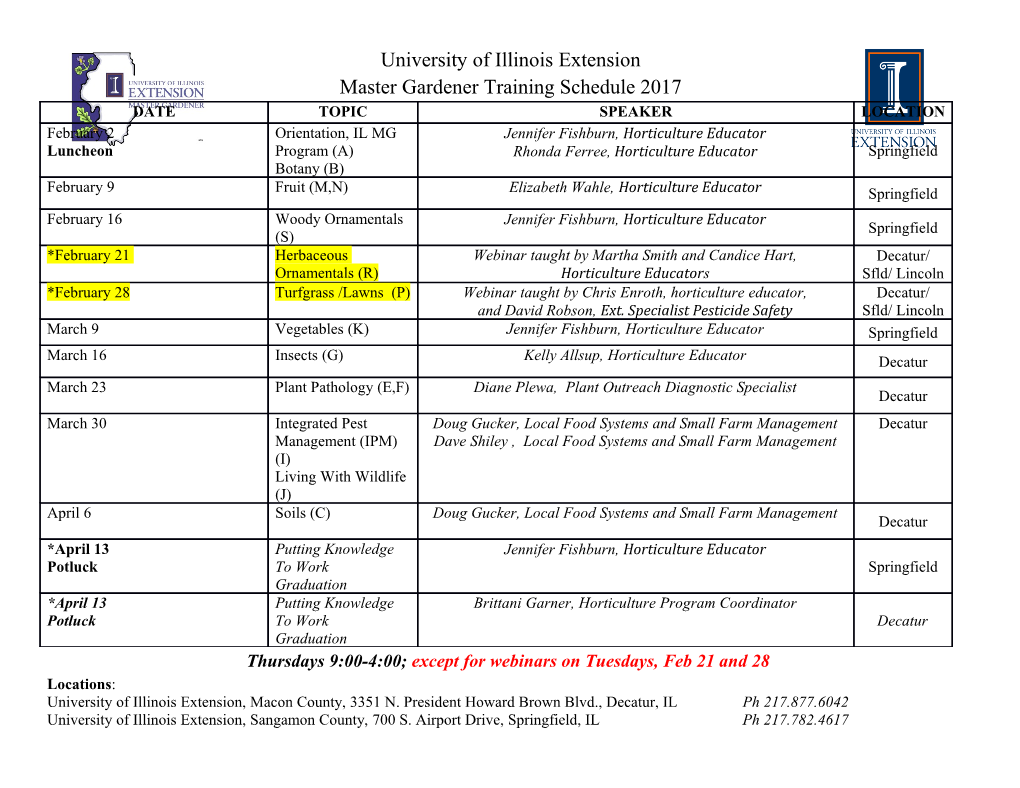
1 1 Hilbert’s Program Revisited Volume I Base case: -computation 휔 Dr Stephen Gaito PerceptiSys Ltd April 7, 2019 Perish then Publish Press. 1 1 2 2 This document was prepared using ConTEXt and LuaTEX on April 7, 2019. Unless explicitly stated otherwise, all content contained in this document which is not software code is Copyright © 2019 PerceptiSys Ltd (Stephen Gaito) and is licensed for release under the Creative Commons Attribution-ShareAlike 4.0 International License (CC-BY-SA 4.0 License). You may obtain a copy of the License at http://creativecommons.org/licenses/by-sa/4.0/ Unless required by applicable law or agreed to in writing, all non-code content distributed under the above CC-BY-SA 4.0 License is distributed on an ‘AS IS’ BASIS, WITHOUT WARRANTIES OR CONDITIONS OF ANY KIND, either express or implied. See the CC-BY-SA 4.0 License whose URL is listed above for the specific language governing permissions and limitations under the License. Again, unless explicitly stated otherwise, all content contained in this document which is software code is Copyright © 2019 PerceptiSys Ltd (Stephen Gaito) and is licensed under the Apache License, Version 2.0 (the "License"); you may not use this code except in compliance with the License. You may obtain a copy of the License at http://www.apache.org/licenses/LICENSE-2.0 Unless required by applicable law or agreed to in writing, software distributed un- der the Apache 4.0 License is distributed on an ‘AS IS’ BASIS, WITHOUT WAR- RANTIES OR CONDITIONS OF ANY KIND, either express or implied. See the Apache 2.0 License whose URL is listed above for the specific language governing permissions and limitations under the License. 2 2 3 3 Wee, sleekit, cowrin, tim’rous beastie, O, what a panic’s in thy breastie! Thou need na start awa sae hasty Wi bickering brattle! I wad be laith to rin an’ chase thee, Wi’ murdering pattle. (Robert Burns’ “To a Mouse” November 1785) Question: What Physics could a wee beastie write down, if a wee beastie could write down Physics? Answer: Quantum Relativity. This cycle of papers is dedicated to all beasties great and small who have given their lives so that one species can know itself. 3 Hilbert’s Program 3 3 4 4 Hilbert’s Program 4 4 4 5 5 Preface Preface Despite the wealth of fruitful work inspired by Hilbert’s Program, it is generally assumed that Gödel’s two Incompleteness Theorems show that Hilbert’s Program has failed. The purpose of this document is to show that while Hilbert’s logical Program is doomed to failure, a computational version of Hilbert’s Program is very definitely capable of providing a neo-platonist foundation of mathematics. The important distinction here is that Gödel’s theorems show that the set of ‘True’ statements of a given consistent formal theory which is at least as powerful as Peano Arithmetic, is a recursively enumerable set which is not recursive. This is what makes Hilbert’s