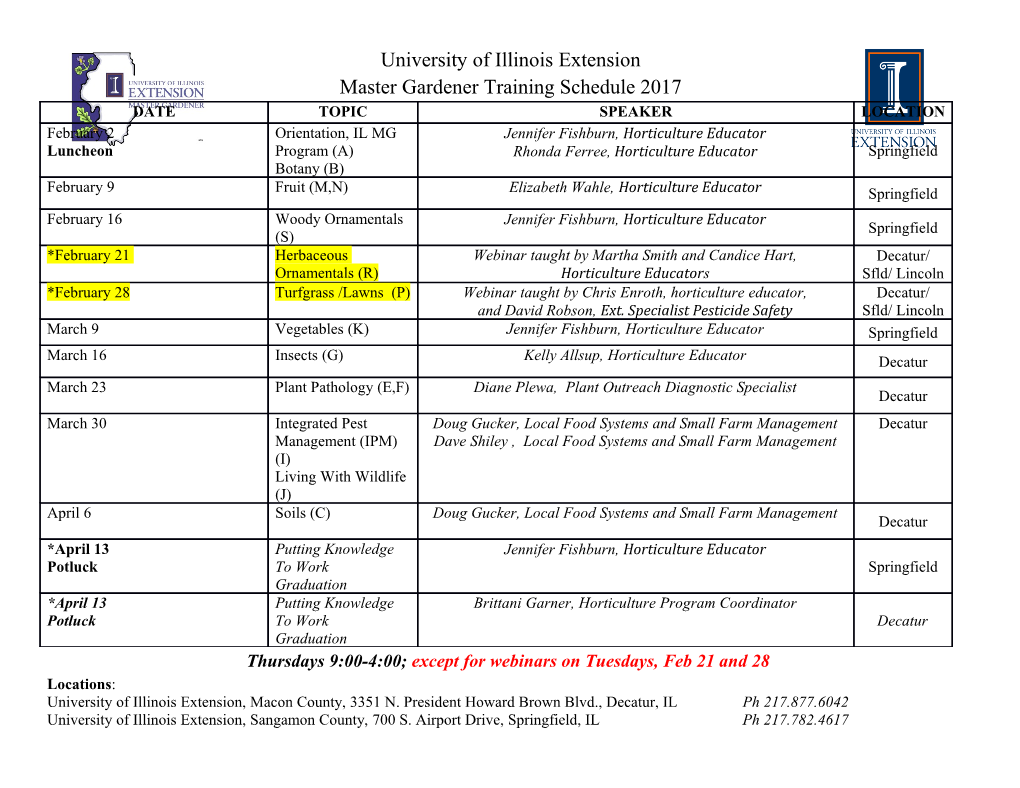
GENERALIZED EIGENVECTORS, MINIMAL POLYNOMIALS AND THEOREM OF CAYLEY-HAMILTION FRANZ LUEF Abstract. Our exposition is inspired by S. Axler’s approach to linear algebra and follows largely his exposition in ”Down with Determinants”, check also the book ”LinearAlgebraDoneRight” by S. Axler [1]. These are the lecture notes for the course of Prof. H.G. Feichtinger ”Lineare Algebra 2” from 15.11.2006. Before we introduce generalized eigenvectors of a linear transformation we recall some basic facts about eigenvalues and eigenvectors of a linear transformation. Let V be a n-dimensional complex vector space. Recall a complex number λ is called an eigenvalue of a linear operator T on V if T − λI is not injective, i.e. ker(T − λI) 6= {0}. The main result about eigenvalues is that every linear op- erator on a finite-dimensional complex vector space has an eigenvalue! Furthermore we call a vector v ∈ V an eigenvector of T if T v = λv for some eigenvalue λ. The central result on eigenvectors is that Non-zero eigenvectors corresponding to distinct eigenvalues of a linear transformation on V are linearly independent. Consequently the number of distinct eigenvalues of T can- not exceed thte dimension of V . Unfortunately the eigenvectors of T need not span V . For example the linear transformation on C4 whose matrix is 0 1 0 0 0 0 1 0 T = 0 0 0 1 0 0 0 0 as only the eigenvalue 0, and its eigenvectors form a one-dimensional subspace of C4. Observe that T,T 2 6= 0 but T 3 = 0. More generally a linear operator T such that T,T 2, ..., T p−1 6= 0 and T p = 0 is called nilpotent of index p. More generally, let T be a linear operator on V , then the space of all linear operators on V is finite- dimensional (actually of dimension n2). Then there exists a smallest positive integer k such that I,T,T 2, ..., T k are not linearly independent. In other words there exist unique complex numbers a0, a1, ..., ak−1 such that k−1 k a0I + a1T + ··· + ak−1T + T = 0. k−1 k The polynomial m(x) = a0 + a1x + ··· + ak−1z + z is called the minimal poly- nomial of T . It is the monic polynomial of smallest degree such that m(T ) = 0. A polynomial q such that q(T ) = 0 is a so-called annihilating polynomial. The 1 Fundamental Theorem of Algebra yields that α1 α2 αm m(x) = (x − λ1) (x − λ2) ··· (x − λm) , where αj is the multiplicity of the eigenvalue λj of T . Since α1 α2 αm m(T ) = (T − λ1I) (T − λ2I) ··· (T − λmI) = 0 αj αj implies that for some j (T − λj) = 0 is not injective, i.e. ker(T − λjI) 6= {0}. What is the structure of the subspace ker(T − λjI)? First of all we call a vector v ∈ V a generalized eigenvector of T if (T − λI)kv = 0 for some eigenvalue λ of T . Then ker(T − λI)k is the space of all generalized eigenvectors of T corresponding to an eigenvalue λ. Lemma 0.1. The