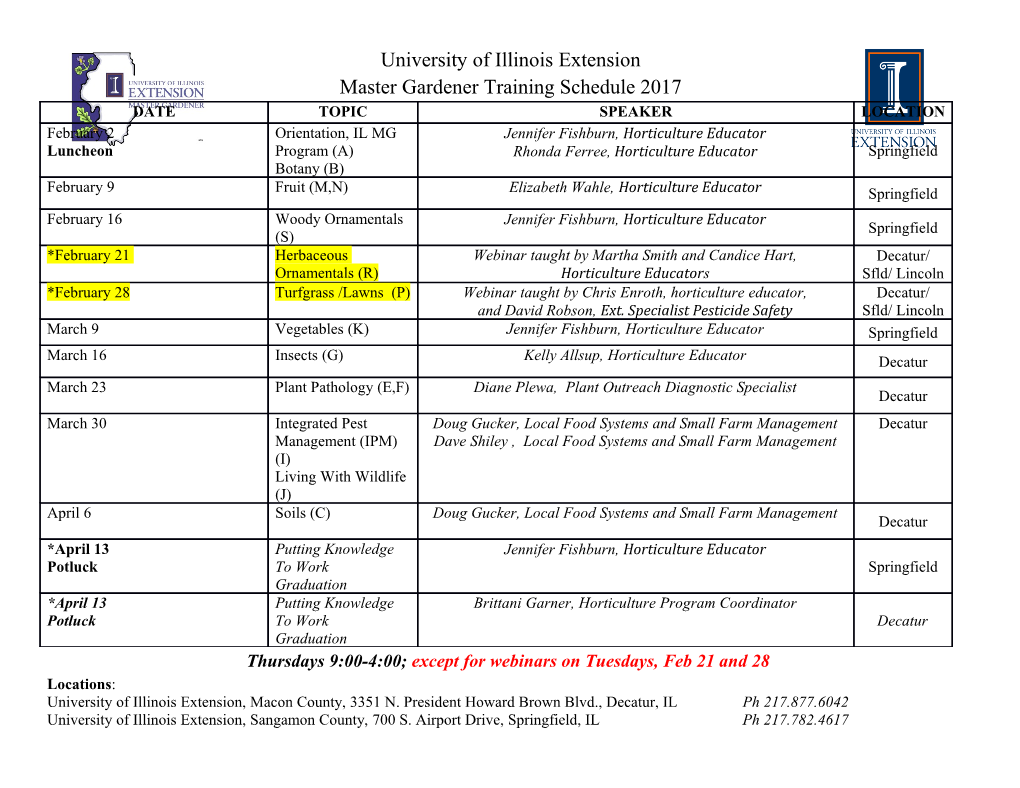
Analyzing∧complex binary data using SAS (by a Non- statistician) Jaswant Singh Veterinary Biomedical Sciences Most researchers use statistics that way a drunkard uses a lamp-post –more for support than illumination - Winfred Castle Stat 101 Dependent Variable (Outcome) Independent Variables (Predictor) Covariates (Confounders) Variable types: – Categorical (Qualitative) • Nominal, Dichotomous, Ordinal/count – Continuous (Quantitative) Fixed versus random factors First thing first….. What is the primary question that I am going to answer? Are the any secondary questions? Understand your Model: – What is my dependent variable? – What is/are my independent variables? – What is the type of data? Are there any confounders (covariates) Simplest Scenario Response variable: Binary Independent variable: Categorical e.g. My Dean would like to know: Does the Mclean’s Prestige rating of an Institution matters for admission into graduate program at UofS? Let’s generate a Frequency Table Prestige High Low Rejected 125 148 Admitted 87 40 Number of students Number 2x2 contingency table Chi-square test Chi-Square test Data Grad; Input Prestige$ Admission$ number; Cards; 1 Rejected 125 1 Admitted 87 2 Rejected 148 2 Admitted 40 Proc freq; Weight number; Tables Prestige*admission/chisq exact nocol norow; Run; Chi-Square: P-value=0.001 Dean got interested but…. Now want us to test Institutional Prestige Rating on 1 to 4 scale (best to worst) Prestige Rank (Highest to Lowest) 1 2 3 4 Rejected 28 97 93 55 Admitted 33 54 28 12 Number of students Number Chi-square test: Two-tailed P-value = 0.001, Degrees of freedom = 3 Simple Situation An Associate Vice-President (Research) is interested in knowing what other factors affect admission into graduate school Variables of Interest (Independent variables): – GRE Score - continuous – Percent Marks - continuous – Prestige of the undergraduate institution – rank (1 to 4) Outcome or Response variable: – Admission to Graduate School is Yes / No (binary) Example and data from ULCA Academic Technology Service: http://www.ats.ucla.edu/stat/sas/dae/logit.htm Logistic Regression Data SAS Code GRE Mark Prestige Adm proc means; 660 82 3 1 var gre mark; 800 90 1 1 run; 640 70 4 1 520 63 4 0 proc freq; 760 65 2 1 tables rank admission admission*rank; 560 65 1 1 run; 400 67 2 0 540 75 3 1 700 88 2 0 800 90 4 0 440 71 1 0 760 90 1 1 700 67 2 0 700 90 1 1 480 76 3 0 780 87 4 0 … …. Proc Logistic proc logistic descending; class rank / param=ref; model admission = gre mark rank; contrast 'Rank 1 vs 2' rank 1 -1 0 /estimate=parm; contrast 