⎟ ⎣⎦00 0 abnn ⎝⎠n ⎝n ⎠ (1)(1)nn b ()n bax− − − n x = nnnn−−11, x n = ()n n −1 (1)n − ann ann−−1, 1 n 1 Total # of mul / div = ∑innOn=+(1)()∼ 2 i =1 2 2010-11-15 Lecture 9 slide 19 ZeroZero DiagonalDiagonal Example 1: After two steps of Gaussian elimination: ×⎡ ×0 0 0⎤ 0 ×⎡ ×0 0 0⎤ 0 0⎢ × 0 0× ⎥ 0 ⎢ ⎥ 0⎢ × 0 0× ⎥ 0 ⎢ 1 1 ⎥ ⎢ ⎥ ⎢0 0 − 1 0⎥ ⎢ 1 1 ⎥ RR ⎢0 0 − 1 0⎥ ⎢ ⎥ RR ⎢ 1 1 ⎥ ⎢ ⎥ 0 0 − 0 1 0⎢ 0 0 0 1⎥ 1 ⎢ RR ⎥ ⎢ ⎥ 0⎢ 0 0 × × 0 ⎥ ⎢0 0 × 0× 0⎥ ⎢ ⎥ ⎢ ⎥ 0⎣⎢ 0 0 × × 0 ⎦⎥ 0⎣ 0 0 × × 0 ⎦ Gaussian elimination cannot continue 2010-11-15 Lecture 9 slide 20 PivotingPivoting Solution 1: Interchange rows to bring a non-zero element into position (k, k): ⎡⎤011 ⎡⎤xx0 ⎢⎥ ⎢⎥011 ⎢⎥××0 ⎢⎥ ⎣⎦⎢⎥××0 ⎣⎦⎢⎥xx0 Solution 2: How about column exchange? Yes Then the unknowns are re-ordered as well. ⎡101⎤ ⎡⎤011 ⎢ ⎥ ⎢⎥ xx0 ⎢⎥××0 ⎢ ⎥ ⎣⎦⎢⎥××0 ⎣⎢xx0⎦⎥ In general both rows and columns can be exchanged! 2010-11-15 Lecture 9 slide 21 SmallSmall DiagonalDiagonal − 4 1 . 25⎡ × 10 1⎤ .⎡ x 251 ⎤ 6⎡ . 25⎤ Example 2: ⎢ ⎥ ⎢ ⎥ = ⎢ ⎥ 12⎣⎢ . 5 12⎦⎥ ⎣ .x 2 5⎦ ⎣ 75 ⎦ Assume finite arithmetic: 3-digit floating point, we have 5 − 4 −10 1 .⎡ 25× 10 1 . 25 ⎤ ⎡ x 1 ⎤ 6⎡ . 25⎤ ⎢ ⎥ ⎢ ⎥ = ⎢ ⎥ ⎣⎢ 12 . 5 12 .⎦⎥ 5⎣ x 2 ⎦ ⎣ 75 ⎦ pivoting 1 . 25⎡ × 10− 4 1 . 25⎤ ⎡ x ⎤ ⎡ 6 . 25 ⎤ ⎢ ⎥ 1 = 5 ⎢ ⎥ ⎢ 5 ⎥ ⎣⎢ 0 1− . 25 × 10⎦⎥ ⎣ x 2 ⎦ 6⎣⎢− . 25 × 10⎦⎥ 12.5 rounded off ⎪⎧ x 2 = 5 ⎨ − 4 x = 0 ( 1 . 25⎩⎪ 10× )x 1 + ( 1 .x 252 = ) 6 .1 25 Unfortunately, (0, 5) is not the solution. Considering the 2nd equation: 12.5 * 0 + 12.5 * 5 = 62.5 ≠ 75. 2010-11-15 Lecture 9 slide 22 AccuracyAccuracy DependsDepends onon PivotingPivoting 5 − 4 −10 1 .⎡ 25× 10 1 . 25 ⎤ ⎡ x 1 ⎤ 6⎡ . 25⎤ ⎢ ⎥ ⎢ ⎥ = ⎢ ⎥ ⎣⎢ 12 . 5 12 .⎦⎥ 5⎣ x 2 ⎦ ⎣ 75 ⎦ pivoting Reason: a11 (the pivot) is too small relative to the other numbers! Solution: Don't choose small element to do elimination. Pick a large element by row / column interchanges. Correct solution to 5 digit accuracy is x1 = 1.0001 x2 = 5.0000 2010-11-15 Lecture 9 slide 23 WhatWhat causescauses accuracyaccuracy problem?problem? • Ill Conditioning: The A matrix close to singular • Round-off error: Relative magnitude too big x− y= 0 x+ y= 1 x− y =0 x+ y= 1 x− y= 0 x− y= 0 1x .− 01 y = 0 . 01 Í resulting in numerical errors ill-conditioned 2010-11-15 Lecture 9 slide 24 PivotingPivoting StrategiesStrategies 1. Partial Pivoting 2. Complete Pivoting 3. Threshold Pivoting 2010-11-15 Lecture 9 slide 25 PivotingPivoting StrategyStrategy 11 1. Partial Pivoting: (Row interchange only) Choose r as the smallest integer such that: ()k ()k k a rk = max a jk j= k,..., n U k Rows k to n L r Search Area 2010-11-15 Lecture 9 slide 26 PivotingPivoting StrategyStrategy 22 2.
Details
-
File Typepdf
-
Upload Time-
-
Content LanguagesEnglish
-
Upload UserAnonymous/Not logged-in
-
File Pages60 Page
-
File Size-