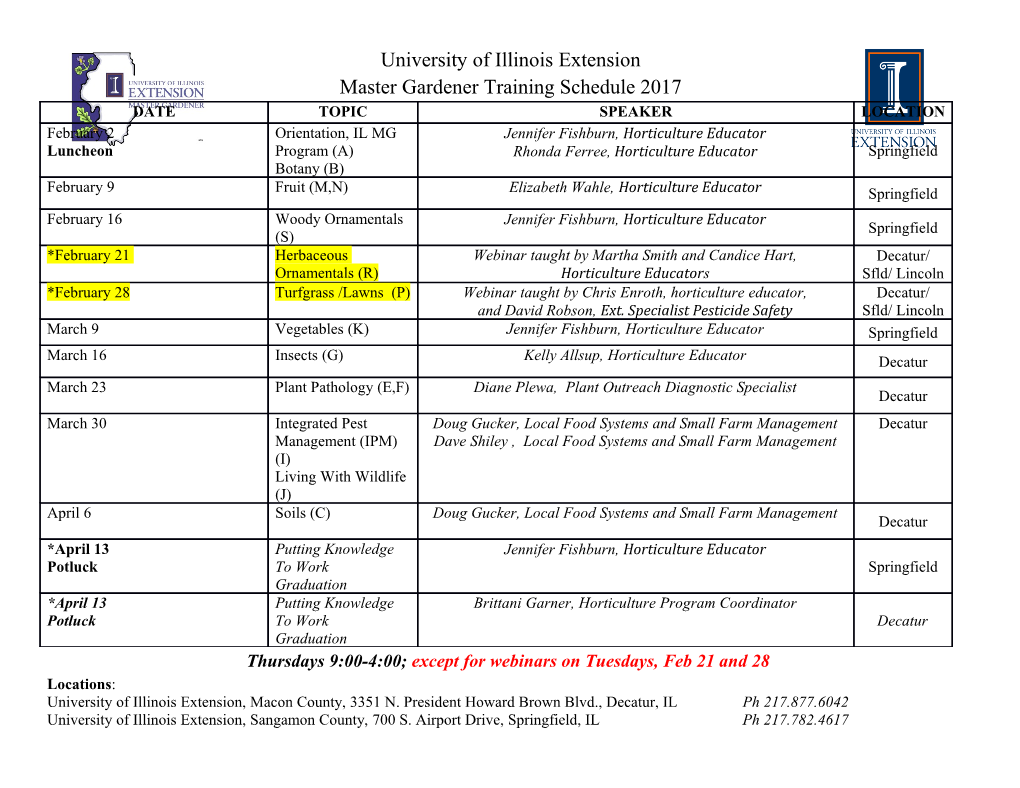
Residual in Residual Based Convolutional Neural Network In-loop Filter for AVS3 Kai Lin∗, Chuanmin Jia∗, Zhenghui Zhao∗, Li Wangy, Shanshe Wang∗, Siwei Ma∗ and Wen Gao∗ ∗Institute of Digital Media, Peking University, Beijing, China Email: fkailin, cmjia, zhzhao, sswang, swma, [email protected] yHikvision Research Institute, Hangzhou, China Email: [email protected] Abstract—Deep learning based video coding tools development the problems of ringing artifacts and restoring the overall has been an emerging topic recently. In this paper, we propose pixel-level error respectively. Inherited from AVS2, DBF, SAO a novel deep convolutional neural network (CNN) based in-loop and ALF are processed sequentially in AVS3. The combination filter algorithm for the third generation of Audio Video Coding Standard (AVS3). Specifically, we first introduce a residual of the three algorithms increases flexibility of in-loop filtering block based CNN model with global identity connection for and promotes degraded frames enhancement significantly. the luminance in-loop filter to replace conventional rule-based Convolutional neural network (CNN) facilitates the devel- algorithms in AVS3. Subsequently, the reconstructed luminance opment of substantial computer vision tasks, such as image channel is deployed as textural and structural guidance for classification [8], segmentation [9]. When it comes to video chrominance filtering. The corresponding syntax elements are also designed for the CNN based in-loop filtering. In addition, compression, CNN also shows enormous potential on deep we build a large scale database for the learning based in-loop learning based coding tools [10]. Deep learning based methods filtering algorithm. Experimental results show that our method have emerged recently to replace conventional coding tools achieves on average 7.5%, 16.9% and 18.6% BD-rate reduction including intra prediction [11], inter prediction [12], [13], in- under all intra (AI) configuration on common test sequences. loop filter [14] and so on. These methods were integrated into In particular, the performance for 4K videos is 6.4%, 15.5% and 17.5% respectively. Moreover, under random access (RA) state-of-the-art video coding standards and obtained satisfying configuration, the proposed method brings 3.3%, 14.4%, and performance. More especially, in-loop filter is certainly suit- 13.6% BD-rate reduction separately. able for CNN by modeling as restoration and inverse problem. In this paper, we propose a deep residual in residual based Index Terms—Video Coding, In-loop Filter, Audio Video Coding neural network to replace conventional in-loop filter methods Standard, Convolution Neural Network and further improve subjective and objective quality. Our contribution can be summarized as follows: I. INTRODUCTION • We build a large scale database for removing compression The third generation of the audio video coding standard artifacts based on DIV2K [15]. Our database contains (AVS3) is a newly established video coding standard de- high resolution original videos and reconstructed videos veloped by China AVS working group. On the top of the compressed by AVS3 reference software with quantiza- second generation of the audio video coding standard (AVS2) tion parameter (QP) ranging from 27 to 50. [1] [2], AVS3 achieves higher compression efficiency [3], • For luma components, we propose a residual block based especially for ultra high definition content. However, arti- fully convolutional network with global identity to re- facts such as blocking, ringing and blurring appear in the place the combination of DBF, SAO and ALF. Afterward- reconstructed images after block-based hybrid coding. Such s, to obtain better enhancement for chroma components, artifacts significantly affect the quality-of-experiences (QoE) reconstructed luminance channels are fed into network of the compressed videos and the restored images could not with chrominance simultaneously as guidance. be properly referenced by the coding of consequent pictures. • Rate-distortion optimization (RDO) is adopted to ensure As a result, suppressing these artifacts is of vital importance overall rate-distortion performances. According to RDO, and plenty of in-loop filter algorithms regarding this have been frame level and coding tree unit (CTU) level flags are investigated. designed to provide more adaptivity and flexibility. Located after inverse transform, in-loop filter contributes to alleviate artifacts and improve both subjective quality and ob- II. RELATED WORK jective quality of degraded frames. To deal with discontinuities Although the coding performance continues to be higher along block boundaries, AVS2 adopted deblocking filter (DBF) with the progress of video coding standards, the unpleasing [4] [5]. However, DBF can not deal with inner samples within