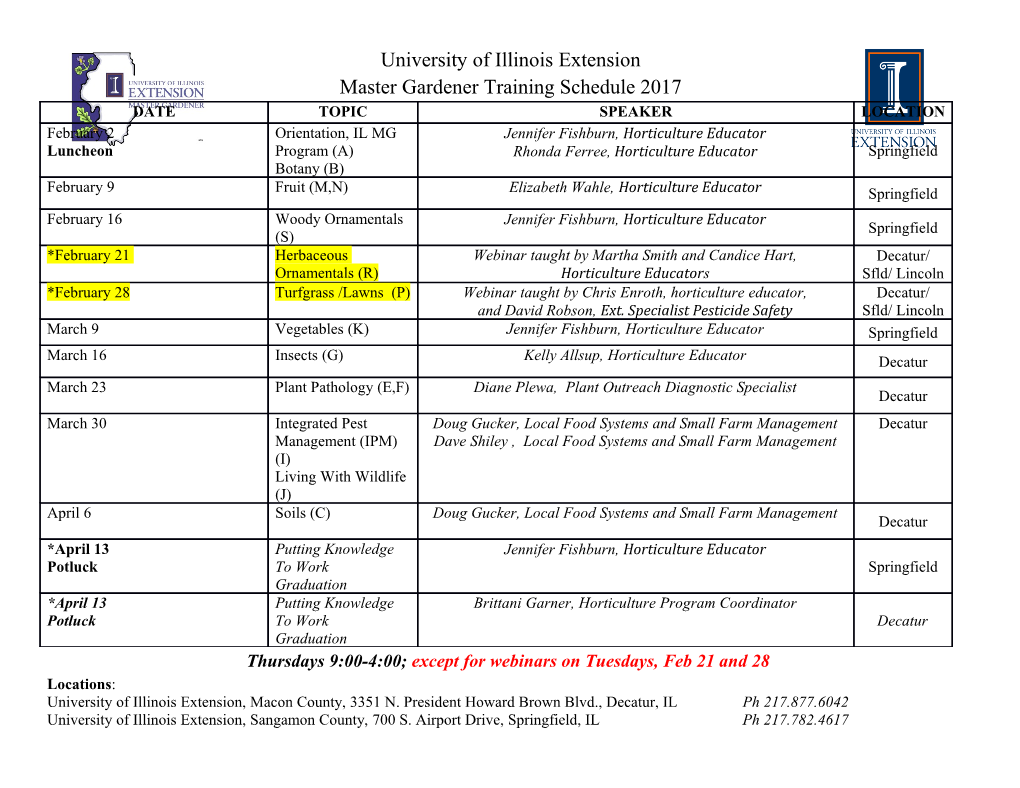
University of Pennsylvania ScholarlyCommons Publicly Accessible Penn Dissertations 2016 Partial Information Framework: Basic Theory and Applications Ville Antton Satopää University of Pennsylvania, [email protected] Follow this and additional works at: https://repository.upenn.edu/edissertations Part of the Applied Mathematics Commons, Mathematics Commons, and the Statistics and Probability Commons Recommended Citation Satopää, Ville Antton, "Partial Information Framework: Basic Theory and Applications" (2016). Publicly Accessible Penn Dissertations. 1991. https://repository.upenn.edu/edissertations/1991 This paper is posted at ScholarlyCommons. https://repository.upenn.edu/edissertations/1991 For more information, please contact [email protected]. Partial Information Framework: Basic Theory and Applications Abstract Many real-world decisions depend on accurate predictions of some future outcome. In such cases the decision-maker often seeks to consult multiple people or/and models for their forecasts. These forecasts are then aggregated into a consensus that is inputted in the final decision-making process. Principled aggregation requires an understanding of why the forecasts are different. Historically, such forecast heterogeneity has been explained by measurement error. This dissertation, however, first shows that measurement error is not appropriate for modeling forecast heterogeneity and then introduces information diversity as a more appropriate yet fundamentally different alternative. Under information diversity differences in the forecasts stem purely from differences in the information that is used in the forecasts. This is made mathematically precise in a new modeling framework called the partial information framework. At its most general level, the partial information framework is a very reasonable model of multiple forecasts and hence offers an ideal platform for theoretical analysis. For one, it explains the empirical phenomenon known as extremization. This is a popular technique that often improves the out-of-sample performance of simple aggregators, such as the average or median, by transforming them directly away from the marginal mean of the outcome. Unfortunately, the general framework is too abstract for practical applications. To apply the framework in practice one needs to choose a parametric distribution for the forecasts and outcome. This dissertation motivates and chooses the multivariate Gaussian distribution. The result, known as the Gaussian partial information model, is a very close yet practical specification of the framework. The optimal aggregator under the Gaussian model is shown to outperform the state-of-the-art measurement error aggregators on both synthetic and many different types of real-world forecasts. Degree Type Dissertation Degree Name Doctor of Philosophy (PhD) Graduate Group Statistics First Advisor Lyle H. Ungar Second Advisor Shane T. Jensen Keywords Expert belief, Forecast heterogeneity, Judgmental forecasting, Model averaging, Noise reduction, Probability modeling Subject Categories Applied Mathematics | Mathematics | Statistics and Probability This dissertation is available at ScholarlyCommons: