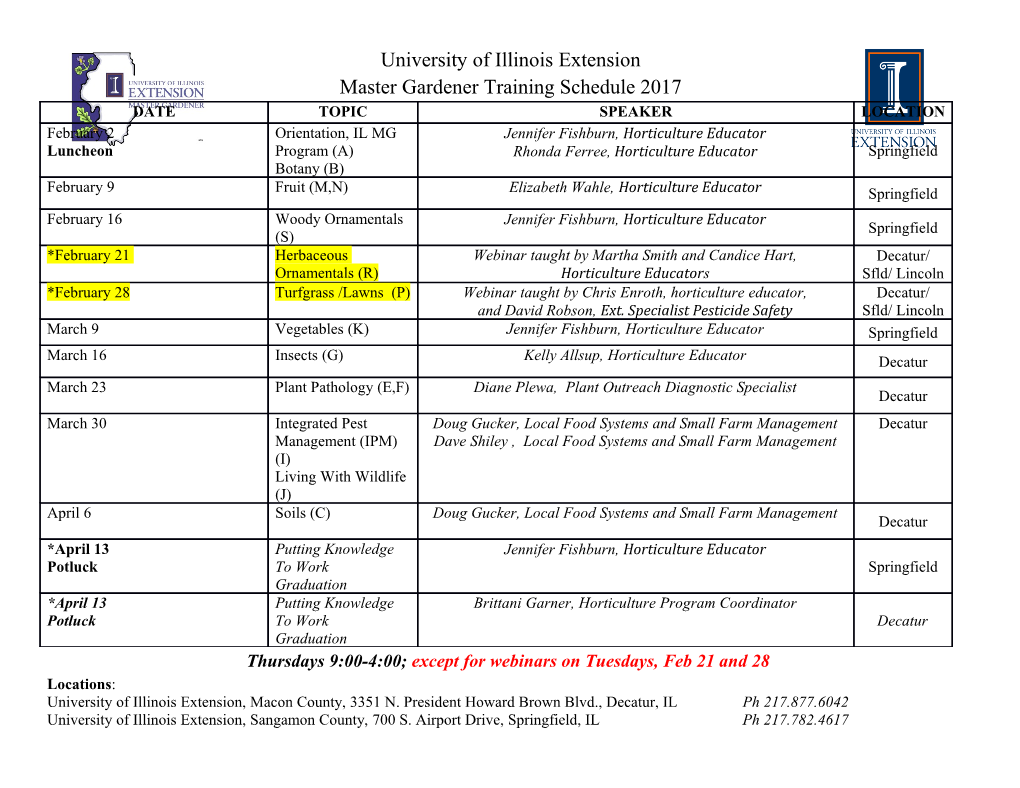
The Bayesian Perspective in the Context of Large Scale Assessments David Kaplan Department of Educational Psychology 17 February 2012 Introduction ❖ Introduction ● 1. Introduction to The research reported in this paper was supported by the Institute of Bayesian Inference Education Sciences, U.S. Department of Education, through Grant 2. An Example: R305D110001 to The University of Wisconsin - Madison. The Multilevel SEM applied to PISA opinions expressed are those of the authors and do not represent 3. Discussion views of the Institute or the U.S. Department of Education. Indiana University - WIM Symposium 2 / 55 ❖ Introduction Probability does not exist. 1. Introduction to Bayesian Inference 2. An Example: - Bruno de Finetti Multilevel SEM applied to PISA 3. Discussion Indiana University - WIM Symposium 3 / 55 ❖ Introduction ● 1. Introduction to Bruno de Finetti was an Italian probabilist and one of the most Bayesian Inference important contributors to the subjectivist Bayesian movement. 2. An Example: Multilevel SEM applied to PISA ● 3. Discussion His statement can be considered the foundational view of the subjectivist branch of Bayesian statistics. ● My goal is to argue for a subjectivist Bayesian approach to conducting research using data from large scale assessments (LSAs). Indiana University - WIM Symposium 4 / 55 ❖ Introduction OUTLINE 1. Introduction to Bayesian Inference 2. An Example: Multilevel SEM applied 1. Introduction to Bayesian inference to PISA 3. Discussion 2. An Example: Multilevel SEM applied to PISA 3. Discussion Indiana University - WIM Symposium 5 / 55 ❖ Introduction 1. Introduction to Bayesian Inference ❖ Frequentist v. Bayesian Probability ❖ Bayes’ Theorem ❖ The Prior Distribution ❖ Exchangeability ❖ Where do priors come from? ❖ The Likelihood ❖ The Posterior Distribution 1. Introduction to Bayesian Inference ❖ Bayesian Model Evaluation and Testing ❖ Implications for ILSAs 2. An Example: Multilevel SEM applied to PISA 3. Discussion Indiana University - WIM Symposium 6 / 55 Frequentist v. Bayesian Probability ❖ Introduction ● 1. Introduction to For frequentists, the basic idea is that probability is represented by Bayesian Inference the model of long run frequency. ❖ Frequentist v. Bayesian Probability ❖ Bayes’ Theorem ❖ The Prior Distribution ● Frequentist probability underlies the Fisher and Neyman-Pearson ❖ Exchangeability ❖ Where do priors come schools of statistics – the conventional methods of statistics we most from? often use. ❖ The Likelihood ❖ The Posterior Distribution ❖ Bayesian Model ● Evaluation and Testing The frequentist formulation rests on the idea of equally probable and ❖ Implications for ILSAs stochastically independent events 2. An Example: Multilevel SEM applied to PISA ● The physical representation is the coin toss, which relates to the idea 3. Discussion of a very large (actually infinite) number of repeated experiments. Indiana University - WIM Symposium 7 / 55 ❖ Introduction ● 1. Introduction to The entire structure of Neyman - Pearson hypothesis testing is Bayesian Inference based on frequentist probability. ❖ Frequentist v. Bayesian Probability ❖ Bayes’ Theorem ❖ The Prior Distribution ● Our conclusions regarding