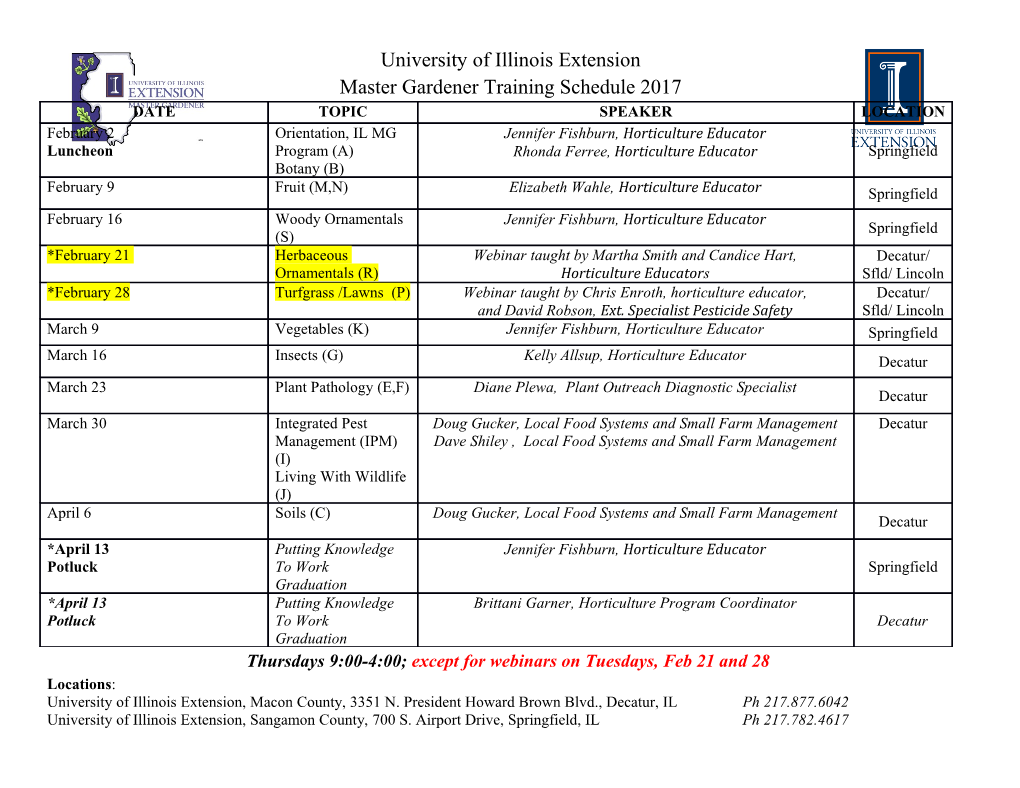
Geometry of Quantum States Ingemar Bengtsson and Karol Zyczk_ owski An Introduction to Quantum Entanglement 12 Density matrices and entropies A given object of study cannot always be assigned a unique value, its \entropy". It may have many different entropies, each one worthwhile. |Harold Grad In quantum mechanics, the von Neumann entropy S(ρ) = Trρ ln ρ (12.1) − plays a role analogous to that played by the Shannon entropy in classical prob- ability theory. They are both functionals of the state, they are both monotone under a relevant kind of mapping, and they can be singled out uniquely by natural requirements. In section 2.2 we recounted the well known anecdote ac- cording to which von Neumann helped to christen Shannon's entropy. Indeed von Neumann's entropy is older than Shannon's, and it reduces to the Shan- non entropy for diagonal density matrices. But in general the von Neumann entropy is a subtler object than its classical counterpart. So is the quantum relative entropy, that depends on two density matrices that perhaps cannot be diagonalized at the same time. Quantum theory is a non{commutative proba- bility theory. Nevertheless, as a rule of thumb we can pass between the classical discrete, classical continuous, and quantum cases by choosing between sums, integrals, and traces. While this rule of thumb has to be used cautiously, it will give us quantum counterparts of most of the concepts introduced in chapter 2, and conversely we can recover chapter 2 by restricting the matrices of this chapter to be diagonal. 12.1 Ordering operators The study of quantum entropy is to a large extent a study in inequalities, and this is where we begin. We will be interested in extending inequalities that are valid for functions defined on R to functions of operators. This is a large morsel. But it is at least straightforward to define operator functions, that is functions of matrices, as long as our matrices can be diagonalized by unitary y y transformations: then, if A = Udiag(λi)U , we set f(A) Udiag f(λi) U , where f is any function on R. Our matrices will be Hermitian≡ and therefore 12.1 Ordering operators 269 they admit a partial order; B A if and only B A is a positive operator. It is a difficult ordering relation to≥ work with though,− ultimately because it does not define a lattice|the set X : X A and X B has no minimum point in general. f ≥ ≥ g With these observations in hand we can define an operator monotone func- tion as a function such that A B f(A) f(B) : (12.2) ≤ ) ≤ Also, an operator convex function is a function such that f(pA + (1 p)B) pf(A) + (1 p)f(B) ; p [0; 1] : (12.3) − ≤ − 2 Finally, an operator concave function f is a function such that f is operator convex. In all three cases it is assumed that the inequalities hold− for all ma- trix sizes (so that an operator monotone function is always monotone in the ordinary sense, but the converse may fail).1 The definitions are simple, but we have entered deep waters, and we will be submerged by difficulties as soon as we evaluate a function at two opera- tors that do not commute with each other. Quite innocent looking monotone functions fail to be operator monotone. An example is f(t) = t2. Moreover the function f(t) = et is neither operator monotone nor operator convex. To get serious results in this subject some advanced mathematics, including frequent excursions into the complex domain, are needed. We will confine ourselves to stating a few facts. Operator monotone functions are characterized by L¨owner's theorem. A function f(t) on an open interval is operator monotone if and only if it can be extended analytically to the upper half plane and transforms the upper half plane into itself. Therefore the following functions are operator monotone: f(t) = tγ ; t 0 if and only if γ [0; 1] ≥ 2 f(t) = at+b ; t = d=c ; ad bc > 0 (12.4) ct+d 6 − − f(t) = ln t ; t > 0 : This small supply can be enlarged by the observation that the composi- tion of two operator monotone functions is again operator monotone; so is f(t) = 1=g(t) if g(t) is operator monotone. The set of all operator mono- tone functions− is convex, as a consequence of the fact that the set of positive operators is a convex cone. A continuous function f mapping [0; ) into itself is operator concave if and only if f is operator monotone. Operator1 convex functions include f(t) = ln t, and f(t) = t ln t when t > 0; we will use the latter function to construct en− tropies. More generally f(t) = tg(t) is operator convex if g(t) is operator monotone. 