artifacts still exist due to the coarse quantization and block the coding block. In addition to DBF, sample adaptive offset based prediction-transform coding framework. In this section, (SAO) [6] and adaptive loop filter (ALF) [7] were proposed we introduce previous work related to conventional in-loop step by step to compensate shortcomings of DBF by handling filter methods and deep learning based approaches separately. A. In-loop filter methods in AVS3 The combination of DBF, SAO and ALF is deployed in AVS3 as post-processing. DBF deals with visible artifacts at block boundaries [5] [16]. DBF detects discontinuities at the block boundaries and attenuates them by applying a filter. According to distribution of samples near boundaries, deci- sions between different filter levels are accomplished. Besides samples along block boundaries, SAO is presented to adjust samples within block based on statistics [6] . SAO classifies reconstructed samples into different categories and obtains an offset for each category. The offset for each category is sig- Fig. 1. Structure of residual block naled after calculation at the encoder. Reconstructed samples add the offset adaptively according to the class [17]. Adaptive loop filter targets at minimizing the mean square error between is much simpler to be captured by network. Therefore a global original samples and reconstructed samples by Wiener-based shortcut is appended to make the network focus on the residual adaptive filter [7] [18]. Different filters are applied to different and detail recovery. Inspired by ResNet [8], our proposed regions, and filter parameters are also transmitted to decoder. network is built by several residual blocks. As depicted in Fig. In order to save bits, the filter parameters of different regions 1, each residual block has two convolutional layers separated can be merged according to RDO. by Rectified Linear Units (ReLU). As aforementioned, the identity connection is utilized to accelerate learning process as B. Deep learning based loop filter well as restoring the details. As shown in Fig. 2, the network Majority of CNN based image restoration methods have architecture for luma components is a fully CNN composed emerged recently and obtained state-of-the-art performance. of three parts. The number of residual block determines the More specially for deep learning based in-loop filtering, on the depth of the whole network. one hand, the network architecture is turning into reasonable Human visual system (HVS) is more sensitive to the ad- and complicated gradually. Dai et al. [19] proposed a variable- justment of luminance, consequently chroma channels are filter-size residue-learning CNN which contained only four commonly downsampled to reduce the volume of data storage. convolution layers. Zhang et al. [20] explored more complex Since luminance accommodates much more details, it can be network. In [20], authors designed various types of high way guidance in the filtering of chroma component. YUV420 is unit and built a deep residual highway CNN based on this unit. a commonly used format in video coding. The height and To adapt to various image contents, Jia et al. [21] devoted to width of the chroma components are both half of the luma a content-aware multimodel filtering. Under the guidance of components in YUV420. In order to get three channels of the discriminative network, each CTU selected the optimal the same size, we upsample chroma components by nearest restoration model in this work. neighbor of each sample, which imports no additional artifacts. On the other hand, more priori knowledge shared by both The network architecture for chroma component is depicted in encoder and decoder was extracted from encoding process to Fig. 3. Residual blocks are main foundation of whole network offer better assistance. Jia et al. proposed a spatial-temporal structure as well. In particular, there are two branches of part1 residue network based in-loop filter in [21]. Current block in chrominance network architecture. Enlarged reconstructed and co-located block in reference frame were both taken into chroma channel and reconstructed luma channel are processed consideration and fed into network. He et al. [22] extracted by different network branches separately and then concaten- CU split partition and generated masks from it subsequently. tate. Afterwards, fused feature maps are handled by the same The authors in [22] further discussed fusion strategy of mask parts as previous network structure. It is worth to mention that and reconstructed frame. Li et al. [23] utilized difference an average pooling layer is put at the end to downsample the between reconstructed and predicted pixel as additional input size of feature maps. of
Details
-
File Typepdf
-
Upload Time-
-
Content LanguagesEnglish
-
Upload UserAnonymous/Not logged-in
-
File Pages5 Page
-
File Size-