https://repository.upenn.edu/edissertations/1991 PARTIAL INFORMATION FRAMEWORK: BASIC THEORY AND APPLICATIONS Ville A. Satopa¨a¨ A DISSERTATION in Statistics For the Graduate Group in Managerial Science and Applied Economics Presented to the Faculties of the University of Pennsylvania in Partial Fulfillment of the Requirements for the Degree of Doctor of Philosophy 2016 Supervisor of Dissertation Co-Supervisor of Dissertation Shane T. Jensen Lyle H. Ungar Associate Professor of Statistics Professor of Computer and Information Science Graduate Group Chairperson Eric Bradlow K.P. Chao Professor, Marketing, Statistics and Education Dissertation Committee Shane T. Jensen, Associate Professor Robin Pemantle, Professor Lyle H. Ungar, Professor Philip E. Tetlock, Professor Edward I. George, Professor PARTIAL INFORMATION FRAMEWORK: BASIC THEORY AND APPLICATIONS COPYRIGHT © 2016 Ville A. Satopa¨a¨ Dedication This dissertation has been dedicated to my mother, whose love and support give me courage to take on anything, and to my father, who lives on as the inspiration in everything I do. Tam¨ a¨ tohtoruus on omistettu aidilleni,¨ jonka rakkaus ja tuki antaa minulle rohkeutta ko- hdata ihan mita¨ vain, ja isalleni,¨ joka ela¨a¨ inspiraationa kaikessa mita¨ teen. iii Acknowledgments First and foremost, I would like to thank my family and friends whose unquestioning sup- port has allowed me to chase my dreams to the edge of the world and back again. Second, I would like to thank my thesis committee for all their mentoring and advice about research, life, and everything in between. Lastly, I would like to thank my two Ph.D. advisors for always having my back, for reminding me of the bigger picture when I was lost in the de- tails, and for making me a much better researcher. Thanks to them, I could not feel any better-equipped to begin my career as a professor. Ensinnakin¨ haluan kiitta¨a¨ perhettani¨ ja ystavi¨ ani¨ joiden ehdoton tuki on antanut minulle mahdollisuuden jahdata haaveitani maailman reunalle asti ja takaisin. Toiseksi haluan ki- itta¨a¨ tutkielmani komiteaa kaikesta mentoroinnista ja neuvoista liittyen tutkimustyoh¨ on,¨ elam¨ a¨an,¨ ja kaikkeen siita¨ valilt¨ a.¨ Lopuksi haluan kiitta¨a¨ kahta tohtoruuden valvojaani siita¨ etta¨ ovat aina pitaneet¨ puoltani, siita¨ etta¨ ovat muistuttaneet minua kokonaiskuvasta kun olen havinnyt¨ yksityiskohtiin, ja siita¨ etta¨ ovat tehneet minusta paljon paremman tutkijan. Heidan¨ ansiosta en voisi tuntea itseani¨ enemman¨ valmiiksi aloittamaan urani professorina. iv ABSTRACT PARTIAL INFORMATION FRAMEWORK: BASIC THEORY AND APPLICATIONS Ville A. Satopa¨a¨ Shane T. Jensen Lyle H. Ungar Many real-world decisions depend on accurate predictions of some future outcome. In such cases the decision-maker often seeks to consult multiple people or/and models for their forecasts. These forecasts are then aggregated into a consensus that is inputted in the final decision-making process. Principled aggregation requires an understanding of why the forecasts are different. Historically, such forecast heterogeneity has been explained by measurement error. This dissertation, however, first shows that measurement error is not appropriate for modeling forecast heterogeneity and then introduces