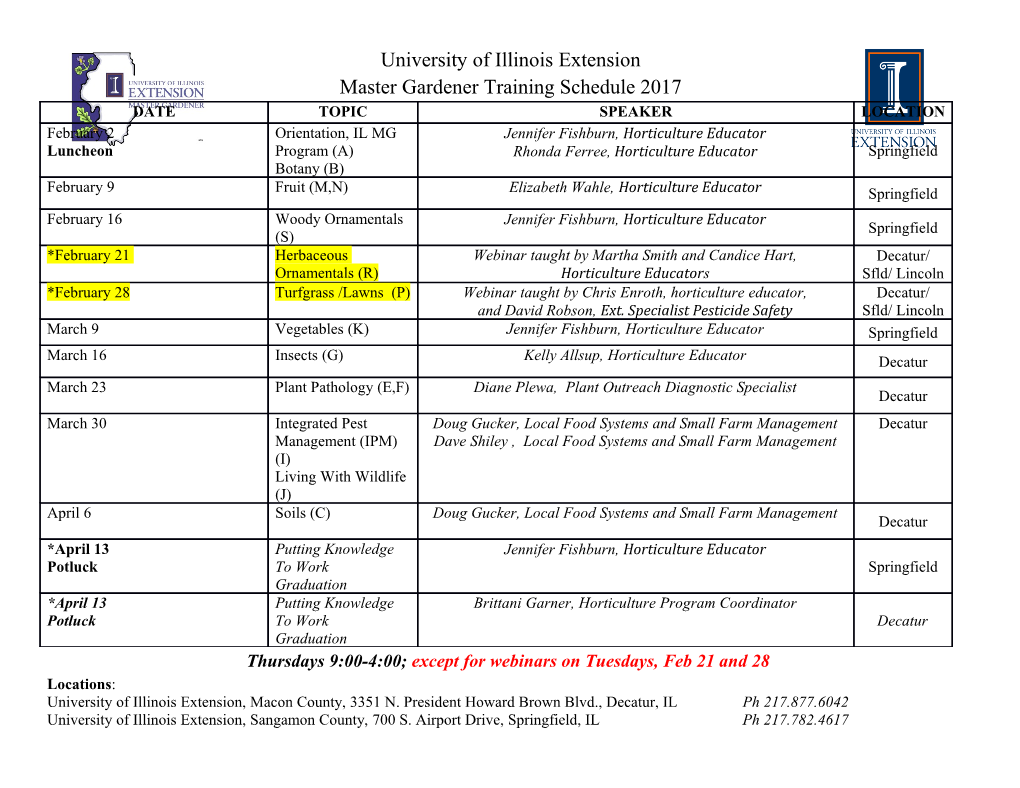
Real-Time Actionable Insights with IBM Streams — Roger Rea IBM Streams Senior Offering Manager [email protected] Data and AI Forum 2019 Session 309 Data and AI Forum / © 2019 IBM Corporation Real-time processing needs are growing Data and AI Forum / © 2019 IBM Corporation Continuous intelligence – What if you could analyze data as it’s created? – What if you could visualize your business? – What if you could better predict your customers’ needs? – What if you could gain insights from unstructured data like audio, text or video? – What if could automate immediate actions? – What if you always knew where your assets were, and where they would be? – What if you could update machine learning models continuously? And do it all in real time? IBM Data & AI / © 2019 IBM Corporation “Continuous intelligence is a design pattern in which real-time analytics are integrated into a business operation, processing current and historical data to prescribe actions in response to business moments and other events.” Gartner: Innovation Insight for Continuous Intelligence Data and AI Forum / © 2019 IBM Corporation 4 Continuous intelligence Stream – Engage data from inside and outside processing of applications or business operations – Enable fast-paced digital business decisions Artificial Machine and process optimization intelligence learning Continuous – Leverage AI, ML, data analytics, real-time intelligence analytics and streaming event data to deliver business optimized solutions – Ensure you can take advantage of the Business rules Deep “perfect storm” of rising supply and management and decision learning demand for real-time situation awareness systems and responsiveness IBM Data & AI / © 2019 IBM Corporation Streams Real-time Analytics Platform TCP TCP SOURCE Recommendation Customer Signature detection Engine affinity UDP UDP Consumption Engine TCP Consumer ingest ingest apps Consumer SINK Edge adapters Edge MQ adapters Edge Systemic memory profile and analytics scoring Final customer MQ store HTTP product affinity Click HTTP Product Owners Stream Big Big data sources HDFS HDFS Purchases Recommendation DM models API ODBC ODBC Mobility & Landline Context Affinity Business rules filtering Continuous Activity FILES Files Product TV affinity Content Mgmt. intelligence Activity Consumer Dynamic Viewing Campaign Mgmt. SPSS C&DS example YP.COM Dashboard CE Profile Mgmt. URL Classificati running over SPSS Modeling on Development only DataRepositories STREAMS FILE LANDING ZONE (I/O) User 1700 models Data Reference Identification → Iterative analytical model in production Catalogs Data Lake deployment Production Feeds Demograp →Real-time customer and DEV T.Data Data Warehouse hics clickstream activity Customer DataSource Samples Analytics Schema & Account Consumer Data Consumer Info Systemic Memory → Real-time Content/ Profile Reporting Views Campaign ingestion Modeling Dev. Data Warehouse Data Warehouse →Real-time recommendations Production IBM Streams to act on all your data in real time Market leader in Enterprise Ready: streaming analytics included with Cloud Pak for Data – Machine Learning – Visual development – Model Scoring – Web console – Geospatial – Management – Video/Image – Enterprise – Text, Speech to connectors like Text, Predictive, JSON, JMS, MQ, Descriptive MQTT Data and AI Forum / © 2019 IBM Corporation 7 Streams to act on all your data in real time Filter /Sample Transform Correlate Classify, Annotate Data and AI Forum / © 2019 IBM Corporation 8 IBM Streams offerings Free Lite Plan – 50 Available 1Q19 hours a month Streams v4.3.1 July 2019 Streams for IBM Cloud Pak Streaming Analytics for Data Streams runtime Streams runtime Streams runtime Containers Baremetal/VM Containers SaaS Common runtime: develop anywhere and deployment everywhere Moved from VM to Containers in 2018 Data and AI Forum / © 2019 IBM Corporation *Moved from VM to Containers in 2018 9 IBM Streams development options Streams Developer IBM Cloud Pak Watson Studio Plus IBM Runner Edition, Streams for Data Streams Flows for Apache Beam, Quick Start Edition – Integrated data in IBM Cloud Java, Scala, Python – Dedicated Streams and AI project – Integrated data and beta plug-ins development IDE experience and AI project for VSCode and Atom and tools – Containers* experience – Local machine – SaaS Python Notebooks Quick Start free Available 4Q18 for non-production *Moved from VM to Containers in 2018 Data and AI Forum / © 2019 IBM Corporation 10 IBM Streams at a glance And even more at github.com/IBMStreams Out of the box with over 200 operators with 1300 functions Communications IBM Streams Hadoop Hadoop data sources Scale-out Runtime HDFS, GPFS, Hive, Hbase, BigSQL, – TCP/IP Parquet, Thrift, Avro – UDP/IP Primary analytics – HTTP – Filter – FTP – Enrich Data RDBMS – RSS – Normalize warehouse IBM Db2, IBM Db2 Parallel writer, – Messaging Toolkit (Kafka, – Windowed Aggregations IBM Db2 Event Store, IBM Informix, XMS, IBM MQ, Apache – Machine Learning IBM BigSQL, IBM Netezza, IBM ActiveMQ, RabbitMQ, MQTT) Netezza NZLoad, Oracle, Microsoft – IBM Data Replication – Scoring (SPSS, Python, R, MLlib) SQL Server, MySQL, Teradata, HP – IP Packet ingest Aster, HP Vertica – Signal Processing Application – CEP & Pattern Matching development – xDR Mediation – Geospatial NoSQL – Java NoSQL – Video/Image – Scala Key Value Stores (Memcached, Redis, – Text Analytics (AQL, UIMA) – Python Redis-Cluster, Aerospike), Column – Speech to Text Oriented Stores (Cassandra, Hbase), – Drag/Drop Streams Processing Document Oriented Stores (IBM Language (SPL) – Rules Cloudant, Mongo, Couchbase, Elastic – – Deep Packet Inspection VS Code, Atom for SPL Search), Object Store Data and AI Forum / © 2019 IBM Corporation 11 Machine learning and real time analytics with “The science of getting computers to act without IBM streams being explicitly programmed”1 Many different approaches to Machine Learning: Use machine learning models created with Watson – Mechanisms: Supervised and Unsupervised Studio to score live data on Streaming Analytics – Algorithms: Decision Trees, Regressions, Classification, using Watson Studio Streams Flows. Clustering, etc. – Inputs: Single and multi-variant, rich feature vectors – Streams has 20 ML algorithms that learn as you go in real time – Streams scores models created offline from popular tools including IBM SPSS, Watson Machine Learning, SparkMLLib, Python, R & PMML libraries – Native ML and Model scoring