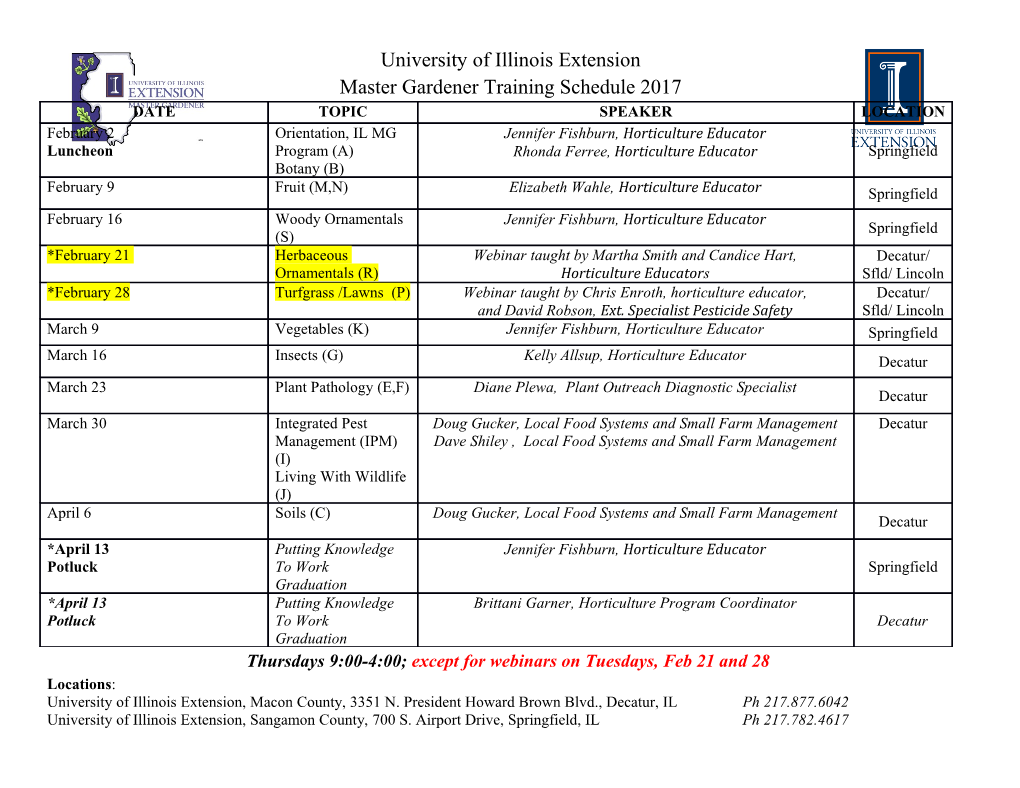
Engage the Public: Poll Question Generation for Social Media Posts Zexin Lu1 Keyang Ding1 Yuji Zhang1 Jing Li1∗ Baolin Peng2 Lemao Liu3 1Department of Computing, The Hong Kong Polytechnic University, HKSAR, China 2Microsoft Research, Redmond, WA 3Tencent AI Lab, Shenzhen, China 1fzexin.lu, keyang.ding, [email protected] [email protected] [email protected] [email protected] Abstract [P1]: ...B站市<超Ç1Gz (The market value of B site exceeds iQiyi)... This paper presents a novel task to generate [Q1]: `们s时8(£*app看Æ频? (Which app do poll questions for social media posts. It offers you usually use to watch videos?) [A ] ~¯Æ频 Tencent Video 优w Youku 1Gz an easy way to hear the voice from the pub- 1 : ( ); ( ); (iQiyi); B站 (B site) lic and learn from their feelings to important [P2]: ...理 ' 分 析 一 下 u ¤ 和 希 林 娜 依 高 : 希 social topics. While most related work tack- 林vocaln实厉³,F/...舞H实力有¹不够看;u les formally-written texts (e.g., exam papers), ¤b舞H厉³...F/1L实力较1些... (A rational we generate poll questions for short and collo- analysis of Akira and Curley G: Curley’s vocal is indeed quial social media messages exhibiting severe great, but ... her dancing is not that good; Akira dances data sparsity. To deal with that, we propose to well ... but her singing is weaker...) 谁更适合S M? encode user comments and discover latent top- [Q2]: c (Who should take the center position?) ics therein as contexts. They are then incorpo- [A2]: u¤ (Akira); 希林娜依高 (Curley G) rated into a sequence-to-sequence (S2S) archi- tecture for question generation and its exten- Figure 1: Example polls from Sina Weibo. Pi, Qi, and sion with dual decoders to additionally yield Ai (i = 1; 2) refer to the i-th source post, its poll ques- poll choices (answers). For experiments, we tion, and the corresponding poll choices (answers). Dif- collect a large-scale Chinese dataset from Sina ferent choices are separated by the “;”. Italic words in Weibo containing over 20K polls. The results “()” are the English translation of the original Chinese show that our model outperforms the popu- texts on their left. In the source posts, we fold the words lar S2S models without exploiting topics from irrelevant to polls in “...” for easy reading. comments and the dual decoder design can fur- ther benefit the prediction of both questions input their reflections via voting. For example, the and answers. Human evaluations further ex- statistics of our dataset show that 13K users on hibit our superiority in yielding high-quality average engaged in a poll compared with 173 com- polls helpful to draw user engagements. mented to a post. For a better illustration of the 1 Introduction task, Figure1 shows two example poll questions on Sina Weibo1, henceforth Weibo, a popular Chinese Social media is a crucial outlet for people to ex- microblog. The goal of our task is to output an change ideas, share viewpoints, and keep con- opinion question, such as Q1 and Q2, and invite nected with the world. It allows us to hear the other users to engage in the discussion to a source public voice for decision making and better under- post (e.g., P1 and P2); poll choices (answers like standing our society. Nevertheless, for the silent A1 and A2) can be produced together to allow easy majority, they tend to read others’ messages instead public engagement (via voting). of voicing their own opinions with words, possibly To date, most progress made in question gener- because of the introvert personality, busy schedule, ation is built upon the success of encoder-decoder and others. How shall we better engage them into frameworks (Du et al., 2017). Despite of the ex- the discussions and learn from their thoughts? tensive efforts made in this line (Sun et al., 2018; In this work, we present a novel application to Yao et al., 2018; Chai and Wan, 2020; Sun et al., automatically generate a poll question for a social 2020), most previous work focus on the processing media post. It will encourage public users, espe- of formally-written texts, such as exam questions cially those reluctant to comment with words, to ∗Jing Li is the corresponding author. 