logical Program fail. In this analysis, one of the two key requirements is that the formal theory is consistent. That is you can never ‘prove’ both a statement and its negation. It is this assumption of consistency which forces the set of ‘True’ statements to be recursively enumerable but not recursive. By working computationally, we can, with an assumption equivalent to the Axiom of Choice, build recursive sets which can provide interpretations of standard Zermelo–Fraenkel set theory (with the Axiom of Choice). For each ordinal, , we can define -computation. Standard computation is then -computation. Using a 1 computationally natural form of Vopěnka’s Principle into which we can then휆 build an interpretation휆 of Zermelo–Fraenkel set theory (ZFC), hence휔 showing that ZFC is (computationally) consistent. By taking Cantor’s Absolutely infinite multiplicities (AIMs)2 seriously, we can do a significant amount of classical analysis even with -computational resources. For a given amount of computational resources, we build a dual pair of structures 3 which correspond to well-founded sets and non-well-founded휔 proper classes, or in terms of the terminology of Computer Science, data and processes. Categorically these dual structures are a dual Topos / Co-Topos pair. While the classical Reals, are located in the well-founded sets (Topos) of ZFC and hence obey classical logic, the -computational Reals are processes (Co-Topos) and obey a weaker process logic. The important realization here is that the ‘strangeness’ of Quantum Me- chanics휔 comes from trying to understand the essentially process nature of Quantum Mechanics within the logic of classical sets. We assert that the natural outcome of developing the ‘logic’ of finite process structures is Quantum Relativity. Unfortu- nately, this is a topic for a subsequent document. In this volume, we will concentrate on the base case of -computation. Sub- sequent volumes will look at how to define the transfinite ordinals and the corre- sponding -computation for . With -computation, we휔 can then show that ZFC has a model and hence is computationally consistent. 휆 휔 ≤ 휆 휆 1 See Adámek and Rosiký’s Locally Presentable and Accessible Categories, we can build recursive sets of the size of a Vopěnka cardinal, [AR94]. 2 See Cantor’s letter to Dedekind dated 1899, [Hei67b]. 3 See Aczel’s Non-Well-Founded Sets,[Acz88], or Barwise and Moss’ Vicious Circles,[BM96]. 5 Hilbert’s Program 5 5 6 6 TODO: make the recursively-enumerable/partially-recursive versus recursive/totally-recursive terminology more uniform. Hilbert’s Program 6 6 6 7 7 Contents Contents Preface 5 Contents 7 1 Theory 9 1.1 Introduction 11 1.1.1 Goals 11 1.1.2 Some Philosophy 12 1.1.2.1 Hume’s problem 12 1.1.2.2 What is a thing? 