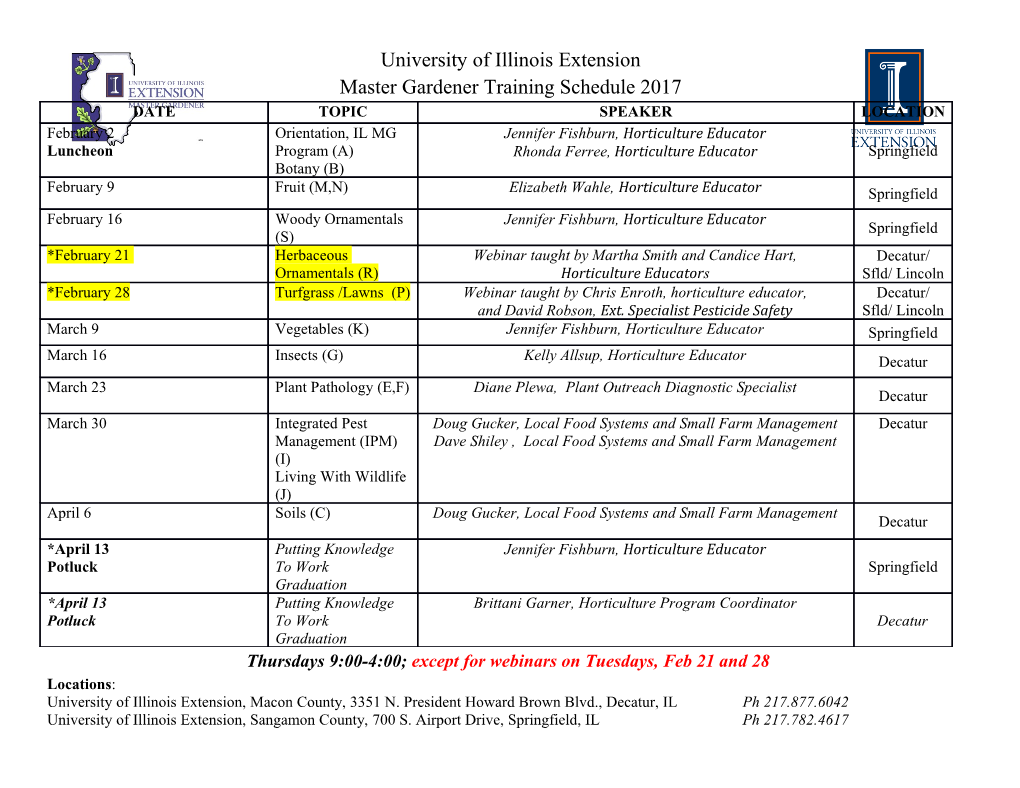
The MADAR Shared Task on Arabic Fine-Grained Dialect Identification Houda Bouamor, Sabit Hassan, Nizar Habashy Carnegie Mellon University in Qatar, Qatar yNew York University Abu Dhabi, UAE fhbouamor,[email protected] [email protected] Abstract Grained Dialect Identification. The shared task was organized as part of the Fourth Arabic Natural In this paper, we present the results and find- Language Processing Workshop (WANLP), collo- ings of the MADAR Shared Task on Ara- cated with ACL 2019.1 This shared task is the first bic Fine-Grained Dialect Identification. This shared task was organized as part of The to target a large set of dialect labels at the city and Fourth Arabic Natural Language Process- country levels. The data for the shared task was ing Workshop, collocated with ACL 2019. created under the Multi-Arabic Dialect Applica- The shared task includes two subtasks: the tions and Resources (MADAR) project.2 MADAR Travel Domain Dialect Identification The shared task featured two subtasks. First subtask (Subtask 1) and the MADAR Twit- is the MADAR Travel Domain Dialect Identifica- ter User Dialect Identification subtask (Sub- task 2). This shared task is the first to target a tion subtask (Subtask 1), which targeted 25 spe- large set of dialect labels at the city and coun- cific cities in the Arab World. And second is the try levels. The data for the shared task was cre- MADAR Twitter User Dialect Identification (Sub- ated or collected under the Multi-Arabic Di- task 2), which targeted 21 Arab countries. All of alect Applications and Resources (MADAR) the datasets created for this shared task will be project. A total of 21 teams from 15 countries made publicly available to support further research participated in the shared task. on Arabic dialect modeling.3 1 Introduction A total of 21 teams from 15 countries in four continents submitted runs across the two sub- Arabic has a number of diverse dialects from tasks and contributed 17 system description pa- across different regions of the Arab World. Al- pers. All system description papers are included though primarily spoken, written dialectal Arabic in the WANLP workshop proceedings and cited in has been increasingly used on social media. Auto- this report. The large number of teams and sub- matic dialect identification is helpful for tasks such mitted systems suggests that such shared tasks on as sentiment analysis (Al-Twairesh et al., 2016), Arabic NLP can indeed generate significant inter- author profiling (Sadat et al., 2014), and machine est in the research community within and outside translation (Salloum et al., 2014). Most previ- of the Arab World. ous work, shared tasks, and evaluation campaigns Next, Section2 describes the shared task sub- on Arabic dialect identification were limited in tasks. Section3 provides a description of the terms of dialectal variety targeting coarse-grained datasets used in the shared task, including the regional dialect classes (around five) plus Mod- newly created MADAR Twitter Corpus. Section4 ern Standard Arabic (MSA) (Zaidan and Callison- presents the teams that participated in each subtask Burch, 2013; Elfardy and Diab, 2013; Darwish with a high-level description of the approaches et al., 2014; Malmasi et al., 2016; Zampieri et al., they adopted. Section5 discusses the results of 2017; El-Haj et al., 2018). There are of course the competition. Finally, Section6 concludes this some recent noteworthy exceptions (Bouamor report and discusses some future directions. et al., 2018; Zaghouani and Charfi, 2018; Abdul- Mageed et al., 2018). 