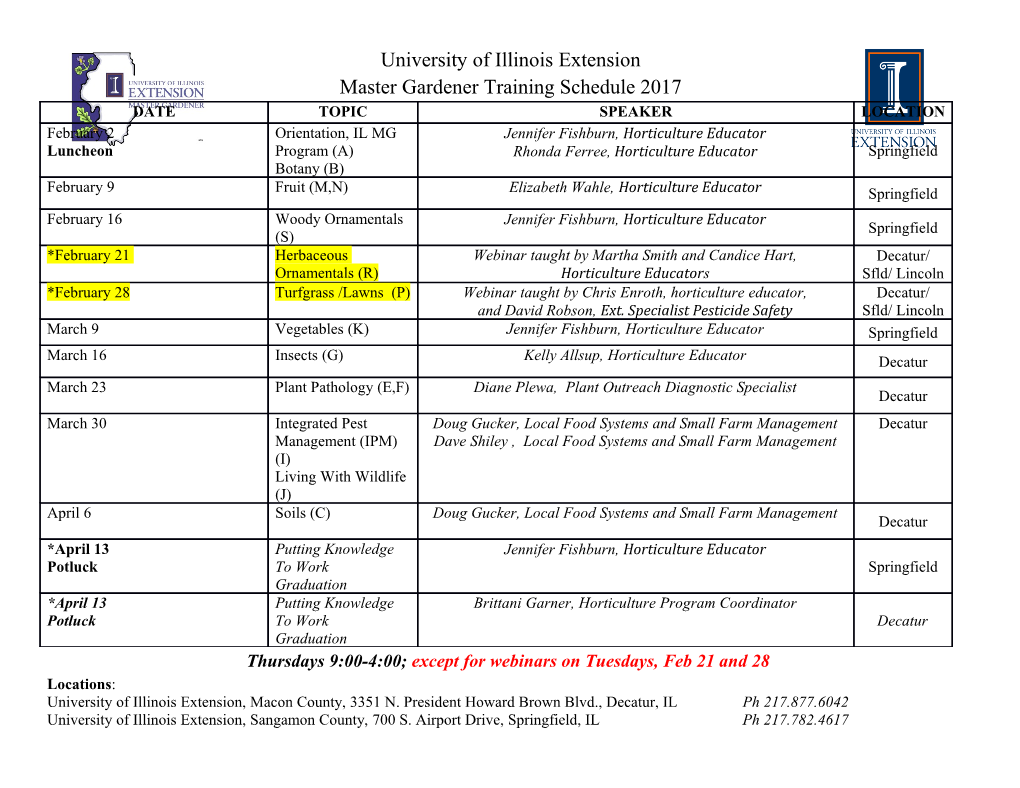
Data Clustering: A Review A.K. JAIN Michigan State University M.N. MURTY Indian Institute of Science AND P.J. FLYNN The Ohio State University Clustering is the unsupervised classification of patterns (observations, data items, or feature vectors) into groups (clusters). The clustering problem has been addressed in many contexts and by researchers in many disciplines; this reflects its broad appeal and usefulness as one of the steps in exploratory data analysis. However, clustering is a difficult problem combinatorially, and differences in assumptions and contexts in different communities has made the transfer of useful generic concepts and methodologies slow to occur. This paper presents an overview of pattern clustering methods from a statistical pattern recognition perspective, with a goal of providing useful advice and references to fundamental concepts accessible to the broad community of clustering practitioners. We present a taxonomy of clustering techniques, and identify cross-cutting themes and recent advances. We also describe some important applications of clustering algorithms such as image segmentation, object recognition, and information retrieval. Categories and Subject Descriptors: I.5.1 [Pattern Recognition]: Models; I.5.3 [Pattern Recognition]: Clustering; I.5.4 [Pattern Recognition]: Applications— Computer vision; H.3.3 [Information Storage and Retrieval]: Information Search and Retrieval—Clustering; I.2.6 [Artificial Intelligence]: Learning—Knowledge acquisition General Terms: Algorithms Additional Key Words and Phrases: Cluster analysis, clustering applications, exploratory data analysis, incremental clustering, similarity indices, unsupervised learning Section 6.1 is based on the chapter “Image Segmentation Using Clustering” by A.K. Jain and P.J. Flynn, Advances in Image Understanding: A Festschrift for Azriel Rosenfeld (K. Bowyer and N. Ahuja, Eds.), 1996 IEEE Computer Society Press, and is used by permission of the IEEE Computer Society. Authors’ addresses: A. Jain, Department of Computer Science, Michigan State University, A714 Wells Hall, East Lansing, MI 48824; M. Murty, Department of Computer Science and Automation, Indian Institute of Science, Bangalore, 560 012, India; P. Flynn, Department of Electrical Engineering, The Ohio State University, Columbus, OH 43210. Permission to make digital/hard copy of part or all of this work for personal or classroom use is granted without fee provided that the copies are not made or distributed for profit or commercial advantage, the copyright notice, the title of the publication, and its date appear, and notice is given that copying is by permission of the ACM, Inc. To copy otherwise, to republish, to post on servers, or to redistribute to lists, requires prior specific permission and/or a fee. © 2000 ACM 0360-0300/99/0900–0001 $5.00 ACM Computing Surveys, Vol. 31, No. 3, September 1999 Data Clustering • 265 CONTENTS Intuitively, patterns within a valid clus- ter are more similar to each other than 1. Introduction 1.1 Motivation they are to a pattern belonging to a 1.2 Components of a Clustering Task different cluster. An example of cluster- 1.3 The User’s Dilemma and the Role of Expertise ing is depicted in Figure 1. The input 1.4 History patterns are shown in Figure 1(a), and 1.5 Outline 2. Definitions and Notation the desired clusters are