'Rank 2 vs 3' rank 0 1 -1 /estimate=parm; contrast 'GRE200' intercept 1 gre 200 mark 74.78 rank 0 1 0 /estimate=prob; contrast 'GRE300' intercept 1 gre 300 mark 74.78 rank 0 1 0 /estimate=prob; contrast 'GRE400' intercept 1 gre 400 mark 74.78 rank 0 1 0 /estimate=prob; contrast 'GRE500' intercept 1 gre 500 mark 74.78 rank 0 1 0 /estimate=prob; contrast 'GRE600' intercept 1 gre 600 mark 74.78 rank 0 1 0 /estimate=prob; contrast 'GRE700' intercept 1 gre 700 mark 74.78 rank 0 1 0 /estimate=prob; contrast 'GRE800' intercept 1 gre 800 mark 74.78 rank 0 1 0 /estimate=prob; Run; How about the Crossed-Categorical Factors? A researcher (me!) is interested to examine factors leading to successful pregnancy outcome: – Blood progesterone levels during previous cycle (luteal- vs. subluteal-P4) – Time between luteolysis and exogenous LH (long- vs. short) – Can subluteal progesterone compensate for short treatment time? (P4*LH interaction) – Does parity matter ? (first-time moms vs. others) – Data were gathered over 2 years (replicate 1 and 2) Approaches LOGISTIC GENMOD GLIMMIX GLM / PROC MIXED Glimmix – Fixed Factors PROC glimmix method=quad; CLASS Progest Proest Type Data Replicate; ID Replicate Progest Proest Type Foll_Dia Preg 32 1 High Long A 14 0 46 1 High Long A 12 1 MODEL Preg (event="1") = 134 1 High Long A 11 1 171 1 High Long B 11 0 Progest Proest Type Replicate 178 2 High Long B 12 1 12 2 High Long A 16 1 34 2 High Long A 15 1 Progest*Proest Progest*Type 36 2 High Long A 15 0 82 2 High Long B 15 1 Proest*Type / dist=bin link=logit; 1 1 High Short B 9 0 17 1 High Short A 9 0 21 1 High Short A 10 0 LSMEANS Progest*Proest /diff 53 1 High Short A 12 0 …………….. lines ilink or adjust=tukey; run; Glimmix – Mixed Factors PROC glimmix method=quad; CLASS Progest Proest Type Replicate; Data ID Replicate Progest Proest Type Foll_Dia Preg MODEL Preg (event="1") = 32 1 High Long A 14 0 46 1 High Long A 12 1 Progest Proest Type 134 1 High Long A 11 1 171 1 High Long B 11 0 178 2 High Long B 12 1 Progest*Proest Progest*Type 12 2 High Long A 16 1 34 2 High Long A 15 1 Proest*Type / dist=bin link=logit; 36 2 High Long A 15 0 82 2 High Long B 15 1 1 1 High Short B 9 0 Random intercept 17 1 High Short A 9 0 21 1 High Short A 10 0 /subject=Replicate; 53 1 High Short A 12 0 …………….. LSMEANS Progest*Proest /diff lines ilink or adjust=tukey; run; Conclusions Use KISS principle We can analyze dichotomous response variable by: – Chi-Square – Logistic regression – GenMod / Glimmix .
Details
-
File Typepdf
-
Upload Time-
-
Content LanguagesEnglish
-
Upload UserAnonymous/Not logged-in
-
File Pages20 Page
-
File Size-