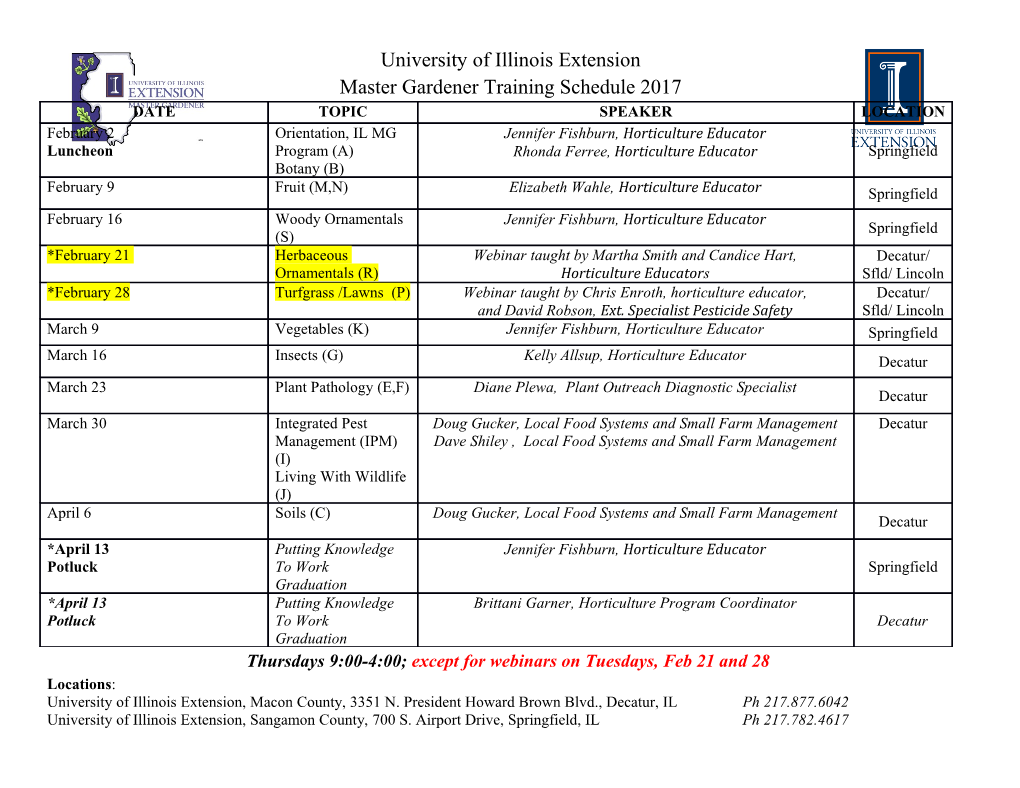
Conditional Expectation Andreas Klappenecker Texas A&M University © 2018 by Andreas Klappenecker. All rights reserved. 1 / 63 Conditional Expectation We are going to define the conditional expectation of a random variable given 1 an event, 2 another random variable, 3 a σ-algebra. Conditional expectations can be convenient in some computations. 2 / 63 Conditional Expectation given an Event Definition The conditional expectation of a discrete random variable X given an event A is denoted as ErX | As and is defined by ErX | As “ x PrrX “ x | As: x ¸ It follows that PrrX “ x and As ErX | As “ x PrrX “ x | As “ x : Pr A x x r s ¸ ¸ 3 / 63 By definition ErX | Y “ 1s “ 1 PrrX “ 1 | Y “ 1s ` 2 PrrX “ 2 | Y “ 1s: PrrX “ 1; Y “ 1s PrrX “ 2; Y “ 1s “ 1 ` 2 : PrrY “ 1s PrrY “ 1s Example Problem Suppose that X and Y are discrete random variables with values in t1; 2u s.t. 1 1 PrrX “ 1; Y “ 1s “ 2 ; PrrX “ 1; Y “ 2s “ 10 ; 1 3 PrrX “ 2; Y “ 1s “ 10 ; PrrX “ 2; Y “ 2s “ 10 : Calculate ErX | Y “ 1s: 4 / 63 Example Problem Suppose that X and Y are discrete random variables with values in t1; 2u s.t. 1 1 PrrX “ 1; Y “ 1s “ 2 ; PrrX “ 1; Y “ 2s “ 10 ; 1 3 PrrX “ 2; Y “ 1s “ 10 ; PrrX “ 2; Y “ 2s “ 10 : Calculate ErX | Y “ 1s: By definition ErX | Y “ 1s “ 1 PrrX “ 1 | Y “ 1s ` 2 PrrX “ 2 | Y “ 1s: PrrX “ 1; Y “ 1s PrrX “ 2; Y “ 1s “ 1 ` 2 : PrrY “ 1s PrrY “ 1s 4 / 63 1 1 3 We have PrrY “ 1s “ PrrX “ 1; Y “ 1s ` PrrX “ 2; Y “ 1s “ 2 ` 10 “ 5 . PrrX “ 1; Y “ 1s PrrX “ 2; Y “ 1s ErX | Y “ 1s “ 1 ` 2 PrrY “ 1s PrrY “ 1s 1{2 1{10 5 1 7 “ 1 ` 2 “ ` 2 “ 3{5 3{5 6 6 6 Example Problem Suppose that X and Y are discrete random variables with values in t1; 2u s.t. 1 1 PrrX “ 1; Y “ 1s “ 2 ; PrrX “ 1; Y “ 2s “ 10 ; 1 3 PrrX “ 2; Y “ 1s “ 10 ; PrrX “ 2; Y “ 2s “ 10 : 5 / 63 PrrX “ 1; Y “ 1s PrrX “ 2; Y “ 1s ErX | Y “ 1s “ 1 ` 2 PrrY “ 1s PrrY “ 1s 1{2 1{10 5 1 7 “ 1 ` 2 “ ` 2 “ 3{5 3{5 6 6 6 Example Problem Suppose that X and Y are discrete random variables with values in t1; 2u s.t. 1 1 PrrX “ 1; Y “ 1s “ 2 ; PrrX “ 1; Y “ 2s “ 10 ; 1 3 PrrX “ 2; Y “ 1s “ 10 ; PrrX “ 2; Y “ 2s “ 10 : 1 1 3 We have PrrY “ 1s “ PrrX “ 1; Y “ 1s ` PrrX “ 2; Y “ 1s “ 2 ` 10 “ 5 . 5 / 63 Example Problem Suppose that X and Y are discrete random variables with values in t1; 2u s.t. 1 1 PrrX “ 1; Y “ 1s “ 2 ; PrrX “ 1; Y “ 2s “ 10 ; 1 3 PrrX “ 2; Y “ 1s “ 10 ; PrrX “ 2; Y “ 2s “ 10 : 1 1 3 We have PrrY “ 1s “ PrrX “ 1; Y “ 1s ` PrrX “ 2; Y “ 1s “ 2 ` 10 “ 5 . PrrX “ 1; Y “ 1s PrrX “ 2; Y “ 1s ErX | Y “ 1s “ 1 ` 2 PrrY “ 1s PrrY “ 1s 1{2 1{10 5 1 7 “ 1 ` 2 “ ` 2 “ 3{5 3{5 6 6 6 5 / 63 Caveat This interpretation does not work for all random variables, but it gives a better understanding of the meaning of ErX | As. Conditional Expectation Interpretation Let F “ 