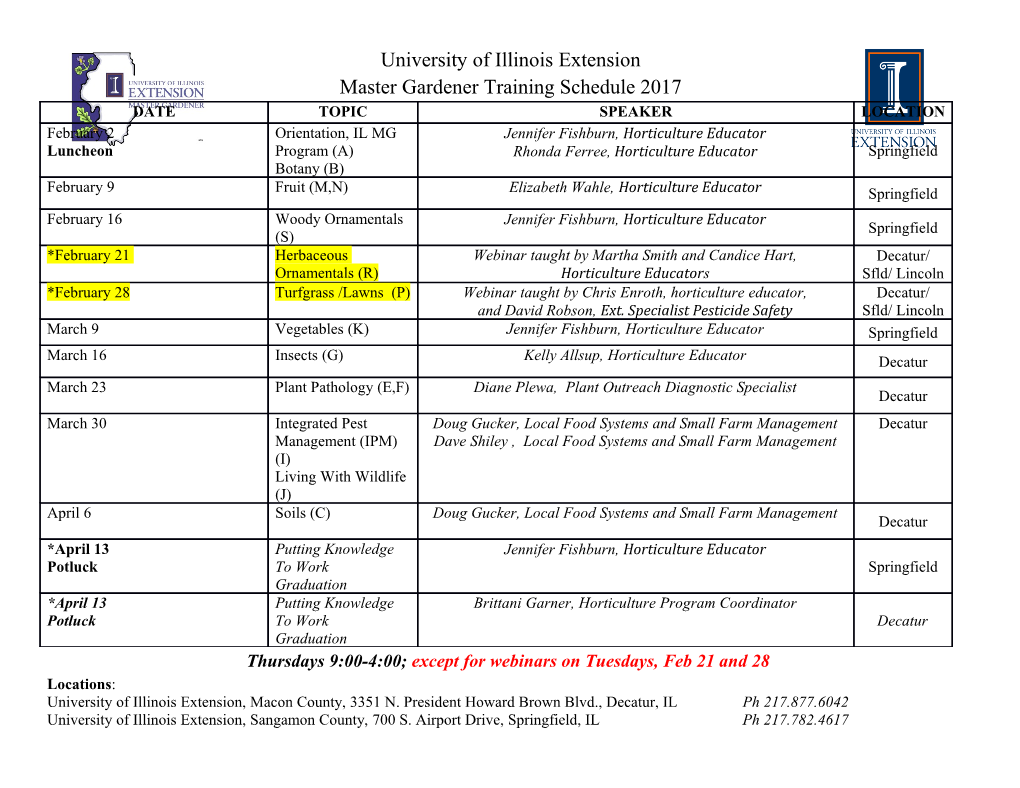
Computing Systems & Performance Beyond Vector/SIMD architectures • Vector/SIMD-extended architectures are hybrid approaches MSc Informatics Eng. – mix scalar + vector operation capabilities on a single device – highly pipelined approach to reduce memory access penalty – tightly-closed access to shared memory: lower latency 2011/12 • Evolution of Vector/SIMD-extended architectures – CPU cores with wider vectors and/or SIMD cores: A.J.Proença • DSP VLIW cores with vector capabilities: Texas Instrument • PPC cores coupled with SIMD cores: Cell Broadband Engine • ARM64 cores coupled with SIMD cores: project Denver (NVidia) • future x86 hybrid cores: Intel, AMD ... Data Parallelism 2 (Cell BE, GPU, ...) – devices with no scalar processor: accelerator devices • penalty on disjoint physical memories (most slides are borrowed) • based on the GPU architecture: SIMD—>SIMT to hide memory latency • ISA-free architecture, code compiled to silica: FPGA AJProença, Computer Systems & Performance, MEI, UMinho, 2011/12 1 AJProença, Computer Systems & Performance, MEI, UMinho, 2011/12 2 Texas Instruments: Keystone DSP architecture Cell Broadband Engine (1) AJProença, Computer Systems & Performance, MEI, UMinho, 2011/12 3 AJProença, Computer Systems & Performance, MEI, UMinho, 2011/12 4 Cell Broadband Engine (2) Cell Broadband Engine (3) AJProença, Computer Systems & Performance, MEI, UMinho, 2011/12 5 AJProença, Computer Systems & Performance, MEI, UMinho, 2011/12 6 Cell Broadband Engine (4) NVidia: Project Denver • Pick a successful SoC: Tegra 3 • Replace the 32-bit ARM Cortex 9 cores by 64-bit ARM cores • Add some Fermi SIMT cores into the same chip AJProença, Computer Systems & Performance, MEI, UMinho, 2011/12 7 AJProença, Computer Systems & Performance, MEI, UMinho, 2011/12 8 What is an FPGA FPGA as a multiple configurable ISA Field-Programmable Gate Arrays (FPGA) A fabric with 1000s of simple configurable logic cells with LUTs, on-chip SRAM, configurable routing and I/O cells AJProença, Computer Systems & Performance, MEI, UMinho, 2011/12 9 AJProença, Computer Systems & Performance, MEI, UMinho, 2011/12 10 The GPU as a compute device: the G80 The CUDA programming model • Compute Unified Device Architecture • CUDA is a recent programming model, designed for – Manycore architectures – Wide SIMD parallelism – Scalability • CUDA provides: – A thread abstraction to deal with SIMD – Synchr. & data sharing between small groups of threads • CUDA programs are written in C with extensions • OpenCL inspired by CUDA, but hw & sw vendor neutral – Programming model essentially identical AJProença, Computer Systems & Performance, MEI, UMinho, 2011/12 11 AJProença, Computer Systems & Performance, MEI, UMinho, 2011/12 12 Graphical Processing Units CUDA Devices and Threads Terminology (and in NVidia) ! Threads of SIMD instructions (warps) • A compute device ! Each has its own PC (up to 48 per SIMD processor) – Is a coprocessor to the CPU or host ! Thread scheduler uses scoreboard to dispatch – Has its own DRAM (device memory)! ! No data dependencies between threads! – Runs many threads in parallel ! Threads are organized into blocks & executed in groups – Is typically a GPU but can also be another type of parallel ! processing device ! of 32 threads (thread block) • Data-parallel portions of an application are expressed as ! Blocks are organized into a grid device kernels which run on many threads - SIMT ! The thread block scheduler schedules blocks to • Differences between GPU and CPU threads SIMD processors (Streaming Multiprocessors) – GPU threads are extremely lightweight ! Within each SIMD processor: • Very little creation overhead, requires LARGE register bank ! 