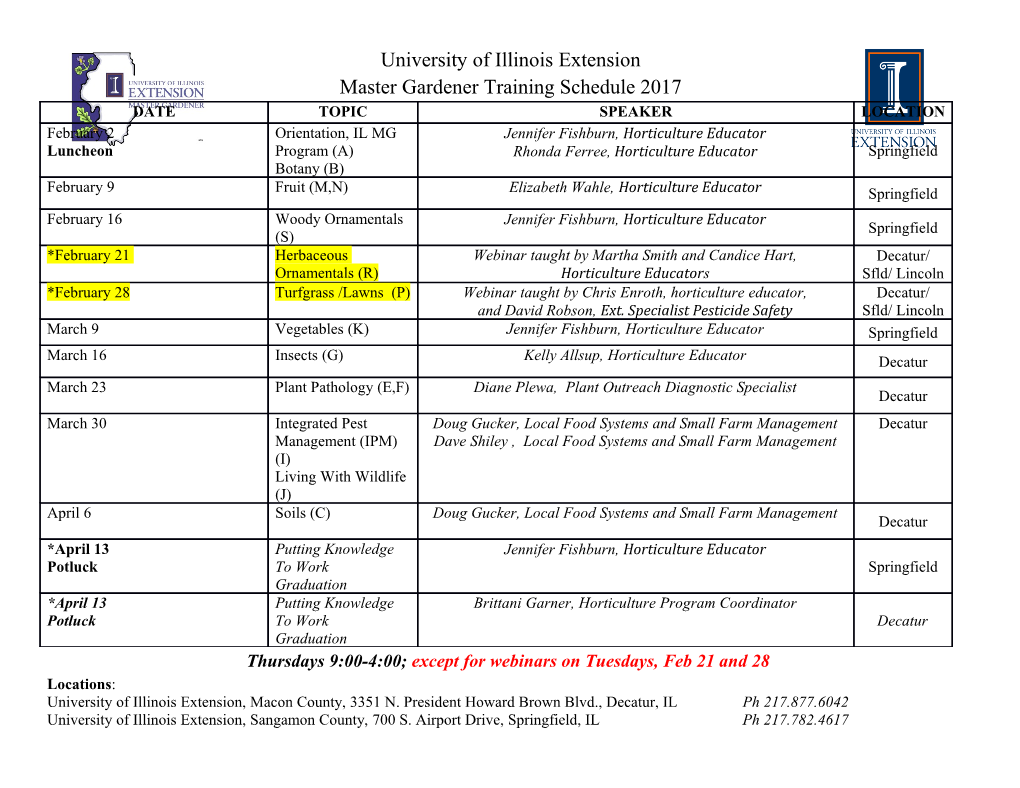
Discovering variable length phrases from symbolic notation of Carnatic music Ranjani, H. G. and Prof. T. V. Sreenivas [email protected], [email protected] Problem Formulation • Given symbolic transcript of a r¯aga, discover repeti- QQA QQA • Any rhythm cycle A = [u1; u2; u3; : : : ; uTA ] s.t. p(A ) = k=1 p(sk) , k=1 θk tive phrases where sk is such that jskj ≤ N and for any TA > N, QA > 1. and s1 = [ub0 ; : : : ub1 ], s2 = [ub1+1; : : : ub2 ] and D P M , G R S , R N D D P P S , s = [u ; : : : u ], b = 1 and b = T . QA bQA−1 +1 bQA 0 QA A In - - - tha - - - cha - - - - - la - S , N S G R G , M , M , G R G M || • A typical segmentation on A gives : A ≡ [s1; s2; s3 ; : : : sQA ] - - mu - - - je - si - - - the - - - P , M- D - P- S N D P- S N D R S , • Z = fbkg; k = 1 : QA e - - ma - - ni - - - - - - - tha - n o P D P- N , D , P M , G , R- G M P || ∗ old - - - lu - - - - du - - - - ra - - • Estimate parameters, θk to maximize posterior p(ZjA; θ): θ = arg maxθ maxZ log p(ZjA; θ ) S ,- D D P- N N D M P D N D P- M , PY An - - - - tha - - ran - - - - - gu - • Constraint : θk = 1 where, Y is total number of unique phrases k=1 G R S- M G M- R G M P- P D M , , , || da - - ni - - - - - - mo - vi - - - • Algorithm : P D ,- D P M- M P ,- P M G R G M P A - - na - - va - - - - - cchi - - - – 1. Find Z∗, Z∗ = arg max log p(A;Z; θold) = arg max log p(AjZ; θold)p(Z; θold) Z2Z Z2Z M D , P S N D P S N D R S , , , || Figure 1: Sample- - - - symbol the - - - na transcript - - - tho - - of - Begada r¯aga. – 2. Update parameters Z∗ P D P S N S- G R G M G M R ,- P M new cj Pan - - tha - - me - - - - - la - - - • Let transcript be denoted by A = [A ;A ;:::A ]. θj = ∗ 1 2 I cZ R , ,- R S N D D P- S , N D N S R|| ra - - - - - sree - - ve - - nu - - - • Any rhythm cycle, Ai [ut=1ut=2 : : : ut=T ], where R S , N D P- D M , , G M R G M P D Ai swaras, uGo -2 - Vpa , - with - la - V - da = - saf S; pa R; - - G; - M; P; D; N; Sg. t Results P S ,- P D P- N , D P M G R G M P ri - - - - - pa - - - - - - la - - D , P M ,- D P M G R ,- G , M P D M G , R S N- R S ,- R N D P D P S N R , S G R- P M , P G R G M P D Figure 2: Rough pitch contours of more than 100 rhythm Figure 3: Rough pitch contours of more than 100 ¯avarthanas from training data of r¯agaBegada (in blue) and