12 1.1.2.3 A Neuron’s eye view 14 1.1.2.4 Mappae Mundi 15 1.1.2.5 Hilbert’s program 15 1.1.2.6 Identity 17 1.1.3 Some Mathematics 17 1.1.3.1 A tale of two foundations 17 1.1.3.2 What does a Mathematician do? 18 1.1.3.3 Cast of thousands 20 1.1.3.4 A Beastie’s environment 21 1.1.3.5 Barr’s ladder 23 1.1.3.6 Lawvere’s Theorem 26 1.1.3.A First section appendix 26 1.1.3.A.1 A test subsubsection 26 1.1.3.B Second section appendix 26 1.1.3.C Third section appendix 27 1.1.4 Some Computer Science 27 1.1.4.1 Extensional and Intensional Objects 27 1.1.5 Some Software Engineering 27 1.1.5.1 ’s Software Architecture 27 1.1.5.2 ’s Complexity Layers 28 1.1.5.3 홹횘횢홻횘홻’s processing components 31 1.1.6 Strategy홹횘횢홻횘홻 32 홹횘횢홻횘홻 7 Hilbert’s Program 7 7 8 8 1.2 Of Wee Beasties and Idealized Mathematicians 35 1.2.1 A wee beastie’s reality, the interpreter 35 1.2.2 What can a ‘wee beastie’ do? 36 1.2.3 What can a ‘wee beastie’ know?홹횘횢홻횘홻 39 1.2.4 Identity and Bisimulation 40 1.2.5 Rigour 41 1.2.A Texts: transcribing Lists of Lists 42 1.2.A.1 Characters 42 1.2.A.2 Symbols 44 1.2.A.3 Texts 45 1.2.A.4 Parsing 45 1.2.B Containers 45 1.2.B.1 Lists 45 1.2.B.2 Stacks 45 1.2.B.3 Dictionaries 46 1.2.B.4 Sets and Power Sets 46 1.3 Computational examples 47 1.3.1 Tree of binary processes 47 1.3.2 Power multi-set 47 1.3.3 Coalgebra 47 1.3.4 Co-Lawvere Theorem 47 1.4 Formal System 49 1.4.1 Mappae Mundi 50 1.4.2 Semantic Functor 53 1.4.3 Syntaxtic Functor 54 2 Implementing in 55 2.1 Abstract Syntax Trees 57 홹횘횢홻횘홻 홹횘횢홻횘홻 2.2 Parser 59 2.3 Type inferencer 61 2.4 Verifier 63 2.5 Interpreter 65 Bibliography 67 Glossary 71 Symbols 73 Subject Index 75 Hilbert’s Program 8 8 8 9 9 1 Theory 9 Hilbert’s Program 9 9 10 10 Hilbert’s Program 10 10 10 11 11 1.1 Introduction 1.1 Introduction 1.1.1 Goals Every long term researcher should have a silly question, if only to keep them focused upon whole problems amidst all of the unending detail. Your ultimate destination, whether or not you get there, influences how you plan to get there. The problem for any researcher, is that the research ‘space’ is infinite dimensional. From the dazzle of choices, you must make ‘one’ sequence of choices which, one hopes, ultimately leads to your answer(s). The vantage point provided by your ultimate goal, influences the questions asked and hence the answers found. The choice of where you want to get to, does have a profound influence on how you choose to get there. My silly question is: Why do babies babble? There are (at least) two aspects to this question: 1. How do organic naive ‘brains’ build models of reality. Too much of classical arti- ficial intelligence focuses on the incremental learning based upon the capabilities of ‘adult’ learners. However this misses the point of learning models of Reality ab initio. 2. Equally important, is the question of what constitutes an efficient model. Animal brains must keenly balance energy use with the comprehensiveness of a given model. Any animal that gets this wrong too much of the time, becomes someone else’s lunch.
Details
-
File Typepdf
-
Upload Time-
-
Content LanguagesEnglish
-
Upload UserAnonymous/Not logged-in
-
File Pages76 Page
-
File Size-