set of generalized eigenvectors of T on a n-dimensional complex vector space corresponding to an eigenvalue λ equals ker(T − λI)n. Proof. Obviously, every element of ker(T − λI)n is a generalized eigenvector of T corresponding to λ. Let us show the other inclusion. If v 6= 0 is a generalized eigenvector of T corre- sponding to V , then we need to prove that (T − λI)nv = 0. By assumption there is a smallest non-negative integer k such that (T − λI)kv = 0. We are done if we show that k ≤ n. In other words we proof that v, (T − λI)v, ..., (T − λI)k−1v are linearly independent vectors. Since then we will have k linearly independent elements in an n-dimensional vector space, which implies that k ≤ n. Let a0, a1, ..., ak−1 be complex numbers such that k−1 a0v + a1(T − λI)v + ··· + ak−1(T − λI) v = 0. k−1 k−1 Apply (T − λI) to both sides of the equation above, getting a0(T − λI) v = 0, k−2 which yields a0 = 0. Now apply (T − λI) to both sides of the equation, getting k−1 a1(T − λI) v = 0, which implies a1 = 0. Continuing in this fashion, we see that aj = 0 for each j, as desired. Following the basic pattern of the proof that non-zero eigenvectors corresponding to discinct eigenvalues of T are linearly independent, we obtain: Proposition 0.2. Non-zero generalized eigenvectors corresponding to distinct eigen- values of T are linearly independent. Proof. Suppose that v1, .., vm are non-zero generalized eigenvectors of T correspond- ing to distinct eigenvalues λ1, ..., λm. We assume that there are complex numbers a1, ..., am such that a1v1 + a2v2 + ··· + amvm = 0. Then we have to show that a1 = a2 = ··· = am = 0. Let k be the smallest positive k integer such that (T − λI) v1 = 0. Then apply the linear operator k−1 n n (T − λ1I) (T − λ2I) ··· (T − λmI) 2 to both sides of the previous equation, getting k−1 n n a1(T − λ1I) (T − λ2I) ··· (T − λmI) v1 = 0. n n We rewrite (T − λ2I) ··· T − λmI) as n n ((T − λ1) + (λ1 − λ2)I) ··· (T − λn) + (λ1 − λn)I) v1 = 0. An application of the binomial theorem gives a sum of terms which when combined k−1 with (T − λ1I) on the left and applied to v1 gives 0, except for the term n n k−1 a1(λ1 − λ2) ··· (λ1 − λm) (T − λ1) v1 = 0. Thus a1 −0. Continuing in a similar fashion, we get aj = 0 for each j, as desired. The central fact about generalized eigenvectors is that they span V . Theorem 0.3. Let V be a n-dimensional complex vector space and let λ be an eigenvalue of T . Then V = ker(T − λI)n ⊕ im(T − λI)n. Proof. The proof will be an induction on n, the dimension of V . The result holds for n = 1. Suppose that n > 1 and that the result holds for all vector spaces of dimension less than n. Let λ be any eigenvalue of T . Then we want to show that n n V = ker(T − λI) ⊕ im(T − λI) =: V1 ⊕ V2. n Let v ∈ V1 ∩ V2. Then (T − λI) v = 0 and there exists a u ∈ V