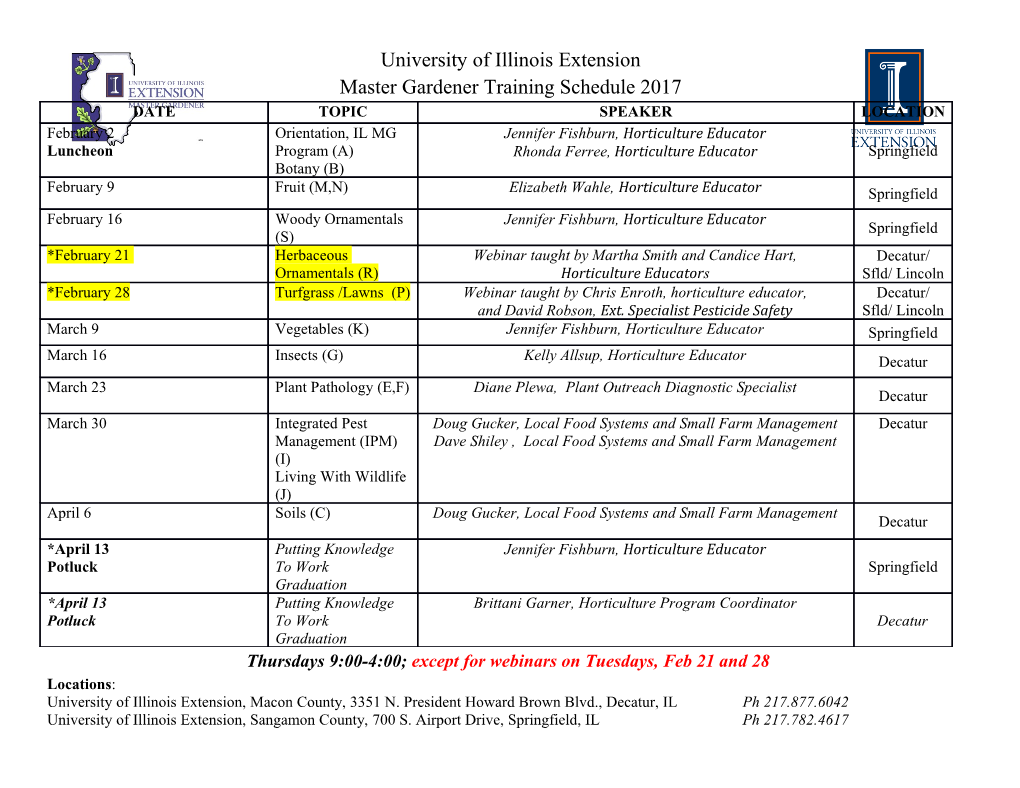
Development of pseudonymised matching methods for linking administrative datasets Pete Jones Office for National Statistics The Beyond 2011 Programme • Office for National Statistics (ONS) conducted a review (Beyond 2011 Programme) for the future approach to the census and population statistics in England and Wales • National Statistician made a recommendation to Government in March 2014 that there should be a predominantly online census in 2021 • This will be supplemented with increased use of administrative data and surveys to enhance census outputs and annual statistics • Part of our research leading up to the recommendation was to explore an administrative data option for producing population statistics • Involved the development of algorithms to link pseudonymised administrative datasets and surveys • Work continues within the 2021 Census Transformation Programme (Beyond 2021 Research & Development Team) Pseudonymised Linkage Model • There are additional challenges associated with the use of admin data • Data quality – particularly lags in data being up to date • Efficiency – need to match datasets with 60 million + records • Public acceptability - ONS unique in holding multiple admin sources in one place • Made the decision that names, dates of birth and addresses will be anonymised with a hashing algorithm (SHA-256) • Converts original identifiers into meaningless hashed values (e.g. John hashes to XY143257461) • Consistently maps same entities to the same hashed value Methodological Developments • Hashing data makes many of the traditional methods for resolving inconsistencies redundant - Cannot run direct string comparison algorithms - Cannot use clerical resolution • Developed alternative ways of tackling data capture inconsistencies (1) The development of match-keys that can be derived in pre- processing and hashed before linking two datasets Pseudonymised Match-Keys Unique records on Key Type EPR (%) 1 Forename, Surname, DoB, Sex, Postcode 100.00% 2 Forename initial , Surname initial, DoB, Sex, Postcode District 99.55% 3 Forename bi-gram, Surname bi-gram, DoB, Sex, Postcode Area 99.44% 4 Forename initial, DoB, Sex, Postcode 99.84% 5 Surname initial, DoB, Sex, Postcode 99.44% 6 Forename, Surname, Age, Sex, Postcode Area 99.46% 7 Forename, Surname, Sex, Postcode 99.19% 8 Forename, Surname, DoB, Sex 98.87% 9 Forename, Surname, DoB, Postcode 99.52% 10 Surname, Forename, DoB, Sex, Postcode (matched on key 1) 100.00% 11 Middle name, Surname, DoB, Sex, Postcode (matched on key 1) 99.90% (2) Similarity Tables • Constructing during pre-processing to support score-based methods that involve string comparison • Non-disclosive to match single variables in isolation prior to encryption Reception Server (Data Import Area) Data Storage Area Original Dataset (Source 2) Forename Surname DoB PostCode John Davis 02/04/1993 B1 2TG John Thomas 23/07/1986 M2 1JH John Smith 16/06/2003 BH12 1LT List of Jon Reed 19/09/1993 DT8 4PB unique Jon Ellis 16/06/2008 KT1 1LL John Extract list of Jonny Johnson 