1http://wanlp2019.arabic-nlp.net In this paper, we present the results and find- 2https://camel.abudhabi.nyu.edu/madar/ ings of the MADAR Shared Task on Arabic Fine- 3http://resources.camel-lab.com. 2 Task Description Region Country City Gulf Yemen Sana’a The MADAR Shared Task included two subtasks: of Aden Djibouti the MADAR Travel Domain Dialect Identification Somalia subtask, and the MADAR Twitter User Dialect Gulf Oman Muscat Identification subtask. UAE 2.1 Subtask 1: MADAR Travel Domain Qatar Doha Dialect Identification Bahrain Kuwait The goal of this subtask is to classify written Ara- KSA Riyadh, Jeddah bic sentences into one of 26 labels representing the Iraq Baghdad, specific city dialect of the sentences, or MSA. The Mosul, Basra participants were provided with a dataset from the Levant Syria Damascus, Aleppo MADAR corpus (Bouamor et al., 2018), a large- Lebanon Beirut scale collection of parallel sentences in the travel Jordan Amman, Salt domain covering the dialects of 25 cities from the Palestine Jerusalem Arab World in addition to MSA (Table1 shows Nile Basin Egypt Cairo, Alexandria, the list of cities). This fine-grained dialect iden- Aswan tification task was first explored in Salameh et al. Sudan Khartoum (2018), where the authors introduced a system that Maghreb Libya Tripoli, Benghazi can identify the exact city with an averaged macro Tunisia Tunis, Sfax F1 score of 67.9%. The participants in this sub- Algeria Algiers task received the same training, development and Morocco Rabat, Fes test sets used in (Salameh et al., 2018). More de- Mauritania tails about this dataset are given in Section3. MSA 2.2 Subtask-2: MADAR Twitter User Dialect Table 1: The list of the regions, countries, and cities Identification covered in Subtask 1 (City column) and Subtask 2 The goal of this subtask is to classify Twitter user (Country column). profiles into one of 21 labels representing 21 Arab countries, using only the Twitter user tweets. The Twitter user profiles as well as the tweets are part (e.g., geo-location data) were not allowed. Sec- of the MADAR Twitter Corpus, which was cre- ond, participants were instructed not to include the ated specifically for this shared task. More details MADAR Twitter Corpus development set in train- about this dataset are given in Section3. ing. However, any publicly available unlabelled data could be used. 2.3 Restrictions and Evaluation Metrics We provided the participants with a set of restric- Evaluation Metrics Participating systems are tions for building their systems to ensure a com- ranked based on the macro-averaged F1 scores ob- mon experimental setup. tained on blind test sets (official metric). We also Subtask 1 Restrictions Participants were asked report performance in terms of macro-averaged not to use any external manually labeled datasets. precision, macro-averaged recall and accuracy However, the use of publicly available unlabelled at different levels: region (Accregion), country data