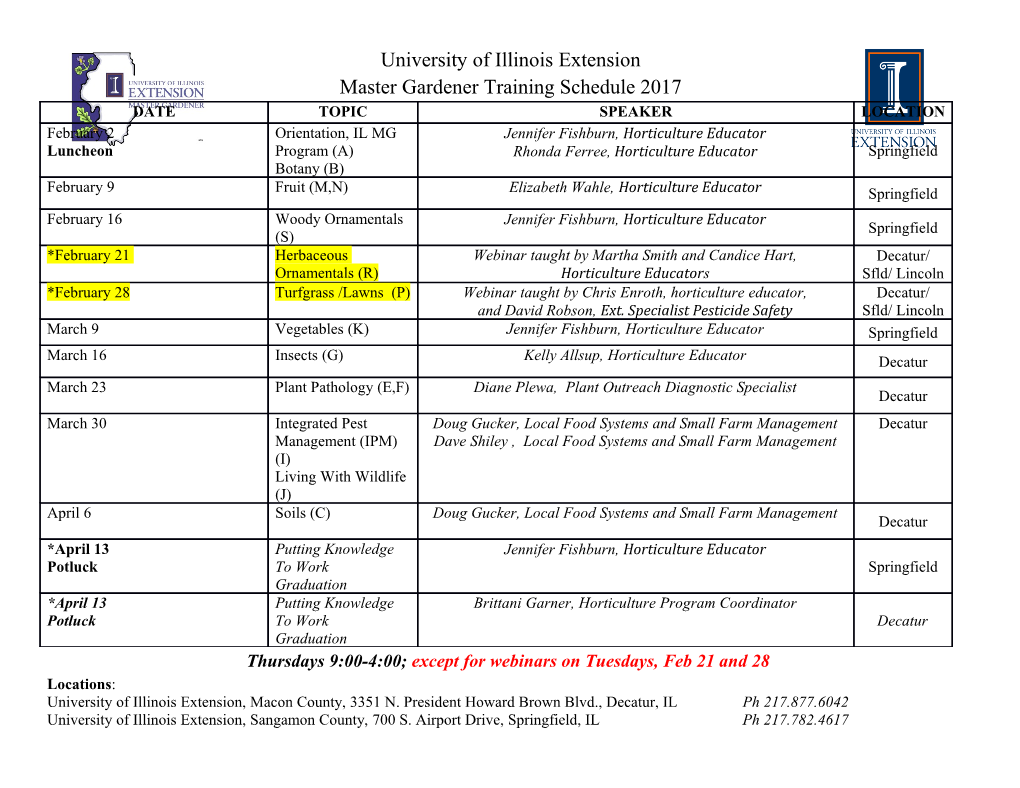
Computational Economics (Algorithmic Game Theory) Xiang-Yang Li, Lan Zhang School of Computer Science and Technology, USTC Professor, CS Department 2021 Graphical Games: Equilibrium and Extensions 2.3 Graphical Games » 2.3.1 Graphical Games • compact representation » 2.3.2 Computing Nash Equilibria • TreeProp • NashProp : a distributed, message-passing algorithm » 2.3.3 Correlated Equilibria • definition and motivation • representation • computation 3 2.3 Graphical Games » 2.3.1 Graphical Games • compact representation » 2.3.2 Computing Nash Equilibria • TreeProp • NashProp : a distributed, message-passing algorithm » 2.3.3 Correlated Equilibria • definition and motivation • representation • computation 4 Multiplayer Games » Large population games with limit players’ interaction. » Game Theory: • provide sound, rigorous mathematical formulation. • limited attention to problem representation: commonly “flat”, large-size representation that don’t exploit “structure”. • graph-based representation introduced to model interaction. » Multiplayer games Algorithms for computing equilibria in large population games with structured interactions Multiplayer Game Example » A multiplayer game consists of 푛 players, each with a finite set of pure strategies or actions available to them, along with a specification of the payoffs to each player. » Example 1: Pollution game • This game is the extension of Prisoner’s Dilemma to the case of many players. Different » Example 2: Tragedy of the commons. geographic locations Multiplayer Game Normal Form » A multiplayer game consists of n players, each with a finite set of pure strategies or actions available to them, along with a specification of the payoffs to each player. » 푎푖 ∈ {0, 1} : the action chosen by player 푖, and the joint action 푎Ԧ ∈ {0, 1}푛 » 푀푖 ∈ [0, 1] : the payoff to player 푖, indexed by the joint action 푎Ԧ. » The actions 0 and 1 are the pure strategies of each player: • a mixed strategy for player 푖 is given by the probability 푝푖 ∈ [0, 1] that the player will play 0 » For any joint mixed strategy, given by a produce distribution 푝Ԧ, we define the expected payoff to player 푖 as : 푀푖 푝Ԧ = 퐸푎~푝Ԧ[푀푖(푎Ԧ)] 7 Issues with Normal Form » The normal form representation of Multiplayer Game with large player population is not good enough. » Complexity: the number of parameters that must be specified grows exponentially with the size of the population. • Assuming 푛 players and 2 actions, as we have here, leads to the need for: 푛 matrices 푀푖(one for each player) each of size 2푛 » Conceptual: the normal form may fail to capture structure that is present in the strategic interaction: • Strong Influence • Weakly Influence 8 Structure in Multiplayer Game » It is assumed that the payoff 푀푖 for player 푖 is a function of all the components 푎푗, (푗 = 1, … , 푛) in the joint action vector 푎Ԧ » However, the payoff for player 푖 may be dependent only on the actions of a subset of player 푁(푖) • Conditional independence payoff assumption » Perhaps: the payoff of each player are determined by the actions of only a small subpopulation. • Strong influence • The environmental pollution of a country is more affected by neighboring countries. 