2Ω with Ω finite. For a random variable X and an event A, we can interpret ErX | As as the average of X p!q over all ! P A. Indeed, we have PrrX “ x and As ErX |As “ x PrrX “ x | As “ x Pr A x x r s ¸ Prr!s ¸ “ X p!q : PrrAs !PA ¸ 6 / 63 Conditional Expectation Interpretation Let F “ 2Ω with Ω finite. For a random variable X and an event A, we can interpret ErX | As as the average of X p!q over all ! P A. Indeed, we have PrrX “ x and As ErX |As “ x PrrX “ x | As “ x Pr A x x r s ¸ Prr!s ¸ “ X p!q : PrrAs !PA ¸ Caveat This interpretation does not work for all random variables, but it gives a better understanding of the meaning of ErX | As. 6 / 63 Conditional Expectation Proposition We have ErXI s ErX | As “ A : PrrAs Proof. As we have seen, PrrX “ x and As 1 ErX |As “ x “ xPrrX “ x and As: Pr A Pr A x r s r s x ¸ ¸ We can rewrite the latter expression in the form ErXI s ErX |As “ A : PrrAs 7 / 63 Definition for General Random Variables Definition The conditional expectation ErX | As of an arbitrary random variable X given an event A is defined by ErXI s A if PrrAs ¡ 0; ErX |As “ PrrAs $ &0 otherwise: % 8 / 63 Properties 9 / 63 Linearity Proposition If a and b are real numbers and X and Y are random variables, then EraX ` bY | As “ aErX | As ` bErY | As: Proof. ErpaX ` bY q I s EraX ` bY | As “ A PrrAs ErXI s ErYI s “ a A ` b A PrrAs PrrAs “ aErX | As ` bErY | As: 10 / 63 Independence Proposition If X and Y are independent discrete random variables, then ErY | X “ xs “ ErY s: Proof. By definition, ErY | X “ xs “ y PrrY “ y | X “ xs y ¸ “ y PrrY “ ys “ ErY s: y ¸ 11 / 63 Important Application 12 / 63 Computing Expectations We can compute the expected value of X as a sum of conditional expectations. This is similar to the law of total probability. Proposition If X and Y are discrete random variables, then ErX s “ ErX | Y “ ys PrrY “ ys: y ¸ 13 / 63 Proposition If X and Y are discrete random variables, then ErX s “ ErX | Y “ ys PrrY “ ys: y ¸ Proof. ErX | Y “ ys PrrY “ ys “ x PrrX “ x|Y “ ys PrrY “ ys y y x ¸ ¸ ´ ¸ ¯ “ x PrrX “ x|Y “ ys PrrY “ ys x y ¸ ¸ “ x PrrX “ x; Y “ ys x y ¸ ¸ “ x PrrX “ xs “ ErX s x ¸ 14 / 63 Why We Need More than One Type of Conditional Expectation 15 / 63 Conditional Expectation We can also define conditional expectations for continuous random variables. Definition The conditional expectation of a discrete random variable Y given that X “ x is defined as ErY | X “ xs “ y PrrY “ y | X “ xs: y ¸ The conditional expectation of a continuous random variable Y given that X “ x is defined as 8 ErY | X “ xs “ y fY |X “x pyq dy; »´8 We assume absolute convergence in each case. 16 / 63 Motivating Example Problem A stick of length one is broken at a random point, uniformly distributed over the stick. The remaining piece is broken once more. Find the expected value of the piece that now remains. 