32 SIMD lanes (thread processors) – GPU needs 1000s of threads for full efficiency ! Wide and shallow compared to vector processors • Multi-core CPU needs only a few AJProença, Computer Systems & Performance, MEI, UMinho, 2011/12 13 Copyright © 2012, Elsevier Inc. All rights reserved. 14 © David Kirk/NVIDIA and Wen-mei W. Hwu, 2007-2009 W. and Wen-mei © David Kirk/NVIDIA ECE 498AL, University of Illinois, Urbana-Champaign CUDA basic model: Single-Program Multiple-Data (SPMD) Programming Model: SPMD + SIMT/SIMD • CUDA integrated CPU + GPU application C program • Hierarchy – Device => Grids – Serial C code executes on CPU – Grid => Blocks – Block => Warps – Parallel Kernel C code executes on GPU thread blocks – Warp => Threads • Single kernel runs on multiple blocks ! CPU Code ! (SPMD) Grid 0 • Threads within a warp are executed in a lock-step way called single- GPU Parallel Kernel instruction multiple-thread (SIMT) KernelA<<< nBlk, nTid >>>(args); . • Single instruction are executed on multiple threads (SIMD) CPU Code – Warp size defines SIMD granularity Grid 1 (32 threads) • Synchronization within a block using GPU Parallel Kernel shared memory KernelB<<< nBlk, nTid >>>(args); . Courtesy NVIDIA AJProença, Computer Systems & Performance, MEI, UMinho, 2011/12 15 AJProença, Computer Systems & Performance, MEI, UMinho, 2011/12 16 © David Kirk/NVIDIA and Wen-mei W. Hwu, 2007-2009 W. and Wen-mei © David Kirk/NVIDIA ECE 498AL, University of Illinois, Urbana-Champaign The Computational Grid: CUDA Thread Block Block IDs and Thread IDs • A kernel runs on a computational • Programmer declares (Thread) Block: grid of thread blocks – Block size 1 to 512 concurrent – Threads share global memory threads CUDA Thread Block • Each thread uses IDs to decide – Block shape 1D, 2D, or 3D what data to work on – Block dimensions in threads ! threadID 0 1 2 3 4 5 6 7 ! – Block ID: 1D or 2D ! • All threads in a Block execute the ! – Thread ID: 1D, 2D, or 3D same thread program • A thread block is a batch of … • Threads share data and synchronize float x = input[threadID]; threads that can cooperate by: while doing their share of the work float y = func(x); output[threadID] = y; – Sync their execution w/ barrier • Threads have thread id numbers … – Efficiently sharing data through a within Block low latency shared memory • Thread program uses thread id to – Two threads from two different select work and address shared data blocks cannot cooperate AJProença, Computer Systems & Performance, MEI, UMinho, 2011/12 17 AJProença, Computer Systems & Performance, MEI, UMinho, 2011/12 18 © David Kirk/NVIDIA and Wen-mei W. Hwu, 2007-2009 W. and Wen-mei © David Kirk/NVIDIA ECE 498AL, University of Illinois, Urbana-Champaign Hwu, 2007-2009 W. and Wen-mei © David