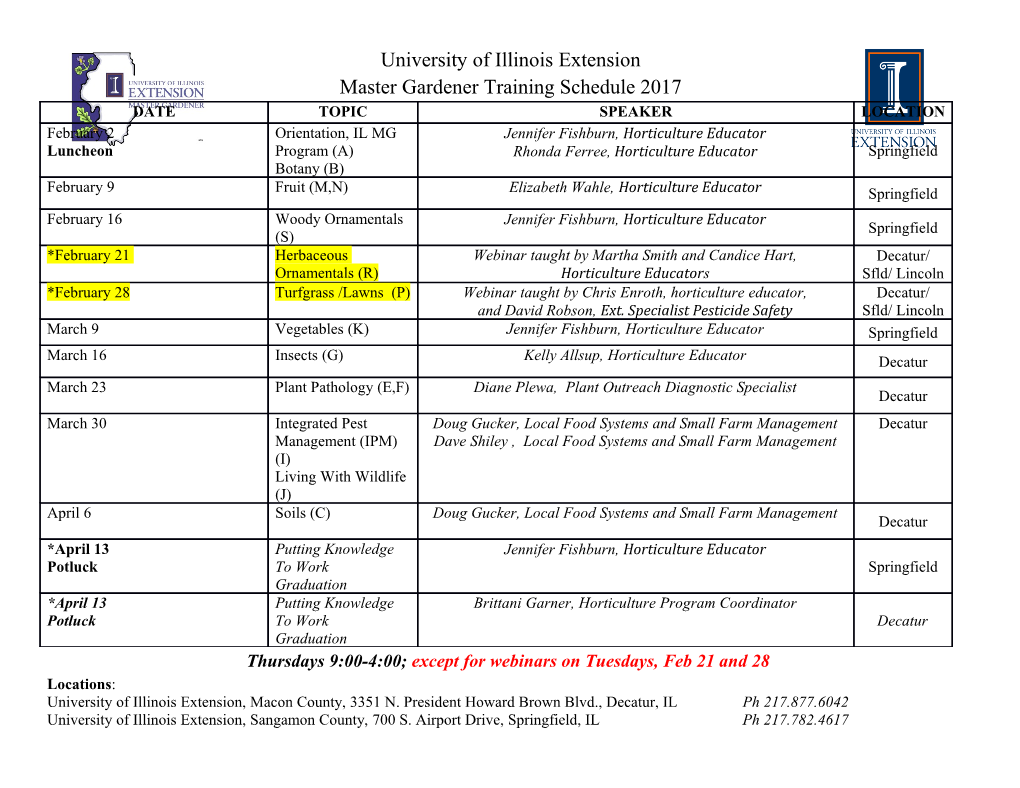
TESTING THE BAYESIAN BRAIN HYPOTHESIS IN VISUAL PERCEPTION A Dissertation presented to the Faculty of the Graduate School at the University of Missouri In Partial Fulfillment of the Requirements for the Degree Doctor of Philosophy by APRIL SWAGMAN Dr. Jeffrey N. Rouder, Dissertation Supervisor JULY 2016 The undersigned, appointed by the Dean of the Graduate School, have examined the dissertation entitled: TESTING THE BAYESIAN BRAIN HYPOTHESIS IN VISUAL PERCEPTION presented by April Swagman, a candidate for the degree of Doctor of Philosophy and hereby certify that, in their opinion, it is worthy of acceptance. Dr. Jeffrey N. Rouder Dr. Clint Davis-Stober Dr. Chi-Ren Shyu Dr. Jeffrey Johnson ACKNOWLEDGMENTS For providing the inspiration for this project and my first experiment, I would like to acknowledge Dr. Wei Ji Ma at New York University, who was sure we would find evidence that people are ideally Bayesian. To all past and present members of the Perception and Cognition Lab{Jon Thiele, Julia Haaf, Jory Province, and Tim Ricker{thank you for the encouragement and friendship you provided as I worked on this project. I would especially like to thank Jory for being a mentor and friend throughout my learning experience in the Rouder lab, and for the significant theoret- ical contributions he made to this work. I would like to thank all the PCL undergrad- uate research assistants who helped out with data collection, and especially to Ryan Bahr who helped me become a more effective mentor and was even willing to learn R along the way. And of course I am eternally grateful to my adviser Jeff Rouder for being helpful, inspiring, and, most importantly, patient as I made my way through this work. Finally I would like to acknowledge my parents and brothers for supporting me through my time at Missouri even though they never understood what I was actually working on. And to Nick{my partner, my best friend, my reason for caring{thank you for believing in me and trusting that I knew what I was doing, even when I so clearly didn't. ii TABLE OF CONTENTS ACKNOWLEDGMENTS . ii LIST OF TABLES . vii LIST OF FIGURES . viii ABSTRACT . xvii CHAPTER 1 Introduction and Review . 1 1.1 Bayesian Ideal Observer Theory . 2 1.2 Support for Bayesian Ideal Behavior . 4 1.3 Evidence against Bayesian Ideal Behavior . 6 1.4 Motivation for Project . 9 2 Innovations in Method and Modeling . 12 2.1 Methodological Solutions and Innovations . 12 2.1.1 Individual Analysis . 12 2.1.2 Production Paradigm . 13 2.1.3 No-Information Condition . 14 2.1.4 Controlled Bayesian Elements and Sensory Manipulation . 15 2.1.5 Consideration of Alternative Models . 16 2.2 Model Construction . 17 2.2.1 Bayesian Ideal Observer Model . 18 iii 2.2.2 Posterior Matching Model . 22 2.2.3 Discrete-State Mixture Model . 25 3 Perception and Judgment of Ellipse Orientation . 27 3.1 Introduction of Experiments 1A, 1B, and 1C . 27 3.2 Method . 29 3.2.1 Participants . 29 3.2.2 Design . 29 3.2.3 Stimuli . 29 3.2.4 Procedure . 