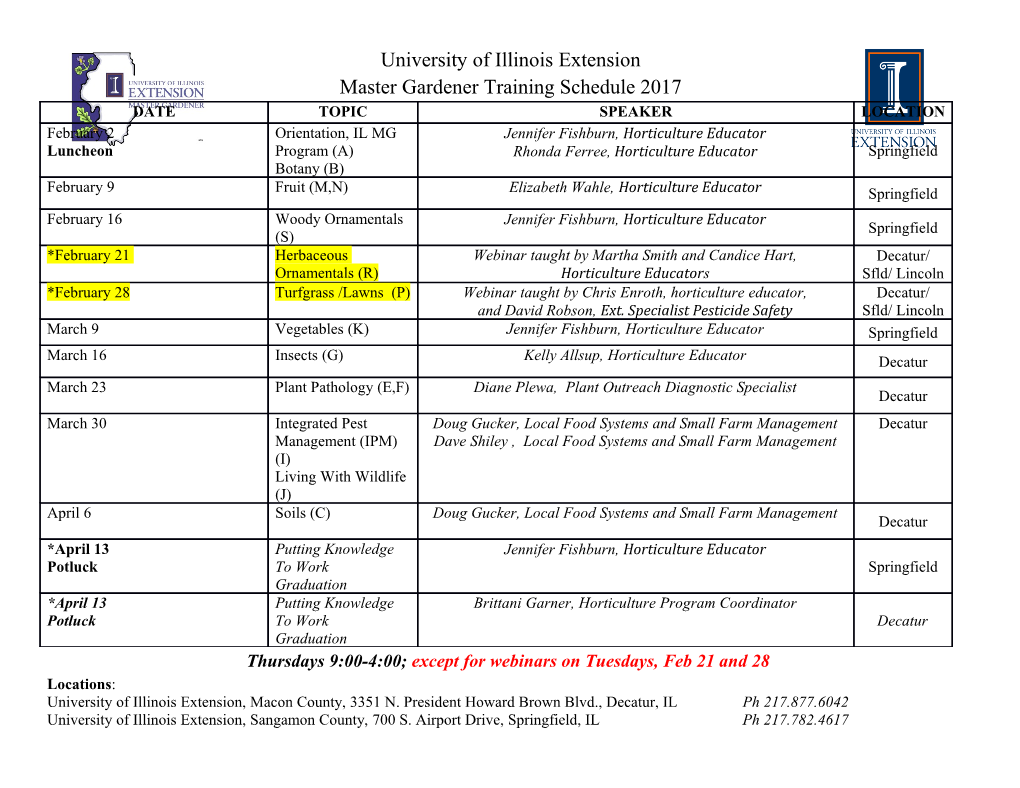
Statistical Analysis of Vehicle Crash Incidents at the Minnesota USA Interstate 494 and Interstate 35 West Interchange using Chi-Square Statistic Goodness-of-Fit Testing Candace Roberts Department of Resource Analysis, Saint Mary’s University of Minnesota, Minneapolis, MN 55404 Keywords: Vehicle Crash Incidents, Minneapolis I-494 and I-35W Cloverleaf Interchange, Contributing Factors, Chi-Square Goodness-of-Fit, Contingency Tables Abstract The Minneapolis, Minnesota (USA) Interstate 494 and Interstate 35 West cloverleaf interchange experiences a continued overcapacity daily traffic volume, creating the lowest safety ranking of highway interchanges in the metropolitan area. During a 10-year period from 2006 through 2015, an average 8.45 monthly crash incidents were reported. The majority of crashes occurred during good weather conditions and were attributed to driver behavior factors. This study utilized contingency tables and Chi-Square Goodness-of-Fit tests to determine associations and statistical equality of contributing factors that influenced crash incidents. Significant findings point to contributing factors for which improvements, in response to human behaviors, will help mitigate such occurrences at this interchange. Introduction However, this creates a juxtapositional situation as traffic interweaves lanes while Population growth in the Minneapolis simultaneously adjusting to decreasing (or metro area (USA) has been steadily increasing) speeds depending on exiting increasing over the past years (U.S. (or entering) pathway. Hotchin (2017) Census Bureau, 2018) and this has states, “…weaving is a problem that may increased demand on area infrastructure. lead to breakdown in traffic operation and Growing traffic volume produces stress on [lead to] more accidents.” aging highways and interchanges making The I-494 and I-35W cloverleaf traffic safety a concern. The Interstate 494 interchange was constructed in the 1960’s and Interstate 35 West (I-494 and I-35W) and has a continued overcapacity daily cloverleaf interchange, located in the traffic volume; the daily traffic volume in Minneapolis suburb of Bloomington, is an 2014 was 290,000 vehicles (Minnesota example of this aging interchange under Department of Transportation, 2013). Its stress. safety ranking is among the worst for The cloverleaf structure was interchanges in the Minneapolis metro designed in the early 20th century to keep area (Short Elliott Hendrickson, Inc., traffic flowing without the use of traffic 2010). Congestion and design flaws on I- signals (Hotchin, 2017). The cloverleaf 494 and I-35W arteries impact the design utilizes four loops (ramps) to interchange in a shockwave effect (SEH) transfer traffic flow seamlessly from one creating stress on traffic maneuvering highway to another while maintaining through the interchange. During a 10-year right-lane exit and entrance flow. span, 2006 through 2015, an average 8.45 Roberts, Candace. 2018. Statistical Analysis of Vehicle Crash Incidents at the Minnesota USA Interstate 494 and Interstate 35 West Interchange using Chi-Square Statistic Goodness-of-Fit Testing. Volume 21, Papers in Resource Analysis. 15 pp. Saint Mary’s University of Minnesota University Central Services Press. Winona, MN. Retrieved (date) http://www.gis.smumn.edu monthly crash incidents were reported as significance with the Chi-Square evident in this study’s dataset. Goodness-of-Fit test to determine The state of Minnesota statistical equality. documentation on traffic collision occurrence preferences the term “crash” to Data “accident” since accident suggests “a random, unavoidable quality about the Historical crash data were obtained from events in question” (Minnesota the Minnesota Department of Department of Public Safety, 2016). Transportation in shapefile and CSV When, in fact, the reduction in the number formats. The data incorporated crash and severity of crashes over the past incidents within 1250’ from the center of decades is attributed to advances in the I-494 and I-35W interchange from technology, engineering, public policy, 2006 to 2015. The dataset contained 1014 and driver behavior, which points to crash incidents involving single and preventable instances rather than random multiple vehicle collisions. accounts (MnDPS). Three criteria are The shapefiles revealed the required to define a motor vehicle crash: location for 87% of incidents occurred at occurrence on a public road, minimum the center of the interchange without $1000 damage or a person is injured, dispersion of occurrence elsewhere. Either transport of motor vehicle (MnDPS; this indicated most likely location for a Minnesota Department of Public Safety, crash to occur or data recording n.d.). preference. In either case, location Understanding impacts leading to analysis, such as hot spot