1weibo.com 29 Proceedings of the 59th Annual Meeting of the Association for Computational Linguistics and the 11th International Joint Conference on Natural Language Processing, pages 29–40 August 1–6, 2021. ©2021 Association for Computational Linguistics in reading comprehension tests. The existing meth- automatic evaluation results show that the latent ods are therefore suboptimal to handle social media topics learned from the first few pieces of user com- languages with short nature and informal styles, ments is already helpful — they result in our mod- which might present challenges to make sense of els’ significantly better performance than the S2S the source posts and decide what to ask. For ex- baselines and their trendy extensions proposed for ample, from the limited words in P1, it is hard to other tasks. For example, our full model achieves capture the meanings of “B站”(B site) and “1G 38.24 ROUGE-1 while S2S with RoBERTa (Liu z”(iQiyi) as video apps, which is nevertheless et al., 2019) yields 34.08. Human evaluation fur- crucial to predict Q1. Moreover, the question itself, ther demonstrates our models’ capability to gener- being in social media fashion, is likely to contain ate poll questions relevant to the source post, fluent fresh words, such as “cM”(center position) in Q2, in language, and particularly engaging to draw user which may further hinder the models’ capability to attentions for discussions. We then quantify mod- predict the poll questions in social media style. els’ sensitivities to the length of varying source To tackle these challenges, we first enrich the posts and poll questions, where the scores of our short contexts of source posts with other users’ model are consistently better. Next, we find our comments; a neural topic model is employed to model exhibits an increasing trend in predicting discover topic words therein and help identify the poll questions that will engage more comments in key points made in source posts. It is based on the future, which suggests the potential helpfulness the assumption that the salient words in a source of comments to indicate engaging questions. At post are likely to be echoed in its comments (Wang last, the performance of dual decoder designs are et al., 2019b), potentially useful to learn the map discussed and it is shown that joint prediction of from posts to poll questions. For example, the questions and their answers can benefit both tasks. core words in Q — “app” and “Æ频”(video)— 1 2 Study Design co-occur frequently in the comments with “B站” (B site) and “1Gz”(iQiyi), which may help 2.1 Task Formulation the model to link their meanings together. The Our major input is a social media post (i.e., source topic representations are then incorporated into a post) and the main output a poll question that con- sequence-to-sequence (S2S) architecture to decode tinue the senses of the source post and encourage poll questions word by word. Furthermore, we ex- public users to voice opinions. For each question, tend the basic S2S to a version with dual decoders possible answer choices (i.e., answers) may also be to generate questions and answers in a multi-task yielded as a side product to enable participants to learning setting and further exploit their correla- easily input their thoughts. To enrich the contexts tions. For example, modeling answers in A2 might of source posts, their reply messages (i.e., user u¤ help indicate that P2 centers around “ ”(Akira) comments) are also encoded as external features. and “希林娜依高”(Curley G), two celebrities. To the best of our knowledge, this work is the 2.2 Data Description first to study poll questions on social media, where Here we describe the dataset we collect to empiri- their interactions among answer choices, source cally study social media polls. posts, and reader users’ comments are comprehen- sively explored. As a pilot study over social media Data Collection. Weibo allows users to create polls, we also contribute the very first dataset con- polls, asking questions to the public and inviting taining around 20K Weibo polls associated with others to share their thoughts via voting. It enables their source posts and user comments.2 We believe the construction of a dataset with user-generated our dataset, being the first of its kind, will largely polls. At the beginning, we gathered around 100K benefit the research on social media polls and how random Weibo posts, whereas less than 0.1% of they help promote the public engagements. them contain polls. The sparse distribution of polls presents the challenge to scale up the dataset. To On our dataset, we first compare the model per- deal with that, we looked in to the sampled polls formance on poll question generation in terms of and draw two interesting points: first, many polls automatic evaluation and human evaluation. The carry trendy hashtags (user-annotated topic labels 2Our dataset and code are publicly available in like #COVID19) to draw user attentions; second, a https://github.com/polyusmart/Poll-Question-Generation user who once created a poll is likely to do it again. 30 Post Comment Qs Ans Choice Voter Num Len Num Len Len Num Len Num 20,252 54.0 173 16.9 11.0 3.4 5.9 13,004 Table 1: Statistics of our dataset. Num: number; Num: average number per post. Len: average count of words per post; Qs: question; Ans: answer.
Details
-
File Typepdf
-
Upload Time-
-
Content LanguagesEnglish
-
Upload UserAnonymous/Not logged-in
-
File Pages12 Page
-
File Size-