shown in Figure 3. Pattern Representation, Feature Selection and 1(b). Here, points belonging to the same Extraction cluster are given the same label. The 4. Similarity Measures variety of techniques for representing 5. Clustering Techniques data, measuring proximity (similarity) 5.1 Hierarchical Clustering Algorithms 5.2 Partitional Algorithms between data elements, and grouping 5.3 Mixture-Resolving and Mode-Seeking data elements has produced a rich and Algorithms often confusing assortment of clustering 5.4 Nearest Neighbor Clustering methods. 5.5 Fuzzy Clustering 5.6 Representation of Clusters It is important to understand the dif- 5.7 Artificial Neural Networks for Clustering ference between clustering (unsuper- 5.8 Evolutionary Approaches for Clustering vised classification) and discriminant 5.9 Search-Based Approaches analysis (supervised classification). In 5.10 A Comparison of Techniques supervised classification, we are pro- 5.11 Incorporating Domain Constraints in Clustering vided with a collection of labeled (pre- 5.12 Clustering Large Data Sets classified) patterns; the problem is to 6. Applications label a newly encountered, yet unla- 6.1 Image Segmentation Using Clustering beled, pattern. Typically, the given la- 6.2 Object and Character Recognition 6.3 Information Retrieval beled (training) patterns are used to 6.4 Data Mining learn the descriptions of classes which 7. Summary in turn are used to label a new pattern. In the case of clustering, the problem is to group a given collection of unlabeled patterns into meaningful clusters. In a 1. INTRODUCTION sense, labels are associated with clus- ters also, but these category labels are 1.1 Motivation data driven; that is, they are obtained solely from the data. Data analysis underlies many comput- Clustering is useful in several explor- ing applications, either in a design atory pattern-analysis, grouping, deci- phase or as part of their on-line opera- sion-making, and machine-learning sit- tions. Data analysis procedures can be uations, including data mining, dichotomized as either exploratory or document retrieval, image segmenta- confirmatory, based on the availability tion, and pattern classification. How- of appropriate models for the data ever, in many such problems, there is source, but a key element in both types little prior information (e.g., statistical of procedures (whether for hypothesis models) available about the data, and formation or decision-making) is the the decision-maker must make as few grouping, or classification of measure- assumptions about the data as possible. ments based on either (i) goodness-of-fit It is under these restrictions that clus- to a postulated model, or (ii) natural tering methodology is particularly ap- groupings (clustering) revealed through propriate for the exploration of interre- analysis. Cluster analysis is the organi- lationships among the data points to zation of a collection of patterns (usual- make an assessment (perhaps prelimi- ly represented as a vector of measure- nary) of their structure. ments, or a point in a multidimensional The term “clustering” is used in sev- space) into clusters based on similarity. eral research communities to describe ACM Computing Surveys, Vol. 31, No. 3, September 1999 266 • A. Jain et al. YY x 4 4 x x x 4 4 5 x 5 x x xxx 4 4 45 5 x x 3 5 5 x x x x x 3 3 4 3 x x 4 4 x 4 x 4 x x 4 4 x x x 44 x xx x6 2 2 