17 / 63 Motivating Example Let X denote the random variable giving the length of the first remaining piece. Then X is uniformly distributed over the unit interval p0; 1q. Let Y denote the random variable giving the length of the second remaining piece. Then Y is uniformly distributed over the shorter interval p0; X q. 18 / 63 Motivating Example: Interpretation Given that X “ x, the random variable Y is uniformly distributed over the interval p0; xq. In other words, Y | X “ x has the density function 1 f pyq “ Y |X “x x for all y in p0; xq. 19 / 63 Example Since the random variable X is uniformly distributed over the interval p0; 1q, we have 1 ` 0 1 ErX s “ “ : 2 2 Motivating Example: Expectation For a random variable Z that is uniformly distributed on the interval pa; bq, we have b 1 1 1 b ErZs “ x dx “ x 2 b ´ a b ´ a 2 »a ˇa b2 ´ a2 b ` a ˇ “ “ : ˇ 2pb ´ aq 2 ˇ 20 / 63 Motivating Example: Expectation For a random variable Z that is uniformly distributed on the interval pa; bq, we have b 1 1 1 b ErZs “ x dx “ x 2 b ´ a b ´ a 2 »a ˇa b2 ´ a2 b ` a ˇ “ “ : ˇ 2pb ´ aq 2 ˇ Example Since the random variable X is uniformly distributed over the interval p0; 1q, we have 1 ` 0 1 ErX s “ “ : 2 2 20 / 63 Does this solve the problem? Now we know the expected length of the second remaining piece, given that we know the length x of the first remaining piece of the stick. Motivating Example Example Since Y |X “ x is uniformly distributed over p0; xq, we get x 1 x ` 0 x ErY | X “ xs “ y dy “ “ : x 2 2 »0 21 / 63 Motivating Example Example Since Y |X “ x is uniformly distributed over p0; xq, we get x 1 x ` 0 x ErY | X “ xs “ y dy “ “ : x 2 2 »0 Does this solve the problem? Now we know the expected length of the second remaining piece, given that we know the length x of the first remaining piece of the stick. 21 / 63 Motivating Example We can also define a random variable ErY | X s that satisfies ErY | X sp!q “ ErY | X “ X p!qs: We expect that 1 ErErY | X ss “ ErX {2s “ : 4 Now this solves the problem. The expected length of the remaining piece is 1/4 of the length of the stick. 22 / 63 Conditional Expectation given a Random Variable 23 / 63 Motivation Question How should we think about ErX | Y s? Answer Suppose that Y is a discrete random variable. If we observe one of the values y of Y , then the conditional expectation should be given by ErX | Y “ ys: If we do not know the value y of Y , then we need to contend ourselves with the possible expectations ErX | Y “ y1s; ErX | Y “ y2s; ErX | Y “ y2s;::: So ErX | Y s should be a σpY q-measurable random variable itself.
Details
-
File Typepdf
-
Upload Time-
-
Content LanguagesEnglish
-
Upload UserAnonymous/Not logged-in
-
File Pages70 Page
-
File Size-