30 3.3 Models . 32 3.3.1 Bayesian Ideal Observer Model . 32 3.3.2 Posterior Matching Model . 35 3.3.3 Discrete-State Mixture Model . 38 3.4 Results . 41 3.4.1 Model Fits . 47 3.5 Conclusions . 57 4 Inferring Center of Mass from a Subset of Items . 60 4.1 Introduction of Experiment 2 . 60 4.2 Method . 62 4.2.1 Participants . 62 4.2.2 Design . 62 4.2.3 Stimuli . 62 iv 4.2.4 Procedure . 63 4.3 Models . 65 4.3.1 Bayesian Ideal Observer Model . 66 4.3.2 Posterior Matching Model . 69 4.3.3 Non-Bayesian Models . 73 4.4 Results . 75 4.4.1 Model Fits . 78 4.5 Conclusions . 86 5 Estimating the Number of Items in a Briefly-Presented Array . 92 5.1 Introduction of Experiment 3 . 92 5.2 Method . 94 5.2.1 Participants . 94 5.2.2 Design . 94 5.2.3 Stimuli . 94 5.2.4 Procedure . 95 5.3 Models . 97 5.3.1 Bayesian Ideal Observer Model . 98 5.3.2 Posterior Matching Model . 99 5.3.3 Discrete-State Mixture Model . 102 5.4 Results . 105 5.4.1 Model Fits . 108 5.5 Conclusions . 115 v 6 Summary and Concluding Remarks . 118 6.1 Summary of Experimental Results . 120 6.1.1 Experiment 1 . 120 6.1.2 Experiment 2 . 121 6.1.3 Experiment 3 . 122 6.2 Discussion of Results . 123 6.3 Critiques and Shortcomings . 124 6.4 Future Directions . 126 Bibliography . 128 VITA . 135 vi LIST OF TABLES Table Page 3.1 AIC Difference Scores, Exp. 1A . 51 3.2 BIC Difference Scores, Exp. 1A . 51 3.3 AIC Differences Scores, Exp. 1B and 1C . 54 3.4 BIC Differences Scores, Exp. 1B and 1C . 54 4.1 AIC Differences Scores, Exp. 2 . 84 4.2 BIC Difference Scores, Exp. 2 . 85 5.1 AIC Differences Scores, Exp. 3 . 111 5.2 BIC Difference Scores, Exp. 3 . 111 vii LIST OF FIGURES Figure Page 2.1 Trial sequence for Experiment 1. Participants briefly saw an ellipse with a given orientation. They produced their response about the ellipse's orientation by moving a dot along an arc and clicking. Partic- ipants then received feedback and points for their response. 13 2.2 The four ellipse eccentricity conditions of Experiment 1 from easiest to most difficult. In Condition 4, the ellipse was actually a circle. 14 2.3 Left panel: Model predictions for the mean of participant responses across the four conditions in Experiment 1A. The mean predictions are the same for the BIO model and the PM model. Right panel: Predictions for the variance of participant responses as sensory uncer- tainty increases, separated by model. The red solid line is from the PM model and the dotted blue line is from the BIO model. 22 3.1 The four ellipse eccentricity conditions of Experiment 1 from easiest to most difficult. In Condition 4, the ellipse was actually a circle. Note the ellipses were drawn in white on a black background in the actual experiment. 30 viii 3.2 Trial sequence for Experiment 1. Participants briefly saw an ellipse with a given orientation. They then produced their response about the ellipse's orientation by moving a dot along an arc and clicking. Participants then received feedback about the correct orientation and points for their response. 