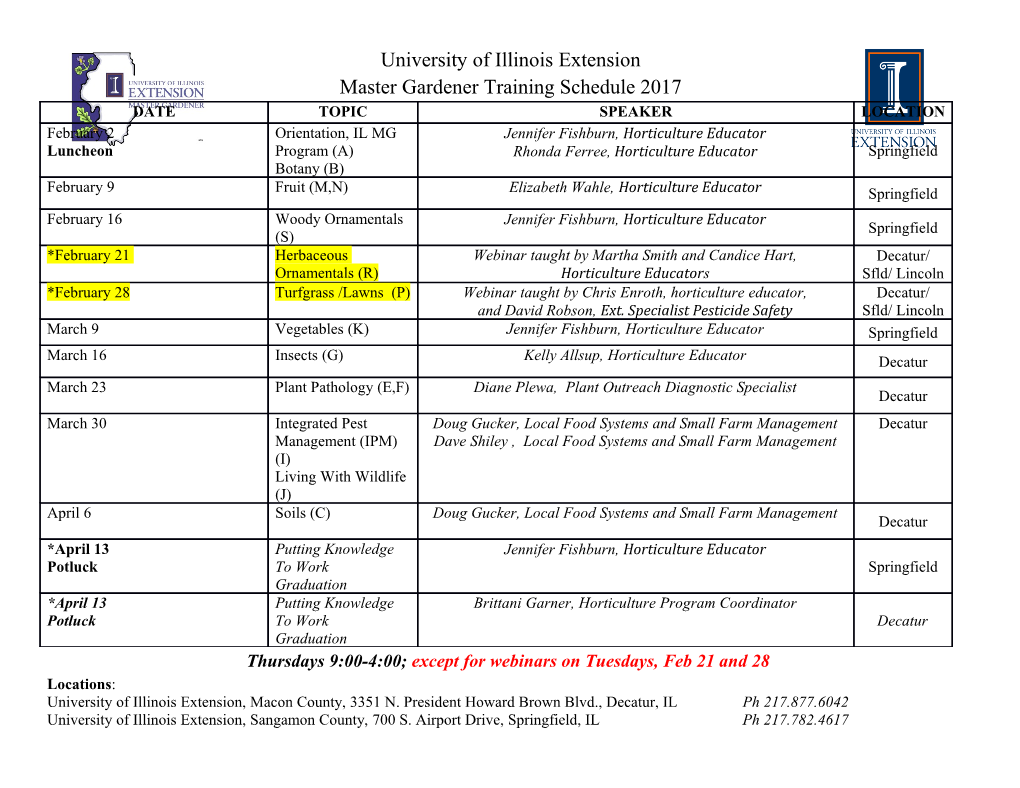
Behavioral Game Theory Heinrich Harald Nax ETH Zurich¨ a thesis submitted for habilitation at the professorship of computational social science in the department of humanities, social and political sciences (d-gess). 2015 Gr¨ußean Oma K¨athi,Elisabeth Eiche und Wilhelm und Margarete Busch. Behavioral Game Theory HH Nax, 2015 Habilitation Committee: Prof. Dirk Helbing (Computational Social Science, ETH Z¨urich) Prof. Guillaume Hollard (Economics, Ecole´ polytechnique) Prof. Andreas Diekmann (Sociology, ETH Z¨urich) Prof. Tatsuyoshi Saijo (Experimental Economics, Kochi University of Technology) 2 Thesis outline In one of his last publications, \The Agencies Method for Modeling Coalitions and Cooperation in Games" (IGTR 10: 539-564, 2008), John F. Nash wrote I feel, personally, that the study of experimental games is the proper route of travel for finding \the ultimate truth" in relation to games as played by human players. But in practical game theory the players can be corporations or states; so the problem of usefully analyzing a game does not, in a practical sense, re- duce to a problem only of the analysis of human behavior. It is apparent that actual human behavior is guided by complex human instincts promoting cooper- ation among individuals and that moreover there are also the various cultural influences acting to modify individual human behavior and at least often to influence the behavior of humans toward enhanced cooperativeness. To most, this quote will come as a surprise because the name John Nash is associated, not with behavioral or experimental research, but rather with the mathematics of game theory and, in particular, with the `Nash equilibrium'. The Nash equilibrium is an interactive solution concept mostly applied to idealized decision-making situations amongst infallible optimizers in the sense of pure material self-interest. But before we turn to Nash's contributions and what the scope of this present thesis in addressing some of the issues raised above will be, let us first turn to the origins and broad evolution of the fields of game theory and of the study of behavior in games. Beginning with the publication of \The Theory of Games and Economic Be- havior" by John von Neumann and Oskar Morgenstern in 1944 (Princeton University Press), the study of human interactions by what since is known as \game theory" has revolutionized the sciences, especially the social sciences and biology. In economics, for instance, game theory changed the way equilib- rium concepts are understood and eleven Nobel Prizes have since gone to game theorists. Game theory provides a sharp language to formulate mathematical models of underlying interactions that promise clean predictions, now integral parts of the social sciences toolbox. A game is defined by a mapping from various combinations of \strategies" taken by the involved \players" into resulting consequences in terms of \pay- offs”. A \solution" predicts which outcomes of the game are to be expected. A major issue with traditional/neoclassical game theory, however, has been that its solution concepts, such as the Nash equilibrium (John F. Nash, 1950) or the strong equilibrium (Robert Aumann, 1959), rely on four rather extreme behavioral and informational assumptions. These are: 1. The joint strategy space is common knowledge. 2. The payoff structure is common knowledge. 3. Players have correct beliefs about other players' behaviors and beliefs. 4. Players optimize their behavior so as to maximize their own material payoffs. 3 In the real-world, the whole ensemble of these assumptions is often unten- able. Players often do not behave like infallible optimizers in the sense of pure material self-interest, and it would be negligent to think of the resulting consistent and structured deviations as inexplicable irrationalities. \Behav- ioral game theory", the title and subject of this thesis, seeks to model these. Broadly speaking, behavioral game theory is separable into two strands of models. The first strand of models presumes that the mismatch between theory and real-world behaviors may be the result of capacity and/or informational con- straints. Hence, decisions are not best described by strictly maximizing be- havior. In particular, maximization fails when players have incomplete infor- mation about the structure of the game and/or about the payoff consequences of different actions taken by themselves and others for the other players. In a repeated game setting, moreover, players may be unable -or only imperfectly able- to observe other players' actions and payoffs as the game goes on. Hence, to describe more realistic human behaviors in complex game settings, models of boundedly rational behavior, possibly allowing for learning dynamics, are necessary. The second strand of explanations for behaviors that consistently