06/01/2002 N7 4ER Jon unique Jonny Daniels 21/10/1949 LN22 1AR Jonny forenames Jonny Barker 14/10/1974 PO11 7TG Jonathan Jonny King 26/02/1998 SO1 4KW …… Jonathan Khan 03/06/1999 E1 2BB Jonathan Wright 11/10/2004 CR21 2JJ Jonathan Walker 10/07/2002 W5 6AD … … … … Similarity Tables • Follow the same process for the 2nd dataset import Reception Server (Data Import Area) Data Storage Area Source 2 Dataset Forename Surname DoB PostCode John Davis 02/04/1993 B1 2TG John Thomas 23/07/1986 M2 1JH John Smith 16/06/2003 BH12 1LT List of unique Jon Reed 19/09/1993 DT8 4PB forenames Jon Ellis 16/06/2008 KT1 1LL Identify any John Jonny Johnson 06/01/2002 N7 4ER additional Jon Jonny Daniels 21/10/1949 LN22 1AR names not on Jonny Jonnie Barker 14/10/1974 PO11 7TG list Jonathan Jonny King 26/02/1998 SO1 4KW Jonnie Jonathan Khan 03/06/1999 E1 2BB …… Jonathan Wright 11/10/2004 CR21 2JJ Jonathan Walker 10/07/2002 W5 6AD … … … … Similarity Tables • Run string comparison algorithm between all names on the list List of String comparison List of unique Forename Matches Score unique algorithm forenames John John 1 John John John Jonny 0.88 Jon Jon John Jon 0.91 Jonny Jonny John Jonathan 0.82 Jonathan Jonathan Jonny Jonny 1 Jonnie Jonnie Jonny John 0.88 …… …… Jonny Jon 0.89 Jonny Jonathan 0.79 Jon Jon 1 Jon John 0.91 Jon Jonny 0.89 Jon Jonathan 0.81 Jonathan Jonathan 1 Jonathan John 0.82 Jonathan Jon 0.81 Jonathan Jonny 0.79 Similarity Tables (example) PR_Forename PR_Surname PR_DoB PR_Sex PR_Pcode SC_Forename SC_Surname SC_DoB SC_Sex SC_Pcode Jon Smyth 13/02/1965 M PO15 5RR John Smith 09/02/1965 M PO15 5RR PR_Forename SC_Forename Similarity Score PR_Surname SC_Surname Similarity Score PR_DoB SC_DoB Similarity Score John John 1 Smith Smyth 0.93 13/02/1965 08/02/1965 0.67 John Jonny 0.88 Smith Smithers 0.87 13/02/1965 09/02/1965 0.67 John Jon 0.91 Smith Smithson 0.85 13/02/1965 10/02/1965 0.67 John Jonathan 0.82 Smith Smith 1 13/02/1965 11/02/1965 0.67 Jonny Jonny 1 Smyth Smith 0.93 13/02/1965 12/02/1965 0.67 Jonny John 0.88 Smyth Smithers 0.9 13/02/1965 13/02/1965 1 Jonny Jon 0.89 Smyth Smithson 0.83 13/02/1965 14/02/1965 0.67 Jonny Jonathan 0.79 Smyth Smyth 1 13/02/1965 15/02/1965 0.67 Jon Jon 1 Smithers Smith 0.87 13/02/1965 16/02/1965 0.67 Jon John 0.91 Smithers Smyth 0.9 13/02/1965 17/02/1965 0.67 Jon Jonny 0.89 Smithers Smithson 0.92 13/02/1965 18/02/1965 0.67 Jon Jonathan 0.81 Smithers Smithers 1 13/02/1965 13/01/1965 0.67 Jonathan Jonathan 1 Smithson Smith 0.85 13/02/1965 13/03/1995 0.67 Jonathan John 0.82 Smithson Smyth 0.83 13/02/1965 13/04/1995 0.67 Jonathan Jon 0.81 Smithson Smithers 0.92 13/02/1965 13/05/1995 0.67 Jonathan Jonny 0.79 Smithson Smithson 1 13/02/1965 13/06/1995 0.67 … … … … … … … … … Candidate Matches ames • The similarity tables identify all the candidate pairs that achieve a specified similarity threshold on forename, surname and DoB Source 1 Source 2 Forename Source 1 Source 2 Surname Source 1 Source 2 Source 1 Overall Forename Forename Score Surname Surname Score DoB DoB DoB Score EFIJ2465 ZASG1635 0.78 CTYG0289 XHDK5456 