31 3.3 Predictions from the BIO model in Equation 3.1 for one participant's responses in each condition of Experiment 1A. The presented angle (in degrees) is on the x-axis, and the participant's response is on the y-axis. A dashed line is drawn at 0 on the y-axis to indicate the mean of the prior distribution. 34 3.4 Predictions from the Posterior Matching model for one participant's responses in each condition of Experiment 1A. The presented angle (in degrees) is on the x-axis, and the participant's response is on the y-axis. A dashed line is drawn at 0 on the y-axis to indicate the mean of the prior distribution. 37 3.5 Predictions from the Discrete State model for one hypothetical par- ticipant in each condition of Experiment 1. The presented angle (in degrees) is on the x-axis, and the participant's response is on the y- axis. A dashed line is drawn at 10 on the y-axis to indicate the mean of this participant's guessing distribution. The parameter values used to plot this image are σD = 3; σG = 20; µG = 10; p1 = :95; p2 = :8; p3 = :6: 40 3.6 Aggregated responses for 29 participants in each of four conditions of Experiment 1A. The dotted diagonal line represents perfect accuracy and the dashed horizontal line at zero shows the prior mean. 42 ix 3.7 Responses across Condition 4 trials for four select participants. The participant's response in degrees is on the y-axis and the trial number (from 1 to 75) is on the x-axis. Participants 22 and 6 show the most ideal response patterns. Participant 14 shows evidence of learning the prior over time. Participant 16 shows an especially variable response pattern captured best by the PM model. 43 3.8 Aggregated responses for all 15 participants in each of four conditions from Experiment 1B. The dotted diagonal line represents perfect ac- curacy and the dashed horizontal line at -20 shows the prior mean. 45 3.9 Aggregated responses for all 15 participants in each of four conditions from Experiment 1C. The dotted diagonal line represents perfect ac- curacy and the dashed horizontal line at 20 shows the prior mean. 46 3.10 BIO and PM parameter estimates for each participant in Experiment 1A. Each Sigma corresponds to the standard deviation of that condi- tion, except for Sigma T which is the estimated motor noise term across conditions. The dashed lines connect a single participant's estimates. 48 3.11 DS parameter estimates for each participant in Experiment 1A. The left panel shows the pj parameters for each condition. The right panel shows the µG, σD, and σG parameters. The dashed lines connect a single participant's estimates. 49 3.12 µG parameter estimates from the Discrete-State model for Experiments 1B (left panel) and 1C (right panel). The dotted lines at −20◦ and 20◦ indicate the prior means in the corresponding experiment. 52 x 3.13 Heat map plot of AIC weights for each model and participant in Ex- periments 1A, 1B, and 1C.
Details
-
File Typepdf
-
Upload Time-
-
Content LanguagesEnglish
-
Upload UserAnonymous/Not logged-in
-
File Pages153 Page
-
File Size-