7 x x x x 2 2 6 7 x x 6 7 x 1 xxx x x 111 6 7 x 1 XX (a) (b) Figure 1. Data clustering. methods for grouping of unlabeled data. sionals (who should view it as an acces- These communities have different ter- sible introduction to a mature field that minologies and assumptions for the is making important contributions to components of the clustering process computing application areas). and the contexts in which clustering is used. Thus, we face a dilemma regard- 1.2 Components of a Clustering Task ing the scope of this survey. The produc- tion of a truly comprehensive survey Typical pattern clustering activity in- would be a monumental task given the volves the following steps [Jain and sheer mass of literature in this area. Dubes 1988]: The accessibility of the survey might (1) pattern representation (optionally also be questionable given the need to including feature extraction and/or reconcile very different vocabularies selection), and assumptions regarding clustering in the various communities. (2) definition of a pattern proximity The goal of this paper is to survey the measure appropriate to the data do- core concepts and techniques in the main, large subset of cluster analysis with its (3) clustering or grouping, roots in statistics and decision theory. Where appropriate, references will be (4) data abstraction (if needed), and made to key concepts and techniques (5) assessment of output (if needed). arising from clustering methodology in the machine-learning and other commu- Figure 2 depicts a typical sequencing of nities. the first three of these steps, including The audience for this paper includes a feedback path where the grouping practitioners in the pattern recognition process output could affect subsequent and image analysis communities (who feature extraction and similarity com- should view it as a summarization of putations. current practice), practitioners in the Pattern representation refers to the machine-learning communities (who number of classes, the number of avail- should view it as a snapshot of a closely able patterns, and the number, type, related field with a rich history of well- and scale of the features available to the understood techniques), and the clustering algorithm. Some of this infor- broader audience of scientific profes- mation may not be controllable by the ACM Computing Surveys, Vol. 31, No. 3, September 1999 Data Clustering • 267 Feature Patterns Pattern Interpattern Clusters Selection/ Similarity Grouping Extraction Representations feedback loop Figure 2. Stages in clustering. practitioner. Feature selection is the Data abstraction is the process of ex- process of identifying the most effective tracting a simple and compact represen- subset of the original features to use in tation of a data set. Here, simplicity is clustering. Feature extraction is the use either from the perspective of automatic of one or more transformations of the analysis (so that a machine can perform input features to produce new salient further processing efficiently) or it is features. Either or both of these tech- human-oriented (so that the representa- niques can be used to obtain an appro- tion obtained is easy to comprehend and priate set of features to use in cluster- intuitively appealing).
Details
-
File Typepdf
-
Upload Time-
-
Content LanguagesEnglish
-
Upload UserAnonymous/Not logged-in
-
File Pages60 Page
-
File Size-