clustering, were vehicle crashes by analyzing historical deemed insignificant in determining areas data provides valuable information in of most likely occurrences due to crash efforts to mitigate such occurrences at the location accuracies. Nonetheless, all 1014 I-494 and I-35W cloverleaf interchange. points are shown in Figure 1, of which 881 This study utilizes contingency tables and points are displayed at the same location. Chi-Square Goodness-of-Fit tests to determine significant association and statistical equality using weather conditions, driver age, driver behavior, vehicle safety, time, and collision type of 1014 crash incidents from 2006 to 2015 at the I-494 and I-35W cloverleaf interchange. Methods Motor vehicle crash occurrences of the I- 494 and I-35W interchange from 2006 to 2015 were extensively analyzed using contingency tables to calculate the Chi- Square Goodness-of-Fit tests to determine Figure 1. I-494 and I-35W cloverleaf interchange: significant statistical association using 1014 motor vehicle crash occurrences, 2006–2015. single dataset attributes tested for Information analyzed for possible 2 significant statistical association driver behaviors as a contributing factor (indicating influence to crash occurrence) accounted for 43.5% of the crash were month, day, year, time, collision incidents, whereas vehicle safety failures type, weather conditions, driver were accountable for only 3%. Factors contributing factors, and driver age. categorized as ‘not clearly identified as Information analyzed for statistical contributing to crash incident’ were equality testing of occurrence across 2006 44.3%. to 2015 were crash frequencies, two Odds are, if the 44.3% ‘not clearly collision types (rear-end and sideswipe), identified factors’ were countable as and two driver contributing factors (driver vehicle safety failures, the whole 44.3% inattention or distraction, and illegal or would not shift to vehicle safety failures. unsafe driving speeds). At most, 22.2% (or half) would shift and the remaining percentage would shift to Data Preparation driver behavior factors, distributing the 44.3% equally; therefore, distribution of Contributing factors due to driver factors as ‘not clearly identified’ were influence, either human behavior or disregarded. With driver behaviors greater vehicle failure, were recorded in multiple than vehicle safety failures, driver fields as were weather conditions. The behaviors as contributing factors attributed fields were equally weighted. Therefore, at a higher percentage to crash incidents in the fields were analyzed as separate the n=1014 dataset case study. entities, rather than the whole entities as The 2015 Minnesota crash analysis recorded in the crash incident to extract (MnDPS) reports, “…most crashes occur influence of a single entity. in good driving conditions” which In simplifying weather conditions included clear weather conditions. The from the two weather fields, clear was n=1014 dataset case study reflected 655 categorized as clear; cloudy or crashes, or 64.6%, occurred during clear clear/cloudy were categorized as cloudy, weather conditions. The second highest reference to rain was categorized as rain, percentage of crash incidents occurred reference to snow was categorized as during cloudy weather conditions at 231 snow, sleet was categorized as sleet, fog crashes (22.8%). Clear or cloudy weather was categorized as fog, and blowing winds conditions comprised 87.4%. In contrast, were categorized as blowing winds. precipitous conditions – rain, snow, sleet – accounted for 12% with remaining 0.6% Extracting Data Subsets attributed to fog and blowing winds. A subset from the dataset (based According to analysis in the Minnesota on the results of the majority of crash Motor Vehicle Crash Facts 2015 incidents were due to driver behaviors (Minnesota Department of Public Safety, during clear or cloudy weather conditions) 2016), driver behaviors are frequently was extracted. The subset contained 402 cited as contributing factors to crash crash incidents. incidents more often over vehicular safety The distribution of crashes here failures. This was demonstrably accurate from 2006 to 2015 is displayed in scatter in the n=1014 dataset case study for the I- plots with the month (as single-letter 494 and I-35W cloverleaf interchange. initials) along the x-axis and the time In the n=1014 dataset case study, along the y-axis. Figure 2 is the original 3 dataset case study (n=1014); Figure 3 is referenced in Zar (2010). the data subset (n=402). The distributions are similar with saturation of crash Contingency Tables incidents during the morning rush hour (6:00 a.m. to 9:00 a.m.), afternoon (12:00 A contingency table contains
Details
-
File Typepdf
-
Upload Time-
-
Content LanguagesEnglish
-
Upload UserAnonymous/Not logged-in
-
File Pages15 Page
-
File Size-