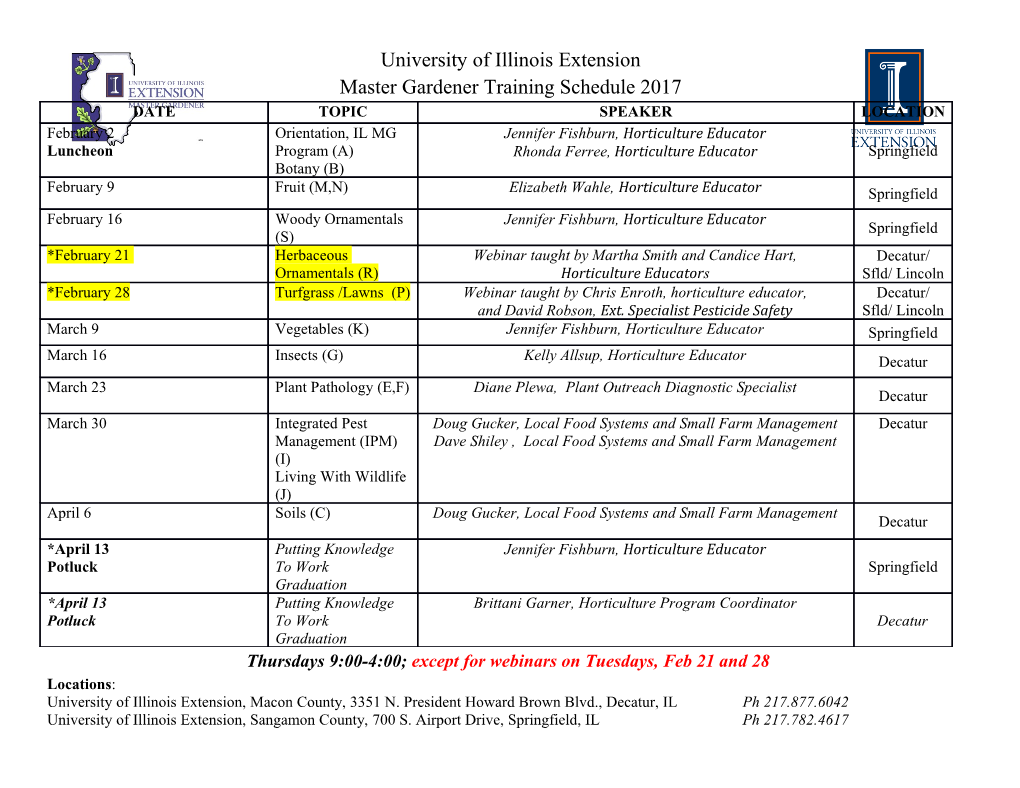
Feature Analytic Considerations for Repeated Measures of eGFR in Cohort Studies of CKD Haochang Shou,*† Jesse Y. Hsu,*† Dawei Xie,*† Wei Yang,*† Jason Roy,*† Amanda H. Anderson,*† J. Richard Landis,*† Harold I. Feldman,*† Afshin Parsa,‡§ and Christopher Jepson*† on behalf of the Chronic Renal Insufficiency Cohort (CRIC) Study Investigators Abstract Repeated measures of various biomarkersprovide opportunities forus toenhanceunderstanding ofmanyimportant *Department of clinical aspects of CKD, including patterns of disease progression, rates of kidney function decline under different Biostatistics, risk factors, and the degree of heterogeneity in disease manifestations across patients. However, because of unique Epidemiology and features, such as correlations across visits and time dependency, these data must be appropriately handled using Informatics and †Center for Clinical longitudinal data analysis methods. We provide a general overview of the characteristics of data collected in cohort Epidemiology and studies and compare appropriate statistical methods for the analysis of longitudinal exposures and outcomes. We Biostatistics, Perelman use examples from the Chronic Renal Insufficiency Cohort Study to illustrate these methods. More specifically, we School of Medicine, model longitudinal kidney outcomes over annual clinical visits and assess the association with both baseline and University of longitudinal risk factors. Pennsylvania, Philadelphia, Clin J Am Soc Nephrol 12: 1357–1365, 2017. doi: https://doi.org/10.2215/CJN.11311116 Pennsylvania; ‡Department of Medicine, Division of Nephrology, Introduction In this paper, we will focus primarily on the appro- University of The term repeated measures refers to data observed priate statistical methods for analyzing longitudinal data Maryland School of repeatedly within the same subject, and they are being as outcomes. In particular, we will discuss exten- Medicine, Baltimore, Maryland; and increasingly collected in many research studies. Aside sively regression methods that can estimate the rate of § from being used to evaluate the reproducibility and Department of change of the longitudinal data in association with Medicine, Baltimore variability of a novel biomarker (1,2), repeated mea- certain risk factors (10,11). The terms repeated mea- Veterans Affairs sures are often generated in the context of longitudinal sures and longitudinal data are used interchangeably. Medical Center, studies, in which one or more biomarkers are ob- Baltimore, Maryland served over time. For chronic diseases, such as CKD, patterns of biomarker trajectories are crucial for un- Motivating Example Correspondence: derstanding of disease prognosis. The Chronic Renal Insufficiency Cohort (CRIC) Study Dr. Haochang Shou, Department of In many studies, the repetitions are predetermined by is a prospective, longitudinal study of patients with CKD, Biostatistics, the study protocol, whereby measures are administered in which repeated measures of serum creatinine and Epidemiology and prospectively at specific intervals during scheduled cystatin C, along with several other laboratory measures, Informatics, Perelman clinical visits or telephone interviews (3,4). In other were collected from each participant during annual School of Medicine, University of scenarios, the repeated data (e.g.,measuresofvital clinical visits (3,7,12). eGFR, calculated on the basis of Pennsylvania, 219 signs, such as BP, heart rate, and respiratory rate) the CRIC Study eGFR equation that incorporates both Blockley Hall, 423 become available at variable time points when certain serum creatinine and cystatin C (13), is an important Guardian Drive, events (e.g., hospitalization) occur. Repeated measures measure of kidney function (12). Describing the rate Philadelphia, PA could also be obtained retrospectively as natural history of change and patterns of eGFR decline as well as 19104.Email: hshou@ mail.med.upenn.edu data through available databases, such as Medicare (5). identifying risk factors that affect CKD progression are Depending on the scientific questions, the longitu- of particular interest in CKD research (10,14,15). dinal measures may serve as the outcomes of interest, Our motivating example involves the effect of func- the exposures, or a combination of both. Examples of tional kidney risk variants in the gene coding for APOL1 these scenarios in kidney disease research include ontherateofeGFRdeclineamongtheCRICStudy comparisons of the burden of coronary artery calcifi- participants. Two haplotypes (G1 and G2) in APOL1 cation for patients at different stages of CKD and have been positively selected and are common in ESRD, treating the longitudinal coronary artery calci- populations of recent African continental descent, but fication measures as the outcome of interest (6); they are very rare or absent in most other populations, evaluations of the associations of longitudinal mea- where exposure to Trypanosomes was not common. sures of GFR with subsequent adverse events, such as These APOL1 variants associated with kidney diseases are ESRDanddeath,asoutcomes(7,8);andaninvesti- believed to account for much of the nonsocioeconomic- gation of the causal relationship between BP and based related disparity in rates of CKD progression kidney function, in which both measures were up- between patients with African ancestry and white dated over time (9). patients. www.cjasn.org Vol 12 August, 2017 Copyright © 2017 by the American Society of Nephrology 1357 1358 Clinical Journal of the American Society of Nephrology DNA samples from the 1411 African ancestry participants fasterdeclinecomparedwithothers.Second,suchasinmost enrolled in the CRIC Study between June of 2003 and August of cohort studies, subjects have varying numbers of visits. Third, 2008 were genotyped for APOL1 risk variants (16). Given the the baseline eGFR values differ across subjects. near absence of these APOL1 risk variants in whites, the In the era of big data, many multivariable measures, such exposure variable was defined in conjunction with race into as imaging scans, proteomics assessments, and electronic three categories: APOL1 high-risk genotype (African ancestry health records (6,19,20), are collected at multiple visits for participants with two copies of the risk variants), APOL1 low- large cohorts of participants. It might no longer be feasible risk genotype (African ancestry participants with zero or one to include all of the measures in one data frame. Utilization of copy of these variants), and white participants (reference group). high-performance computing and central databases, such as The investigators were interested in assessing whether the National Institute of Diabetes and Digestive and Kidney rates of eGFR decline differ among the three exposure Diseases Repository (21,22), that crosslink various measures groups. The longitudinal outcome in this example was the to a unique subject-visit identity is crucial to handle complex annual eGFR measures for each participant for up to 7 years and heterogeneous data. In addition, advanced statistical after enrollment. Other covariates included demographics tools, including dynamic visualization interface and hierar- (e.g.,age,sex,andclinicalsite),socioeconomicvariables chical clustering visualization using graph structures (23,24), (e.g., income and education level), and traditional clinical need to be leveraged to CKD research involving big data risk factors (e.g., systolic BP and body mass index) and were (25–28). mostly observed at baseline (16). Time Dependency and Correlated Observations Data Preparation and Visualization Longitudinal data have unique and crucial characteris- For longitudinal studies of moderate size, the dataset is often tics. First, they are typically accompanied by a time variable prepared in either of the two ways: wide format (one row of that indicates when each measurement occurred, and they data per participant) or long format (multiple rows of data per define a natural ordering of the repeated measures within participant; one per visit) (17) (details are in Supplemental each subject. Second, the repeated measures within the Appendix 1). The long format is generally more preferred for same subject are potentially correlated. For example, within advanced statistical modeling, because it can handle subjects each subject, the eGFR values of subsequent visits might with different numbers of clinical visits or irregular time points depend on those at earlier visits. Such intrinsic clusters of measurement; it is also easier for dynamically updating the defined by subjects result in dependency (correlation) dataset with future follow-up visits. among repeated observations, which violates the assump- Exploratory analysis using graphs can help researchers to tion of independent observations on which many simple frame the hypothesis and select appropriate statistical models. analytic methods are based. Hence, using traditional linear Some commonly used visualization tools for longitudinal data regression and ignoring data correlations might lead to include spaghetti plot, heat map, and lasagna plot (18) (Figures inaccurate estimates and erroneous inferences about the 1 and 2). These plots show several features of the eGFR associations of risk factors with kidney function decline trajectories. First, patients with APOL1 high risk seem to have a (29). Figure 1. | Spaghetti plot of eGFR over time for 15 random Chronic Renal Insufficiency Cohort (CRIC) Study participants. The figure shows an example
Details
-
File Typepdf
-
Upload Time-
-
Content LanguagesEnglish
-
Upload UserAnonymous/Not logged-in
-
File Pages9 Page
-
File Size-