9 Graphical Model » Graphical Model : models of structed probabilistic interaction. • 푛 points represent 푛 players, for which naïve representation is size : 2푛 • representation size mostly a function of the “degree of local interaction” among points or players. » Graph Model allows: • easy interpretation • compact representation • efficient computation in some cases Graphical Game & Graph Model » Graphical Games adopt a simple Graph Model. » A graphical game is described by an undirected graph 퐺: • players ~ vertices » A player or vertex 푖 has payoffs that are entirely specified by the actions of 푖 and those of its neighbor set in 퐺 • Strong Influence along neighbors » Now the payoff to player 푖 is a function only of the actions of 푖 and its neighbors: • Rather than the actions of the entire population • Reduce Complexity. 11 Graphical Games » Graphical games can capture and exploit locality or sparsity of direct influences. » Graphical Games: • borrow representational ideas from graphical models. • intuitive graph interpretation: a player’s payoff is only a function of its neighborhood. • example : geography, networks • analogy to probabilistic graphical model : special structure. Graphical Games Formal Definition » A graphical game is a pair (퐺, ℳ), where 퐺 is an undirected graph over the vertices {1, … , 푛}, and ℳ is a set of 푛 local game matrices. For any joint action 푎Ԧ, the 푖 local game matrix 푀푖 ∈ ℳ specifies the payoff 푀푖(푎 ) for player 푖, which depends only on the actions by the players in 푁(푖). Graphical Games Definition » 퐺 : undirected graph representing the local interaction » 푀 : a set of 푛 local game matrices » Player 푖’s payoff 푀푖 푎Ԧ is only a function of its neighborhood 푁(푖): implies conditional independence payoff assumption. ′ ′ » Local payoff matrix 푀푖 : 푀푖 푎Ԧ = 푀푖 푎Ԧ 푁 푖 » Graphical game complexity: max degree of local interaction 푘 = 푚푎푥푖 푁 푖 ≪ 푛 representation size 푂(푛2푘) (exponential in max degree) Equilibrium Definition » Nash equilibrium(NE): A Nash equilibrium for the game is a mixed strategy 푝Ԧ such that for any player 푖, and for any value ′ 푝푖 ∈ [0, 1] ′ 푀푖 푝Ԧ ≥ 푀푖(푝Ԧ[푖: 푝푖 ]) We say that 푝푖 is a best response to the rest of 푝Ԧ » 휖-Nash equilibrium: An 휖-Nash equilibrium is a mixed strategy ′ 푝Ԧ such for any player 푖, and for any any value 푝푖 ∈ [0, 1] ′ 푀푖 푝Ԧ + 휖 ≥ 푀푖(푝Ԧ[푖: 푝푖 ]) We say that 푝푖 is an ε-best response to the rest of 푝Ԧ 15 2.3 Graphical Games » 2.3.1 Graphical Games • compact representation » 2.3.2 Computing Nash Equilibria • TreeProp • NashProp » 2.3.3 Correlated Equilibria • definition and motivation • representation • computation 16 TreeNash Notation and Concept » TreeNash is a tree algorithm, which runs in polynomial time and provably computes approximations of all equilibria. » Capital letters: 푈, 푉 푎푛푑 푊 to denote player/vertex to distinguish them from their chosen actions/values(lower letter 푢, 푣, 푤). » Local game matrix: 푀푉 to denote the local game matrix for the player/vertex 푉. TreeNash Notation and Concept » If G is a tree, we choose an arbitrary vertex