can all be integrated within a Streams application. With this approach, real time scores can be generated on the incoming data Data and AI Forum / © 2019 IBM Corporation 12 Streams: Use the language of choice Streams Processing Language stream<rstring item> Sale = Join(Bid; Ask) { window Bid: sliding, time(30); Streams Processing Language (SPL) Ask: sliding, count(50); param match : Bid.item == Ask.item – Tailored to stream processing && Bid.price >= Ask.price; output Sale: item = Bid.item; – High-level, declarative composition language Java Topology } – Graphical editor support /* * Declare a source stream (hw) with String as tuples that sends * two tuples "Hello" and "World!", and prints them to output. Create topologies in Java */ Topology = new Topology("HelloWorld"); – Indirect support for Scala TStream<String> hw = topology.strings("Hello", "World!"); hw.print(); Python topologies and operators StreamsContextFactory.getEmbedded().submit(topology).get(); – Integration with Jupyter notebook Python Topology – Integration with IBM Watson Studio – Add Python functions in line with SPL code Publish/subscribe data exchange – Between applications written in any language Data and AI Forum / © 2019 IBM Corporation 13 Advantage: Scale out with ease Create logical application flow – Without concern for throughput limitations As needed, add parallel paths – Based on runtime performance profiling User-Defined Parallelism (UDP) – Simple change in code or graphical editor • @parallel annotation Data and AI Forum / © 2019 IBM Corporation 14 Static vs. dynamic composition Static connections – Specified at application development-time and do not change at run-time Dynamic connections – Partially specified at application development-time (Name or properties) – Established at run-time, as new jobs come and go • Specifications can also be updated at run-time Dynamic application composition – Incremental deployment of applications – Dynamic adaptation of applications Data and AI Forum / © 2019 IBM Corporation 15 Application scenarios and real-world use cases IBM Streams is being applied in many industries – Market and Customer Watch the Video Watch the video Watch the Video Watch the Video Watch the Video Intelligence Read the Case Study – Call Center Customer Care – Manufacturing – Personalized Customer Watch the video Watch the video Read the Case Study Read the Case Study Watch the video Experience – Network Analytics – IoT, Connected Car Watch the Video Watch the Video Watch the Video Watch the Video Watch the Video and Telematics – Cyber Security – Health and Improved Patient Outcomes Watch the Video Watch the Video See the Presentation Read the Case Study Read the Case Study – Operational Optimization Data and AI Forum / © 2019 IBM Corporation 16 Medtronic Sugar.IQ IBM Technology Medtronic Sugar.IQ The Results: Sugar.IQ with Watson Watson Platform Past–Present– 90% 36 minutes for Health Future AUC accuracy level when Average more in range – Mobile app management How have I done? predicting the risk of per day hypoglycemia two to four – Data management – Important glucose hours
Details
-
File Typepdf
-
Upload Time-
-
Content LanguagesEnglish
-
Upload UserAnonymous/Not logged-in
-
File Pages22 Page
-
File Size-