the null and alternative hypotheses ❖ Exchangeability ❖ Where do priors come presuppose the idea that we could conduct the same experiment an from? infinite number of times. ❖ The Likelihood ❖ The Posterior Distribution ❖ Bayesian Model ● Evaluation and Testing Our interpretation of confidence intervals also assumes a fixed ❖ Implications for ILSAs parameter and CIs that vary over an infinitely large number of 2. An Example: identical experiments. Multilevel SEM applied to PISA 3. Discussion Indiana University - WIM Symposium 8 / 55 ❖ Introduction ● 1. Introduction to But there is another view of probability as subjective belief. Bayesian Inference ❖ Frequentist v. Bayesian Probability ● The physical model in this case is that of the “bet”. ❖ Bayes’ Theorem ❖ The Prior Distribution ❖ Exchangeability ● ❖ Where do priors come Consider the situation of betting on who will win the World Series. from? ❖ The Likelihood ❖ The Posterior ● Distribution Here, probability is not based on some notion of an infinite number ❖ Bayesian Model of repeatable and stochastically independent events, but rather Evaluation and Testing ❖ Implications for ILSAs some notion of how much knowledge you have and how much you 2. An Example: are willing to bet. Multilevel SEM applied to PISA 3. Discussion ● Thus, subjective probability allows one to address questions such as “what is the probability that the Cubs will win the World Series?” Relative frequency supplies information, but it is not the same as probability and can be quite different. ● This notion of subjective probability underlies Bayesian statistics. Indiana University - WIM Symposium 9 / 55 Bayes' Theorem ❖ Introduction ● 1. Introduction to Consider the joint probability of two events, Y and X, for example Bayesian Inference observing smoking and lung cancer jointly. ❖ Frequentist v. Bayesian Probability ❖ Bayes’ Theorem ❖ The Prior Distribution ● The joint probability can be written as ❖ Exchangeability ❖ Where do priors come from? p(Y,X) = p(Y |X)p(X) (1) ❖ The Likelihood ❖ The Posterior Distribution ❖ Bayesian Model Evaluation and Testing ● Similarly ❖ Implications for ILSAs 2. An Example: Multilevel SEM applied p(X, Y ) = p(X|Y )p(Y ) (2) to PISA 3. Discussion Indiana University - WIM Symposium 10 / 55 ❖ Introduction ● 1. Introduction to Because these are symmetric, we can set them equal to each other Bayesian Inference to obtain the following ❖ Frequentist v. Bayesian Probability ❖ Bayes’ Theorem p(Y |X)p(X) = p(X|Y )p(Y ) (3) ❖ The Prior Distribution ❖ Exchangeability Therefore ❖ Where do priors come from? p(X|Y )p(Y ) ❖ The Likelihood p(Y |X) = (4) ❖ The Posterior p(X) Distribution ❖ Bayesian Model Evaluation and Testing and the inverse probability theorem (Bayes’ theorem) states ❖ Implications for ILSAs 2. An Example: p(Y |X)p(X) Multilevel SEM applied p(X|Y ) = (5) to PISA p(Y ) 3. Discussion ● Why do we care? Because this is how you could go from the probability of having cancer given that the patient smokes, to the probability that the patient smokes given that he/she has cancer. ● We simply need the marginal probability of smoking and the marginal probability of cancer (what we will call prior probabilities). Indiana University - WIM Symposium 11 / 55 ❖ Introduction ● 1. Introduction to A fundamental difference (among many) between Bayesians and Bayesian Inference frequentists, concerns the nature of parameters. ❖ Frequentist v. Bayesian Probability ❖ Bayes’ Theorem ● ❖ The Prior Distribution Frequentists view parameters as unknown fixed characteristics of a ❖ Exchangeability population, estimated from sample data. ❖ Where do priors come from? ❖ The Likelihood ❖ The Posterior ● Bayesians view parameters as unknown but random quantities that Distribution ❖ Bayesian Model can be characterized by a distribution. Evaluation and Testing ❖ Implications for ILSAs ● 2. An Example: In fact, Bayesians view all unknowns as possessing probability Multilevel SEM applied to PISA distributions. 3. Discussion ● This is a fundamental difference! It has implications for estimation. Indiana University - WIM Symposium 12 / 55 ❖ Introduction ● 1. Introduction to Because Bayesians view parameters probabilistically, we need to Bayesian Inference assign them probability distributions. ❖ Frequentist v. Bayesian Probability ❖ Bayes’ Theorem ● Consider again Bayes’ theorem in light of data and parameters. ❖ The Prior Distribution Since everything is random possessing a probability distribution, we ❖ Exchangeability ❖ Where do priors come can write Bayes’s theorem as from? ❖ The Likelihood p(Y |θ)p(θ) ❖ The Posterior p(θ|Y ) = (6) Distribution p(Y ) ❖ Bayesian Model Evaluation and Testing ❖ Implications for ILSAs where p(θ|Y ) is the posterior distribution of θ given the data Y , p(θ) 2. An Example: is the prior distribution of θ, and p(Y ) is the marginal distribution of Multilevel SEM applied the data. to PISA 3. Discussion ● Note that the marginal distribution p(Y ) does not involve model parameters. It is there to normalize the probability so that it integrates to 1.0. As such, we can ignore it and write Bayes’ theorem as p(θ|Y ) ∝ p(Y |θ)p(θ) (7) Indiana University - WIM Symposium 13 / 55 ❖ Introduction ● 1. Introduction to Let’s look at Bayes’ theorem again. Bayesian Inference ❖ Frequentist v. p(θ|Y ) ∝ p(Y |θ)p(θ) (8) Bayesian Probability ❖ Bayes’ Theorem ❖ The Prior Distribution ❖ Exchangeability ❖ Where do priors come ● This simple expression reflects a view about the evolutionary from? ❖ The Likelihood development of knowledge. It says ❖ The Posterior Distribution ❖ Bayesian Model Current knowledge ∝ Current evidence/data × Prior knowledge Evaluation and Testing ❖ Implications for ILSAs 2. An Example: ● The problem lies in what we consider to be prior knowledge. Multilevel SEM applied to PISA ● Frequency is not enough. We use frequency as a piece of 3. Discussion information to inform a subjective experience of uncertainty which we quantify as probability. ● Probability does not exist as a feature of the external world. It is a subjective quantification of uncertainty. This is the essence of de Finetti’s quote. Indiana University - WIM Symposium 14 / 55 The Prior Distribution ❖ Introduction ● 1. Introduction to A critically important part of Bayesian statistics is the prior Bayesian Inference distribution. ❖ Frequentist v. Bayesian Probability
Details
-
File Typepdf
-
Upload Time-
-
Content LanguagesEnglish
-
Upload UserAnonymous/Not logged-in
-
File Pages55 Page
-
File Size-