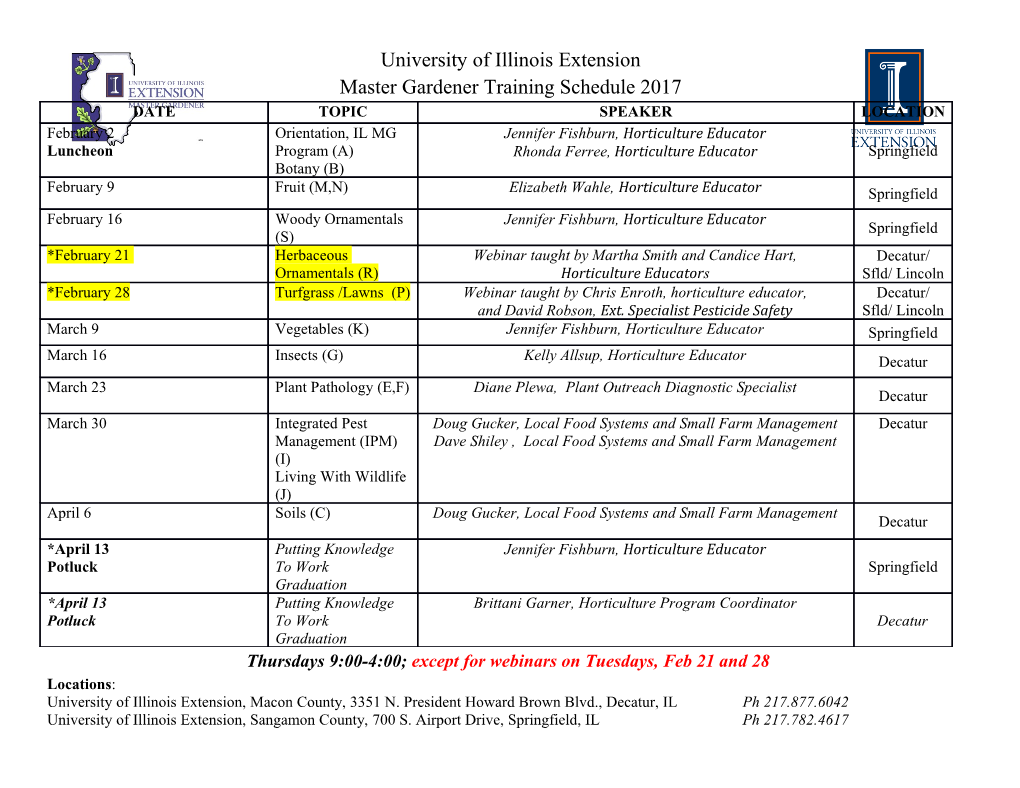
RSS International Conference Abstracts booklet GLA SG OW 4 – 7 September 2017 www.rss.org.uk/conference2017 Abstracts are ordered in date, time and session order 1 Plenary 1 - 36th Fisher Memorial Lecture Monday 4 September – 6pm-7pm And thereby hangs a tail: the strange history of P-values Stephen Senn Head of the Competence Center for Methodology and Statistics, Luxembourg Institute of Health RA Fisher is usually given the honour and now (increasingly) the shame of having invented P-values. A common theme of criticism is that P-values give significance too easily and that their cult is responsible for a replication crisis enveloping science. However, tail area probabilities were calculated long before Fisher and naively and commonly given an inverse interpretation. It was Fisher who pointed out that this interpretation was unsafe and who stressed an alternative and more conservative one, although even this can be found in earlier writings such as those of Karl Pearson. I shall consider the history of P-values and inverse probability from Bayes to Fisher, passing by Laplace, Pearson, Student, Broad and Jeffreys, and show that the problem is not so much an incompatibility of frequentist and Bayesian inference, as an incompatibility of two approaches to dealing with null hypotheses. Both these approaches are encountered in Bayesian analyses, with the older of the two much more common. They lead to very different inferences in the Bayesian framework but much lesser differences in the frequentist one. I conclude that the real problem is that there is an unresolved difference of practice in Bayesian approaches. This does not, of itself, constitute a defence of P-values but it does suggest that some of the problems for which they are blamed will not be resolved merely by abandoning them. 2 1.1 Contributed – Medical Statistics: Clinical Trials Tuesday 5 September – 9am-10am The analysis of two-stage randomised trials when some participants are indifferent to treatment choice Stephen Walter, Robin Turner, Petra Macaskill, Kirsten McCaffery, Les Irwig McMaster University Participants in clinical trials would often prefer to receive one of the treatments being compared, if that were allowed. Effects of preferences on study outcomes can be important, but they are unidentifiable in standard trial designs. However they can be estimated using a two-stage trial design, in which a random subset of patients are indeed permitted to choose their treatment, while the remaining patients are randomised in the usual way. We have previously discussed the optimum design and analysis of the two-stage randomised trial, but we assumed that all participants have a preferred treatment [1,2]. We now extend this work to allow for some participants to have no preference, even if they are offered a choice. We obtain unbiased estimates and tests of the effects of preferences on study outcomes, as well as the usual direct treatment effect, in this context. This approach will be illustrated using a medical versus surgical trial in which 69% of participants in the choice arm had no preferred treatment. We conclude that the two-stage design remains attractive even if some participants have no stated treatment preference. It allows insight into potentially important determinants of study outcomes that cannot be estimated in other trials. Furthermore the outcomes in trials participants with various treatment preferences, including being indifferent, are themselves of interest to trial investigators, and may inform optimum treatment decision-making for future patients. 1. Walter SD, Turner RM, Macaskill P, McCaffery KJ, Irwig L. Beyond the treatment effect: evaluating the effects of patient preferences in randomised trials. Statistical Methods in Medical Research, 2014. 2. Walter SD, Turner RM, Macaskill P, McCaffery KJ, Irwig L. Optimal allocation of participants for the estimation of selection, preference and treatment effects in the two-stage randomised trial design. Statistics in Medicine 31, 1307-22, 2012 3 1.1 Contributed – Medical Statistics: Clinical Trials Tuesday 5 September – 9am-10am Covariate adjustment and prediction of mean response in randomised trials Jonathan Bartlett AstraZeneca A key quantity which is almost always reported from a randomised trial is the mean outcome in each treatment group. When baseline covariates are collected, these can be used to adjust these means to account for imbalance in the baseline covariates between groups, thereby resulting in a more precise estimate. Qu and Luo (DOI: 10.1002/pst.1658) recently described an approach for estimating baseline adjusted treatment group means which, when the outcome model is non-linear (e.g. logistic regression), is more appropriate than the conventional approach which predicts the mean outcome for each treatment group, setting