contradict equilibrium predictions based on the standard assumptions of self-interest and unbounded rationality may be that players are guided by alternative pref- erences. In other words, provided the assumption of material self-interest is flawed and that, instead, higher-order motives such as altruism or social norms guide a player's actions, then self-interest predictions are misguided, even if players follow maximizing behavior. It is not that players' behavior is not maximizing, rather their maximand is something else than narrow self- interest. The two strands of explanations both have their respective appeals, and which model is preferable will depend on the context of the application. To describe the trading behavior of agents on financial markets, for example, one may favor the first type of explanation; intention but failure to maximize own material payoff. Similarly, such an approach may be preferable to describe behavior in traffic/congestion games. By contrast, richer preference formulations allowing for, for example, reciprocal considerations may be suitable to model volun- tary contributions in situations such as community effort tasks or collective bargaining. Of course, in reality, we would expect an admixture of both ex- planations to matter in most situations, and their relative degrees to depend on the precise context and setting of the game. \Behavioral game theory", with the subtitle \Experiments in strategic inter- action", is also the title of one of the first and best-known textbooks that introduce this area of research to a broader audience (Camerer 2003; Prince- ton University Press). Therein, the two strands of explanations sketched above are expertly summarized and reviewed. This thesis builds on this body of work, its aim being to synthesize the two approaches. New methods to combine and 4 to disentangle the two are proposed and applied to different games, illustrating the need for a more nuanced theory, allowing for context-dependent behavior in games. The thesis consists of theoretical and behavioral studies. Moreover, the thesis also proposes a theoretical model of the complex decision-making of coalitions of individuals, and not just of individuals. The thesis is structured as follows: Chapter 1. Introduction Chapter 1 provides an introduction to behavioral game theory. It focusses on the context of public goods games in general, and on social preference explanations in particular. A novel, unbiased method to estimate players social preferences is proposed, and used to disentangle social preferences from other factors such as learning. Data from several laboratory experiments is used. Interactions in preferences are discovered and assessed. The author is the first and main author of the materials contained in this chap- ter. Underlying the chapter are two papers. One is joint work with Maxwell Burton-Chellew (Department of Zoology and Magdalen College, University of Oxford) and Stuart West (Department of Zoology and Nuffield College, Uni- versity of Oxford), the other with Ryan Murphy and Kurt Ackermann (Depart- ment of Humanities, Social and Political Sciences, ETH Z¨urich). One paper is currently under review, the other published in Economics Letters (\Interactive preferences", Economics Letters 135: 133-136, 2015). Chapter 2. Learning Chapter 2 turns to learning behavior. It introduces a model of directional learning and proposes a novel solution concept that bridges Nash (1950) and Aumann (1959)-strong equilibria in the context of public goods games. Direc- tional learning is shown to offer an alternative explanation of the behavioral regularities commonly observed in public goods experiments. This chapter is joint work with MatjaˇzPerc (Faculty of Natural Sciences and Mathematics, University of Maribor, Slovenia & Department of Physics, Fac- ulty of Science, King Abdulaziz University, Jeddah, Saudi Arabia). The paper is published in Scientific Reports (\Directional learning and the provisioning of public goods", Scientific Reports 5: 8010, 2015). MatjaˇzPerc and the author are joint first authors. Chapter 3. Social preferences versus learning Chapter 3 creates a horse-race between social preference explanations and directional learning in a repeated public goods game setting. Learning comes 5 out as the winner, but explanations related to social preferences, especially conditional cooperation, continue to matter. This chapter is joint work with Maxwell Burton-Chellew (Department of Zo- ology and Magdalen College, University of Oxford) and Stuart West (Depart- ment of Zoology and Nuffield College, University of Oxford). It is published in the Proceedings of the Royal Society B (\Payoff-based learning explains the decline in cooperation in public
Details
-
File Typepdf
-
Upload Time-
-
Content LanguagesEnglish
-
Upload UserAnonymous/Not logged-in
-
File Pages306 Page
-
File Size-