Kirk/NVIDIA ECE 498AL, University of Illinois, Urbana-Champaign Parallel Memory Sharing CUDA Memory Model Overview Thread • Local Memory: per-thread (Device) Grid – Private per thread • Each thread can: Local Memory – Auto variables, register spill – R/W per-thread registers Block (0, 0) Block (1, 0) • Shared Memory: per-block – R/W per-thread local memory Block Shared Memory Shared Memory – Shared by threads of the same – R/W per-block shared memory block Registers Registers Registers Registers ! – R/W per-grid global memory ! Shared – Inter-thread communication ! ! Memory – Read only per-grid constant • Global Memory: per-application Thread (0, 0) Thread (1, 0) Thread (0, 0) Thread (1, 0) – Shared by all threads memory Grid 0 – Inter-Grid communication – Read only per-grid texture Local Local Local Local memory Memory Memory Memory Memory . Host Global • The host can R/W Memory Global Sequential Grid 1 Memory global, constant, and Constant Grids Memory in Time texture memories . Texture Memory AJProença, Computer Systems & Performance, MEI, UMinho, 2011/12 19 AJProença, Computer Systems & Performance, MEI, UMinho, 2011/12 20 © David Kirk/NVIDIA and Wen-mei W. Hwu, 2007-2009 W. and Wen-mei © David Kirk/NVIDIA ECE 498AL, University of Illinois, Urbana-Champaign Hwu, 2007-2009 W. and Wen-mei © David Kirk/NVIDIA ECE 498AL, University of Illinois, Urbana-Champaign Graphical Processing Units Hardware Implementation: NVIDIA GPU Memory Structures Memory Architecture ! Each SIMD Lane has private section of off-chip Device DRAM • Device memory (DRAM) Multiprocessor N – Slow (2~300 cycles) ! “Private memory” (Local Memory) Multiprocessor 2 ! – Local, global, constant, Multiprocessor 1 Contains stack frame, spilling registers, and private and texture memory variables Shared Memory ! Each multithreaded SIMD processor also has Registers Registers Registers • On-chip memory Instruction … Unit local memory (Shared Memory) – Fast (1 cycle) Processor 1 Processor 2 Processor M ! Shared by SIMD lanes / threads within a block – Registers, Constant Cache shared memory, ! Memory shared by SIMD processors is GPU Texture constant/texture cache Cache Memory (Global Memory) ! Device memory Host can read and write GPU memory Courtesy NVIDIA AJProença, Computer Systems & Performance, MEI, UMinho, 2011/12 21 Copyright © 2012, Elsevier Inc. All rights reserved. 22 Families in NVidia GPU NVidia GPU structure & scalability AJProença, Computer Systems & Performance, MEI, UMinho, 2011/12 23 AJProença, Computer Systems & Performance, MEI, UMinho, 2011/12 24 The NVidia Fermi architecture GT200 and Fermi SIMD processor Fermi Multithreaded SIMD Processor (Streaming Fermi Multiprocessor) Architecture AJProença, Computer Systems & Performance, MEI, UMinho, 2011/12 25 AJProença, Computer Systems & Performance, MEI, UMinho, 2011/12 26 Graphical Processing Units Fermi Architecture Innovations Fermi: Multithreading and Memory Hierarchy ! Each SIMD processor has ! Two SIMD thread schedulers, two instruction dispatch units ! 16 SIMD lanes (SIMD width=32, chime=2 cycles), 16 load-store units, 4 special function
Details
-
File Typepdf
-
Upload Time-
-
Content LanguagesEnglish
-
Upload UserAnonymous/Not logged-in
-
File Pages8 Page
-
File Size-