was allowed. Participants were not allowed (Acccountry) and city (Acccity). Accuracy at to use the development set for training. coarser levels (i.e., country and region in Sub- task 1; and region in Subtask 2) is computed by Subtask 2 Restrictions First, participants were comparing the reference and prediction labels af- asked to only use the text of the tweets and the spe- ter mapping them to the coarser level. We follow cific information about the tweets provided in the the mapping shown in Table1. Each participating shared task (see Section 3.2). Additional tweets, team was allowed to submit up to three runs for external manually labelled data sets, or any meta each subtask. Only the highest scoring run was information about the Twitter user or the tweets selected to represent the team. 3 Shared Task Data Dialect Sentence Aleppo Q .ÈA®£@ è » ø YK. Next, we discuss the corpora used for the subtasks. Alexandria .ÈA®£@Q¯ñÊK. PðA« Algiers 3.1 The MADAR Travel Domain Corpus .ÈA®£@ ©K ñºK QKH. Ag ú G@P Amman In Subtask 1, we use a large-scale collection of .ÈA®£@Q¯ñÊK. ø YK. Aswan parallel sentences covering the dialects of 25 Arab .ÈA®£@ Q¯ñÊK. QK A« IJ» cities (Table1), in addition to English, French and Baghdad .ÈA®£@ ÈAÓ èQ YK P@ MSA (Bouamor et al., 2018). This resource was Basra .ÈAêk. èQ YK P@ Beirut Q a commissioned translation of the Basic Travel- .XBñÊË è » ø YK. ing Expression Corpus (BTEC) (Takezawa et al., Benghazi .ÈA®£@ ¨AJK. éJ ËAÓ úæK . 2007) sentences from English and French to the Cairo .ÈA®£@ Q¯ñÊK. QK A« Damascus different dialects. It includes two corpora. The .ÈA®£@ èQ» øYK. first consists of 2,000 sentences translated into 25 Doha .ÈAîE éÊKA ¯ IJ ªK . Fes Arab city dialects in parallel. We refer to it as Cor- .PAªË@ ø P@PYË@ ÈAK X é J.Ë IJ ªK. pus 26 (25 cities plus MSA). The second corpus Jeddah .ÈA®£@ èQ AK.@ has 10,000 additional sentences (non-overlapping Jerusalem .ÈA®£@ èPQk . øYK. with the 2,000 sentences) from the BTEC cor- Khartoum .ÈA®£ @Q¯ñÊK QK@X pus translated to the dialects of only five selected . Mosul .ÈA®£@ èQ YJ«@ cities: Beirut, Cairo, Doha, Tunis and Rabat. We Muscat Q refer to it as Corpus 6 (5 cities plus MSA). An .ÈA®£CË è AªK.@ Rabat .PAªË@ øP@PYË@ ÈAKX ñºKQK IJ ªK example of a 27-way parallel sentence (25 cities . Riyadh Q plus MSA and English) extracted from Corpus 26 .ÈA®£CË è ùªK.@ Salt is given in Table2. The train-dev-test splits of the . ÈA®£CË Q¯ñÊK. ø YK. Sana’a Q corpora are shown in Table3. Corpus 6 test set .ú ÍA®£@ è ú æ@ 4 was not included in the shared task. Sfax .XBðCË ÈñK QÓ I. m' Tripoli .PAª ¨AJÓ éJ ËAÓ ú æ.K 3.2 The MADAR Twitter Corpus Tunis .PAª ¨AJÓ ÈñK QÓ I. m' For Subtask 2, we created a new dataset, the MSA .ÈA®£CË IJ »Ag. YK P@ MADAR Twitter Corpus, containing 2,980 Twit- ter user profiles from 21 different countries. Table 2: An example from Corpus 26 for the English sentence ‘I’d like a children’s sweater.’ Corpus collection Inspired by the work of Mubarak and Darwish(2014) we collected a set Sentences * Variant Total of Twitter user profiles that reflects the way users Corpus 6 train 9,000 * 6 54,000 from different regions in the Arab World tweet.
Details
-
File Typepdf
-
Upload Time-
-
Content LanguagesEnglish
-
Upload UserAnonymous/Not logged-in
-
File Pages9 Page
-
File Size-