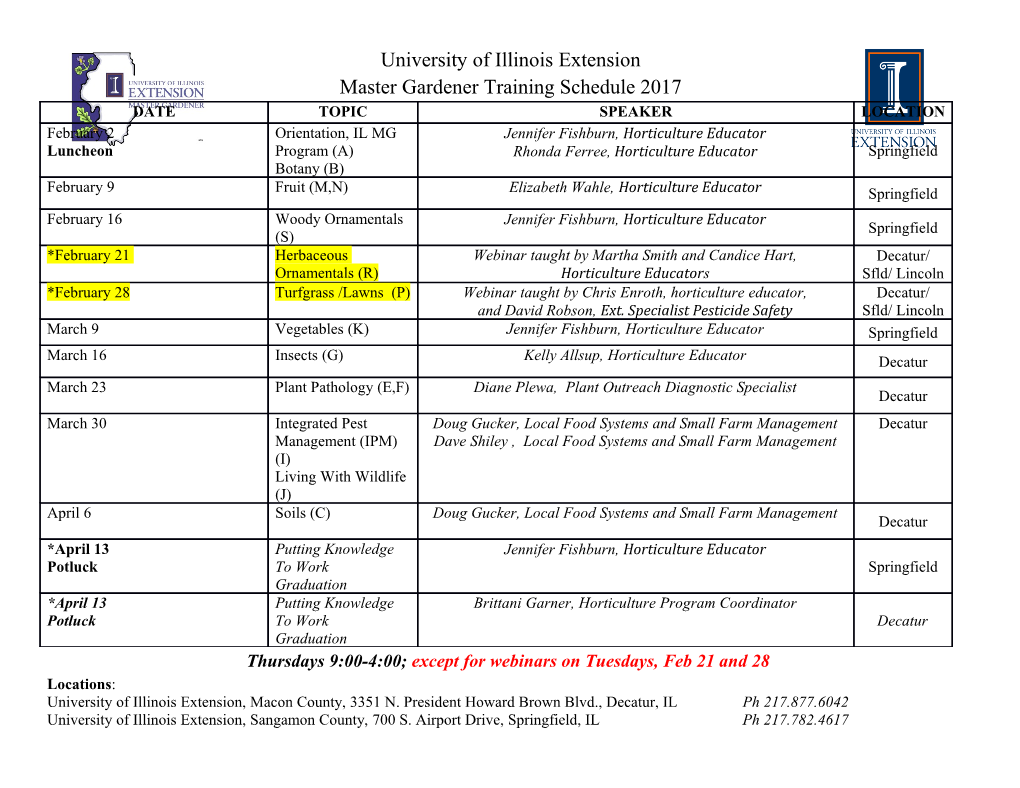
Interrelation of Droughts and Floods through Outlier Detection on Rivers in Serbia Borislava Blagojevic1, Aleksandra Ilic2, Stevan Prohaska2 1 Faculty of Civli Engineering and Architecture, Nis, Serbia 2 Jaroslav Černi Institute for the Development of Water Resources, Belgrade, Serbia Abstract As floods and droughts are normal, recurrent features in climate, this paper will present an introduction to an in-depth analysis of draught and flood interrelation on rivers in Serbia. There are 144 gauge stations flow data available for analysis. These stations form an observation network of Hydro-Meteorological Service of the Republic of Serbia. Gauge stations from the Province of Vojvodina will not be taken into consideration due to unnatural flow regime caused by Danube-Tisa-Danube channel. Data sets to be analyzed are annual minima, maxima, average flow, and 30-day minima. Outlier identification will be performed by Pilot and Harvey test. It is expected that extremely wet/dry years will be shown as synchronized outliers at a gauge station in each of the tested series either as high or low outlier. Taking existence of an outlier as the only drought/flood criteria, new series of extreme events will be formed. Data for years when low outlier appears in the series of annual minima will be excluded from the series of annual maxima and vice versa. This approach will lead to formation of series with data from the same population. Statistics of the series of extreme events will be compared for observed series, series from the same population and series of 30 days minima. The significance of difference in statistics of these series will be analyzed and presented. Regions prone to hydrologic droughts, floods, or both are expected to be identified for the territory of Serbia. Key words: floods, hydrologic draught, outliers Introduction This paper presents an introduction to an in-depth analysis of draught and flood interrelation on rivers in Serbia. The research is part of an interdisciplinary project ‘Extreme Hydrologic Features: Floods and Droughts’. Taking outliers in hydrologic series as the only indicators of extreme floods and draughts, a working hypothesis for this research was set: extremely wet/dry years would show as synchronized outliers at a gauge station in each of the tested series, either as high or low outlier. It was also expected that regions prone to hydrologic droughts, floods, or both, could be identified on the territory of Serbia. Studied area and input data The studied area was territory of Serbia, south of Danube (Dunav) and Sava rivers. Within this territory, rivers belonging to the Black and Aegean Sea basins were investigated. Flow records at hydrologic gauge stations (G.S.) from the Republic of Serbia Hydro-Meteorological Service (RHMZS) Surface Water Observation Network were used. Figure 1 shows river network and division into sub--basins. Calculation and analyses were performed on the following series - data sets: annual minima (AMIN), annual maxima (AMAX), annual average flow (AAVG), and 30-day minima (30DMIN). The following data sets were not taken into consideration: less than 25 years of