0.93 GXCX6714 AFIQ8834 0.33 0.68 EFIJ2465 VRXM2613 0.91 CTYG0289 XHDK5456 0.93 GXCX6714 LRQP3671 0.67 0.84 EFIJ2465 HDNR3167 0.69 CTYG0289 CTYG0289 1 GXCX6714 EYGI9391 0.33 0.67 • The researcher will only ever see the hashed fields • Hashed variables are now redundant (can delete them) • The only usable information is the scores themselves • But what do you do with the scores? Training models with clerical review • Impractical to rely on clerical review when linking datasets at national level • Clerical review is redundant when records are hash encoded • ONS have arrangements to re-identify hashed values for small samples of match candidates for clerical review (approx. 1000 records) • Clerically matched samples can then be used as the basis of supervised matching models • Logistic regression has produced good results - Dependent variable is the clerical decision (binary Y/N) - Covariates are the similarity metrics (name / dob) , name commonality, geographic distances • Logistic regression provides a single threshold for designating matches / non-matches (p = 0.5) Match Classification with Logistic Regression t tables Classification Tablea Observed Predicted Match Percentage No Yes Correct Match No 78 3 96.3 Yes 2 283 99.3 Overall Percentage 98.6 a. The cut value is .500 Testing the Algorithms • Undertook record level comparison with links made by the 2011 Census QA Team (used exact / probabilistic / clerical matching) • Linked 1% of sample of records from the NHS Patient Register to the 2011 Census Comparison of PR to Census/CCS match rates: Census QA and B2011 100% 90% 80% 70% 60% 50% Census QA match rate 40% B2011 match rate 30% B2011 Precision 20% B2011 Recall 10% 0% Summary Tables Pseudonymised Pseudonymised Census QA Pseudonymised false positive false negative Local Authority match rate match rate rate rate Birmingham 82.0% 81.0% 0.4% 1.7% Westminster 65.1% 64.2% 0.4% 1.9% Lambeth 64.0% 63.5% 0.8% 1.6% Newham 68.3% 67.1% 0.5% 2.2% Southwark 66.3% 65.0% 0.4% 2.3% Powys 94.3% 93.4% 0.2% 1.2% Aylesbury Vale 89.9% 89.6% 0.3% 0.6% Mid Devon 88.6% 88.6% 0.2% 0.2% Total 72.7% 71.7% 0.5% 1.9% Pseudonymised Pseudonymised Census QA Pseudonymised false positive false negative Local Authority Type match rate match rate rate rate City Local Authorities 71.3% 70.3% 0.5% 2.0% Rural Local Authorities 91.2% 90.7% 0.3% 0.8% Total 72.7% 71.7% 0.5% 1.9% Further research • Causes of false negatives – developing new blocking strategies to identify a higher number of match candidates • Testing the use of unsupervised probabilistic matching - Fellegi-Sunter framework - EM algorithm for match probabilities - Duplicate link method for threshold setting (Blakely & Salmond 2002) • Exploring its potential application in 2021 Census to Coverage Survey Matching • Method to be used in the new Admin Data Research Centre (led by Southampton University) • Looking to collaborate with other NSIs and government departments .
Details
-
File Typepdf
-
Upload Time-
-
Content LanguagesEnglish
-
Upload UserAnonymous/Not logged-in
-
File Pages15 Page
-
File Size-