top ten cycles from symbolic transcripts oBegada r¯aga. frequently occurring phrases (sorted aided by other colors) as discovered by 8-multigram. Two characteristic phrase(s) are highlighted using (black and red) arrowheads. • Multiple and unknown phrases • N determines maximum length of sub=sequence • Variable length phrases • Propose a modified 2-stage approach: Y Assumptions – Obtain fskgk=1 containing ≤ N length phrases, using multigram training n o • Rhythm cycle contains note sequences : concatena- 0 Y – Create new vocab: V = V [ fsi : jsij = N; θi > Pthrg; 8i 2 fsigi=1 . tion of independent phrases 0 – Replace any occurrence of si in data with its corresponding entry from V • Phrases are well within rhythm cycle 0 Y 0 0 – Obtain fsjgj=1 containing N + N length phrases through a second stage of multigram training • Phrases are repeated across rhythm cy- cles/compositions Experimental details • Publicly available online database [http://www.shivkumar.org/music/] (notations by Dr. Shivakumar Kalyanaraman) • Experiments on 12 r¯agas: Hari-Kambhoji, Bhairavi, Shankar¯abharana, Th¯odi, N¯attai, Panthuvar¯ali, Madhyam¯avathi, Khamas, Begada, Kalyani, Reethigowla and Sahana • Octave folded • Each note of unit duration Figure 4: Perplexity values of N-gram, N-multigram and modified (N; N 0)-multigram on training and testing symbolic music data for the r¯agas considered. • Training: > 2000 note sequences; Testing: > 1500 per r¯aga • Performance measures: perplexity, semantic rele- vance Conclusions • Use of 7 notes as generally available in transcription • Discovering grammatical structure of music • Obtain phrases containing varied length sub- sequences • Multigram perplexity lower than N-gram on training and test data • Modified multigram for longer length sequences Figure 5: Rough pitch contours of more than 100 ¯avarthanas from training data of r¯agaBegada (in blue) and top ten • Appreciable number of musicological phrases cap- frequently occurring phrases (sorted aided by other colors) as discovered by modified N 0-multigram with (N; N 0) = (8; 8). tured Two characteristic phrase(s) are highlighted using (black and red) arrowheads..
Details
-
File Typepdf
-
Upload Time-
-
Content LanguagesEnglish
-
Upload UserAnonymous/Not logged-in
-
File Pages1 Page
-
File Size-