observation, unnatural river regime, and sets containing zero-flow in AMIN. BALWOIS 2010 - Ohrid, Republic of Macedonia - 25, 29 May 2010 1 Figure 1 River network of Serbia and division into sub-basins. Study area on the south of Danube (Dunav) and Sava rivers and excluding sub-basin of the Beli Drim river on the south. Image source: http://www.hidmet.gov.rs/eng/hidrologija/povrsinske/index.php Methodology Detection of outliers was performed by Pilot and Harvey test, following research on historic floods in Serbia (Prohaska et. al. 2009). Extreme events testing In hydrological practice, historic events are those which, in an uninterrupted ascending series (time series), considerably exceed or deviate from the subsequent, neighboring values for the event under consideration. The Pilot and Harvey test is generally used to obtain an objective detection of historic events (outliers) under extreme hydrologic conditions (floods and droughts). The assumption is that quantitative characteristics of these conditions adhere to the Log-Pearson III (LPT3) probability distribution. Under such circumstances, the upper and lower limits for the outliers are computed using the following formulas (1), (2): • Upper limit YH = Yav + KN Sy (1) • Lower limit YL = Yav - KN Sy (2) (these equations apply if -0.4> Csy > 0.4), BALWOIS 2010 - Ohrid, Republic of Macedonia - 25, 29 May 2010 2 where: YH – log of the value of the upper limit of the outlier; YL – log of the value of the lower limit of the outlier; Yav – average value of the time series Y; Y = log X X - observed time series; Sy – standard deviation of the time series Y; Csy –skew coefficient of the time series Y; KN – frequency factor (critical value) for the risk coefficient ά=10% N - total number of members for the Y series for which statistical parameters will be calculated. The frequency factor, KN, is computed using the formula (3): 0.25 0.5 0.75 KN = -3.6220 + 6.2844 N – 2.49835 N + 0.491436 N – 0.037911 N (3) The historic flood detection procedure itself involves a comparison of empirical distribution functions with defined outlier limits. If any empirical point falls outside the defined (upper or lower) limit, then such a point with probability 1- ά = 0.90 is deemed to represent a historic event. In compliance with the test conditions, only the data sets that satisfied -0.4> Csy > 0.4 condition were taken into consideration for investigation. Calculations of statistical parameters and return periods of outliers In order to estimate return period of detected outliers by Pilot and Harvey test, procedure below has to be preformed because calculated statistical parameters do not reflect the actual characteristics of the analyzed processes. When outliers are detected, they have to be adjusted based on whether they occurred during a monitoring period or not, assuming that the random variable X follows the Pearson 3 (PT3) or LPT3 distribution. If an outlier occurs outside of an observation period, n, and if it is not exceeded during a longer period of time, N, then the empirical probabilities of a tested series Pi (i=1,2,3,......, n+1) are computed using the formula (4): P1 = 1/(N+1), P2 = 1/(n+1), P3 = 2/(n+1) , P4 = 3/(n+1), ......, Pn+1 = n/(n+1) (4) If two outliers have occurred, one during the monitoring period n, and the other outside that period, and neither have been exceeded during the longer period of time N, then empirical probabilities of series Pi are computed based on the formula (5): P1 = 1/(N+1), P2 = 2/(N+1), P3 = 3/(n+1) , P4 = 4/(n+1), ......, Pn+1 = n/(n+1) (5) If the random variable X follows the PT3 or LPT3 distribution, then adjusted statistical parameters for a single outlier outside the monitoring period are computed using the formulas (6), (7), (8): • Average value – Xav,N ⎡ n X ⎤ X + (N −1) i ⎢ N ∑ n ⎥ X = ⎣ i=1 ⎦ (6) av,N N where: XN is the value of outlier – random variable X, which has not been exceeded during the time period N, and Xi are values of the members of the basic data series for the monitoring period n. BALWOIS 2010 - Ohrid, Republic of Macedonia - 25, 29 May 2010 3 • Coefficient of variation – Cv,N 1 ⎡ N −1 n ⎤ 2 2 (7) Cv,N = ⎢(k N −1) + ∑ (ki −1) ⎥ N −1 ⎣ n i=1 ⎦ where: X N k N = is the value of the modulus coefficient for outlier, X av,N X i ki = is the modulus coefficient of the random variable X during the monitoring period. X av,N • Skewness coefficient – Cs,N N ⎡ N −1 n ⎤ C = (k −1)3 + (k −1)3 (8) s,N 3 ⎢ N ∑ i ⎥ (N −1)(N − 2)Cv,N ⎣ n i=1 ⎦ If there are two outliers, one during and the other outside the monitoring period, then statistical parameters are computed using the following formulas (9), (10), (11): • Average value – Xsr,N ⎡ n X ⎤ X + X + (N − 2) i ⎢ N N −1 ∑ n ⎥ X = ⎣ i=2 ⎦ (9) av,N N • Coefficient of variation – Cv,N 1 ⎡ N − 2 n ⎤ 2 2 2 (10) Cv,N = ⎢(k N −1) + (k N −1 −1) + ∑(ki −1) ⎥ N −1 ⎣ n −1 i=2 ⎦ • Skewness coefficient – Cs,N N ⎡ N − 2 n ⎤ C = (k −1)3 + (k −1)3 + (k −1)3 (11) s,N 3 ⎢ N N −1 ∑ i ⎥ (N −1)(N − 2)Cv,N ⎣ n −1 i=2 ⎦ If an outlier occurs within or outside series which follow the LPT3 distribution, the following procedure is used to adjust the statistical parameters: The weight coefficient – W is determined based on the number of events which fall outside the outlier limits, using the formula (12): N − Z W = (12) n + L where: Z is the number of high outliers, which is above high outlier limit L is the number of low outlier, which is lower than low outlier limit BALWOIS 2010 - Ohrid, Republic of Macedonia - 25, 29 May 2010 4 In case of both high and low outliers, the adjusted values of the statistical parameters are determined using the log values of the basic random variable X (or using the random variable Y), as follows Average * values - YL n Z W ∑∑Yi,L + Y j,L Y * = i==11j (13) L N −WL * 2 • Variance – ( S L ) n Z * * 2 W ∑∑(Yi,L − Y L ) + (Y j;L − Y L ) (S * )2 = i==11j (14) L N −WL −1 * • Skewness coefficient - GL n Z ⎡ * 3 * 3 ⎤ ⎢W ∑∑(Yi,L − YL ) + (Y j,L − YL ) ⎥ N −WL * ⎢ i==11j ⎥ (15) GL = * 3 (N −WL −1)(N −WL − 2) ⎢ (S L ) ⎥ ⎢ ⎥ ⎣ ⎦ Empirical probabilities are calculated using the expression (16): m* P = (16) N +1 with: m* = m for 1≤ m ≤ Z m* = Wm – (W-1)(Z+0.5) for (Z +1) ≤ m ≤ (Z + n + L) where: m* is the weighted order, m is the order of the data point in the series.
Details
-
File Typepdf
-
Upload Time-
-
Content LanguagesEnglish
-
Upload UserAnonymous/Not logged-in
-
File Pages13 Page
-
File Size-