information diversity as a more appropriate yet fundamentally different alternative. Under information diversity differences in the forecasts stem purely from differences in the information that is used in the forecasts. This is made mathematically precise in a new modeling framework called the partial information framework. At its most general level, the partial information framework is a very reasonable model of multiple forecasts and hence offers an ideal platform for the- oretical analysis. For one, it explains the empirical phenomenon known as extremization. This is a popular technique that often improves the out-of-sample performance of simple aggregators, such as the average or median, by transforming them directly away from the marginal mean of the outcome. Unfortunately, the general framework is too abstract for practical applications. To apply the framework in practice one needs to choose a paramet- ric distribution for the forecasts and outcome. This dissertation motivates and chooses the multivariate Gaussian distribution. The result, known as the Gaussian partial information model, is a very close yet practical specification of the framework. The optimal aggregator under the Gaussian model is shown to outperform the state-of-the-art measurement error aggregators on both synthetic and many different types of real-world forecasts. v Contents 1 Introduction 1 2 Combining Multiple Probability Predictions Using a Simple Logit Model 10 2.1 Introduction . 10 2.2 Theory . 14 2.3 Results and Discussion . 20 2.4 Conclusions . 33 2.5 Acknowledgements . 36 3 Probability Aggregation in Time-Series: Dynamic Hierarchical Modeling of Sparse Expert Beliefs 37 3.1 Introduction . 38 3.2 Geopolitical Forecasting Data . 41 3.3 Model . 43 3.4 Model Estimation . 46 3.5 Synthetic Data Results . 49 3.6 Geopolitical Data Results . 53 3.7 Discussion . 66 3.8 Acknowledgements . 67 4 Modeling Probability Forecasts via Information Diversity 69 4.1 Introduction and Overview . 70 4.2 Prior Work on Aggregation . 75 4.3 The Gaussian Partial Information Model . 78 4.4 Probability Extremizing . 85 4.5 Probability Aggregation . 91 4.6 Summary and Discussion . 96 4.7 Acknowledgments . 98 vi 5 Partial Information Framework: Model-Based Aggregation of Estimates from Diverse Information Sources 99 5.1 Introduction . 100 5.2 Model-Based Aggregation . 104 5.3 Model Estimation . 116 5.4 Applications . 124 5.5 Discussion . 135 5.6 Acknowledgments . 137 6 Bayesian Aggregation of Two Forecasts in the Partial Information Framework139 6.1 Introduction . 140 6.2 Aggregation function for fixed parameters . 144 6.3 Bayesian model . 146 6.4 Comparison of Aggregations With Hypothetical Data . 147 6.5 Comparison of Estimators with 2012 Presidential Election Data . 150 6.6 Acknowledgments . 154 7 Combining and Extremizing Real-Valued Forecasts 155 7.1 Introduction . 156 7.2 Forecast and Aggregation Properties . 160 7.3 Extremizing Real-Valued Forecasts . 165 7.4 Simulation Study . 168 7.5 Case Study: Concrete Compressive Strength . 175 7.6 Summary and Discussion . 179 7.7 Acknowledgements . 182 8 Conclusion
Details
-
File Typepdf
-
Upload Time-
-
Content LanguagesEnglish
-
Upload UserAnonymous/Not logged-in
-
File Pages253 Page
-
File Size-