1 The theory of operator monotone functions was founded by L¨owner (1934) [?]. An interesting early paper is by Bendat and Sherman (1955) [?]. For a survey see Bhatia's book [?], and (for matrix means) Ando (1994) [?]. 270 Density matrices and entropies Finally we define the mean AσB of two operators. We require that AσA = A, as well as homogeneity, α(AσB) = (αA)σ(αB), and monotonicity, AσB CσD if A C and B D. Moreover we require that (T AT y)σ(T BT y) ≥ T (AσB)T y,≥as well as a≥suitable continuity property. It turns out [?] that≥ every mean obeying these demands takes the form 1 1 A σ B = pA f B pA ; (12.5) p p A A where A > 0 and f is an operator monotone function on [0; ) with f(1) = 1. (It is not known how to define a mean of more than two operators.)1 The mean will be symmetric in A and B if and only if f is self inversive, that is if and only if f(1=t) = f(t)=t : (12.6) Special cases of symmetric means include the arithmetic mean for f(t) = (1 + t)=2, the geometric mean for f(t) = pt, and the harmonic mean for f(t) = 2t=(1+t). It can be shown that the arithmetic mean is maximal among symmetric means, while the harmonic mean is minimal [?]. We will find use for these results throughout the next three chapters. But to begin with we will get by with inequalites that apply, not to functions of operators directly but to their traces. The subject of convex trace functions is somewhat more manageable than that of operator convex functions. A key result is that the inequality (1.11) for convex functions can be carried over in this way;2 Klein's inequality. If f is a convex function and A and B are Hermitian operators, then Tr[f(A) f(B)] Tr[(A B)f 0(B)] : (12.7) − ≥ − As a special case Tr(A ln A A ln B) Tr(A B) (12.8) − ≥ − with equality if and only if A = B. To prove this, use the eigenbases: A e = a e B f = b f e f = c : (12.9) j ii ij ii j ii ij ii h ij j i ij A calculation then shows that e f(A) f(B) (A B)f 0(B) e = h ij − − − j ii = f(a ) c 2[f(b ) (a b )f 0(b )] = (12.10) i − j ij j j − i − j j j X = c 2[f(a ) f(b ) (a b )f 0(b )] : j ij j i − j − i − j j j X 2 The original statement here is due to Oskar Klein (1931) [?]. 12.2 Von Neumann entropy 271 This is positive by eq. (1.11). The special case follows if we specialize to f(t) = t ln t. The condition for equality requires some extra attention|it is true. Another useful result is Peierl's inequality. If f is a strictly convex function and A is a Hermitian operator, then Trf(A) f( f A f ) ; (12.11) ≥ h ij j ii i X where f is any complete set of orthonormal vectors, or more generally a fj iig resolution of the identity. Equality holds if and only if fi = ei for all i, where A e = a e . j i j i j ii ij ii To prove this, observe that for any vector f we have j ii f A f = f e 2f(a ) f( f e 2a ) = f( f A f ) : (12.12) h ij j ii jh ij j ij j ≥ jh ij j ij j h ij j ii j j X X Summing over all i gives the result. We quote without proofs two further trace inequalities, the Golden Thomp- son inequality TreAeB TreA+B ; (12.13) ≥ with equality if and only if the Hermitian matrices A and B commute, and its more advanced cousin, the Lieb inequality 1 ln A−ln C+ln B 1 1 Tre Tr A B du ; (12.14) ≥ C + u C + u Z0 where A; B; C are all positive.3 12.2 Von Neumann entropy Now we can begin. First we establish some notation. In chapter 2 we used S to denote the Shannon entropy S(p~) of a probability distribution. Now we use S to denote the von Neumann entropy S(ρ) of a density matrix, but we may want to mention the Shannon entropy too. When there is any risk of confusing these entropies, they are distinguished by their arguments. We will also use S S(ρ ) to denote the von Neumann entropy of a density matrix ρ acting i ≡ i i on the Hilbert space Hi.
Details
-
File Typepdf
-
Upload Time-
-
Content LanguagesEnglish
-
Upload UserAnonymous/Not logged-in
-
File Pages25 Page
-
File Size-