such that (T − λI)nu = v. Applying (T − λI)n to both sides of the last equation, we have that 2n n (T − λI) u = 0. Consequently, (T − λI) u = 0, i.e. v = 0. Thus V1 ∩ V2 = {0}. Now V1 and V2 are the kernel and the image of a linear operator on V , we have dim V = dim V1 + dim V2. Note that V1 6= {0}, because λ is an eigenvalue of T , thus dim V2 < n. Furthermore n T maps V2 into V2 since T commutes with (T −λI) . By our induction hypothesis, V2 is spanned by the generalized eigenvectors of T |V2 , each of wich is also a generalized eigenvector of T . Everything in V1 is a generalized eigenvector of T , which gives the desired result. Corollary 0.4. If 0 is the only eigenvalue of a linear operator on V , then T is nilpotent. Proof. By assumption 0 is the only eigenvalue of T . Then every vector v in V is a generalized eigenvector of T corresponding to the eigenvalue λ = 0. Consequently p T = 0 for some p. As a consequence we get the following structure theorem for linear transformations. Theorem 0.5. Let λ1, ..., λm be the distinct eigenvalues of T , with E1, ..., Em de- noting the corresponding sets of generalized eigenvectors. Then (1) V = E1 ⊕ E2 ⊕ · · · ⊕ Em; (2) T maps each Ej into itself; (3) each (T − λjI)|Ej is nilpotent; (4) each T |Ej has only one eigenvalue, namely λj. 3 Proof. (1) Follows from the linear independence of generalized eigenvectors cor- responding to distinct eigenvalues and that the generalized eigenvectors of λj span Ej. k (2) Suppose v ∈ Ej. Then (T − λjI) v = 0 for some positive integer k. Further- more we have k k (T − λj) T v = T (T − λj) v = T (0) = 0, i.e. T v ∈ Uj. (3) is a reformulation of the definition of a generalized eigenvector. (4) Let λ be an eigenvalue of T |Uj , with corresponding non-zero eigenvector v ∈ Uj. Then (T − λjI)v = (λ − λj)v, and hence k k (T − λjI) v = (λ − λj) v for each positive integer k. But v is a generalized eigenvector of T corre- sponding to λj, the left hand side of the equation is 0 for some k, i.e. λ = λj. The next theorem connects the minimal polynomial of T to th decomposition of V as a direct sum of generalized eigenvectors. Theorem 0.6. Let λ1, ..., λm be the distinct eigenvalues of T , let Ej denote the set of the generalized eigenvectors corresponding to λj, and let αj be the smallest positive αj integer such that (T − λjI) v = 0 for every v ∈ Ej. Let α1 α2 αm m(x) = (x − λ1) (x − λ2) ··· (x − λm) . Then (1) m has degree at most dim(V ); (2) if p is another annihilating polynomial of T , then p is a polynomial multiple of m; (3) m is the minimal polynomial of T . Proof. Each αj is at most the dimension of Ej and V = E1 ⊕ · · · ⊕ Em gives that the αj’s can at most add up to n.
Details
-
File Typepdf
-
Upload Time-
-
Content LanguagesEnglish
-
Upload UserAnonymous/Not logged-in
-
File Pages5 Page
-
File Size-