as the root (which we visualize as being at the bottom, with the leaves Leaf/Parent at the top). » Upstream: any vertex on a path from 푉 to any leaf will be called upstream from 푉: downstream upstream • 푈푃퐺 푉 to denote the set of all vertices in 퐺 that are upstream from 푉 » Downstream: any vertex on the path Root/Child from a vertex 푉 to the root will be called downstream from 푉. TreeProp NE » Suppose that 푉 is the child of 푈 in 퐺. » So, we let 퐺푈 denote the subgraph induced by the vertices in 푈푃퐺(푈) the subtree of 퐺 rooted at 푈 » Let: 푣 ∈ [0, 1] is a mixed strategy for 푈 player/vertex 푉: ℳ푉=푣 will be the subset of payoff matrices in ℳ corresponding to the vertices in 푈푃퐺(푈) TreeProp NE » An NE for the graphical game 푈 푈 (퐺 , ℳ푉=푣) is a conditional equilibrium “upstream” from 푈 – that is an equilibrium for 퐺푈 given that 푉 plays 푣. » Here we are simply exploiting the fact that since 퐺 is a tree, fixing a mixed strategy 푣 for the play of 푉 isolates 퐺푈 from the rest of 퐺. TreeProp Downstream: 푇(푣, 푢) » Now suppose that vertex 푉 has 푘 parents 푈1, 푈2, … , 푈푘 and the single child 푊. » The data structures sent from each 푈푖 to 푉, on the downstream pass of TreeNash , and in turn from 푉 to 푊 : • Each parent 푈 will send to 푉 a 푖 푇(푣, 푢푖) binary-valued “table” 푇(푣, 푢푖) TreeProp Downstream: 푇(푣, 푢) » The table is indexed by the continuum of possible values for the mixed strategies 푣 ∈ [0, 1] of 푉 and 푢푖 ∈ [0, 1] of 푈1, 푈2, … , 푈푘 » The semantics of this table: for any pair (푣, 푢 ), 푇(푣, 푢 ) will be 1 if and 푖 푖 푇(푣, 푢푖) only if there exists an NE for 푈 푈푖 푖 (퐺 , ℳ푉=푣) in which 푈1 = 푢푖 TreeProp Downstream: 푇(푤, 푣) » The data structures sent from 푉 to 푊, on the downstream pass of TreeNash » 푇(푤, 푣) = 1 ⟺ there exists a vector of mixed strategies 푢 = (푢1, … , 푢푘) (called a witness) for the parents 푈 = (푈1, … , 푈푘) of 푉 such that: 푇 푣, 푢푖 = 1 for all 1 ≤ 푖 ≤ 푘 푉 = 푣 is a best response to 푈 = 푢, 푊 = 푤. 푇(푤, 푣) TreeProp Downstream » There may be more than one witness for 푇 (푤, 푣) = 1: • 푉 will also keep a list of the witnesses 푢 for each pair (푤, 푣) for which 푇 (푤, 푣) = 1 • These witness lists will be used on the upstream pass. » The downstream pass of the algorithm terminates at the root 푊. TreeProp Upstream » Begin: Root node W choose any witness (푉1, … , 푉푘) to 푇(푤) = 1, and then passing both w and 푣푖 to each parent 푉푖. • The interpretation is that 푊 will play 푤, and is “instructing” 푉푖 to play 푣푖 • if a vertex 푉 receives a value 푣 to play from its downstream 푇(푤, 푣) neighbor 푊, and the value 푤 that 푊 will play, then it must be that 푇 (푤, 푣) = 1. TreeProp Upstream » 푉 chooses a witness 푢 to 푇 (푤, 푣) = 1, and passes each parent 푈푖 their value 푢푖 as well as 푣 » Note that the semantics of 푇 (푤, 푣) = 1 ensure that 푉 = 푣 is a best response 푇(푣, 푢푖) to 푈 = 푢, 푊 = 푤. TreeProp Algorithm Flow » Downstream Pass: • each node 푉 receives 푇(푣, 푢푖) from each 푈푖; • node 푉 computes 푇(푤, 푣) and witness lists for each 푇(푤, 푣) = 1 » Upstream Pass: • each node 푉 receives value (푤, 푣) from node W, s.t.
Details
-
File Typepdf
-
Upload Time-
-
Content LanguagesEnglish
-
Upload UserAnonymous/Not logged-in
-
File Pages65 Page
-
File Size-