the baseline covariates to their mean values. In this talk I will first emphasize that when the outcome model is non-linear, the aforementioned `conventional’ approach estimates a different quantity than the unadjusted group means and `Qu and Luo’ estimator. I will then describe how for many commonly used outcome model types, the Qu and Luo estimates are unbiased even when the outcome model is misspecified. Qu and Luo described how standard errors and confidence intervals can be calculated for these estimates, but treated the baseline covariates as fixed constants. When, as is usually the case in trials, the baseline covariates of patients would not be fixed in repeated sampling, I show that these standard errors are too small. I will describe a simple modification to their approach which provides valid standard errors and confidence intervals. I will then discuss the impact of stratified randomisation and missing outcomes on the preceding results, and give suggestions for when baseline adjusted means may or may not be preferred to unadjusted means. The analytical results will be illustrated through simulations and application to a recently conducted trial with recurrent events analysed using negative binomial regression. 4 1.1 Contributed – Medical Statistics: Clinical Trials Tuesday 5 September – 9am-10am Common pitfalls in Oncology Trials Arnaud Demange, Alan Phillips, Jia Ma ICON Clinical Research Oncology trials fail far too frequently, and often for similar reasons. Understanding the risks in oncology trial designs and analyses, and how to mitigate them, will equip developers to reduce the failure rate in late stage oncology trials and move effective drugs to market faster. We have observed oncology studies fail frequently due to five prevalent issues: 1. assumptions that undermined a trial’s design; 2. inadequate patient selection criteria; 3. problematic phase 2 trials; 4. over-interpretation of subgroup analyses; and 5. optimistic selection of a non-inferiority margin. This presentation will review these common issues in the context of specific failed trials and propose practical countermeasures that would not compromise study integrity, including adopting an adaptive design and deploying a more stringent statistical significance level to minimize the false discovery rate. 5 1.2 Contributed - Official statistics and public policy: Use of the Census Tuesday 5 September – 9am-10am An online census: Will people respond online? Characteristics of online and paper respondents David Corps, Victor Meirinhos Office for National Statistics The 2021 Census in England and Wales will be a primarily online census for the first time. To understand further the willingness to respond online and the characteristics of those who may be unwilling or unable to respond online, a large-scale test was designed for early 2017. Sixty thousand households were sent either a paper questionnaire or a letter including a unique access code enabling online response. Anyone receiving a unique access code could request a paper questionnaire and those who received a paper questionnaire could choose to respond online. Here, we discuss the differences in response rates by mode for those given a paper questionnaire and those sent a unique access code. Furthermore, we present the analyses of characteristics of respondents, using a mixed effects modelling approach to investigate differences in characteristics among those who respond via paper or online for those given a paper questionnaire, and those responding online or via paper for those sent a unique access code. This research will play an important role in informing the design of the 2021 Census and in understanding more about the digitally excluded population in general in an increasingly digital world. 6 1.2 Contributed - Official statistics and public policy: Use of the Census Tuesday 5 September – 9am-10am New opportunities and challenges in census coverage assessment Viktor Racinskij Office for National Statistics By definition census is a complete enumeration of a population of interest. However, when dealing with large and complex human populations census coverage errors are inevitable. Estimation of the coverage errors has been a vibrant area in statistics for over sixty years. In the context of the Census of England and Wales, the work related to the coverage error estimation is known as coverage assessment and it is one of the key components in producing high quality population statistics. Innovations in data collection and pre-processing open a wider range of opportunities for the coverage assessment methods compared to what was available in the past. Unsurprisingly, the new opportunities bring new challenges. This talk covers
Details
-
File Typepdf
-
Upload Time-
-
Content LanguagesEnglish
-
Upload UserAnonymous/Not logged-in
-
File Pages356 Page
-
File Size-