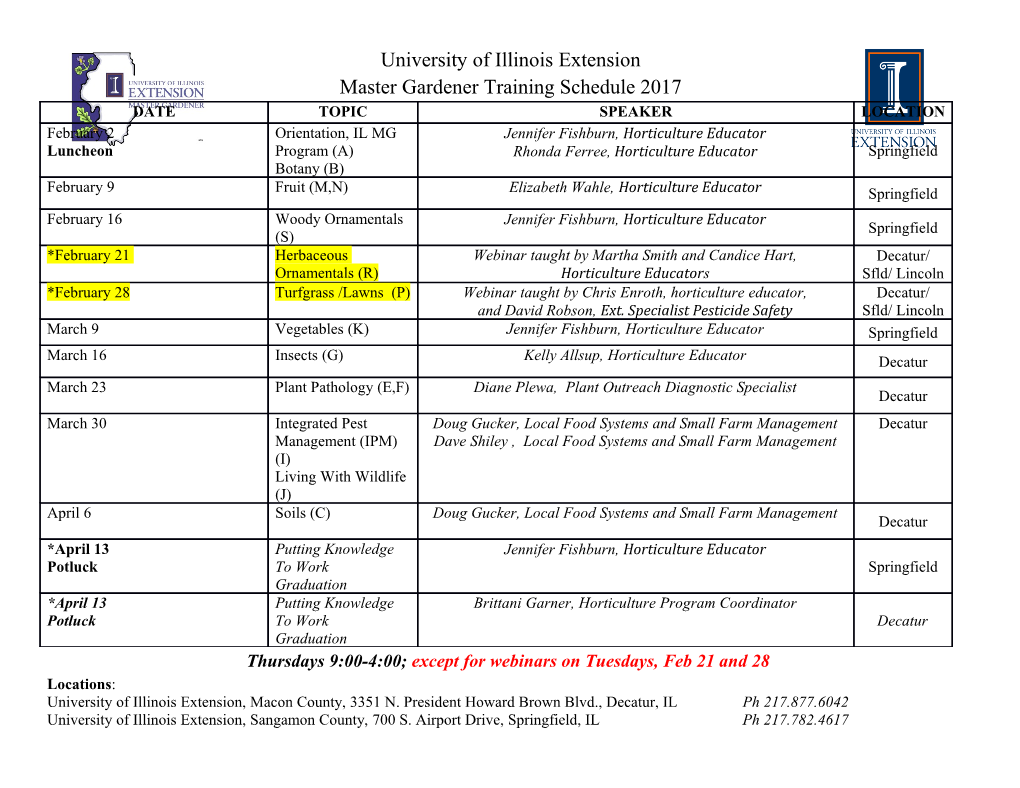
Practical Statistics – part II ‘Composite hypothesis, Nuisance Parameters’ W. Verkerke (NIKHEF) Wouter Verkerke, NIKHEF Summary of yesterday, plan for today • Start with basics, gradually build up to complexity of Statistical tests with simple hypotheses for counting data “What do we mean with probabilities” Statistical tests with simple hypotheses for distributions “p-values” “Optimal event selection & Hypothesis testing as basis for event selection machine learning” Composite hypotheses (with parameters) for distributions “Confidence intervals, Maximum Likelihood” Statistical inference with nuisance parameters “Fitting the background” Response functions and subsidiary measurements “Profile Likelihood fits” Introduce concept of composite hypotheses • In most cases in physics, a hypothesis is not “simple”, but “composite” • Composite hypothesis = Any hypothesis which does not specify the population distribution completely • Example: counting experiment with signal and background, that leaves signal expectation unspecified Simple hypothesis ~ s=0 With b=5 s=5 s=10 s=15 Composite hypothesis (My) notation convention: all symbols with ~ are constants Wouter Verkerke, NIKHEF A common convention in the meaning of model parameters • A common convention is to recast signal rate parameters into a normalized form (e.g. w.r.t the Standard Model rate) Simple hypothesis ~ s=0 With b=5 s=5 s=10 s=15 Composite hypothesis ‘Universal’ parameter interpretation makes it easier to work with your models μ=0 no signal Composite hypothesis μ=1 expected signal with normalized rate parameter μ>1 more than expected signal Wouter Verkerke, NIKHEF What can we do with composite hypothesis • With simple hypotheses – inference is restricted to making statements about P(D|hypo) or P(hypo|D) • With composite hypotheses – many more options • 1 Parameter estimation and variance estimation – What is value of s for which the observed data is most probable? s=5.5 ± 1.3 – What is the variance (std deviation squared) in the estimate of s? • 2 Confidence intervals – Statements about model parameters using frequentist concept of probability – s<12.7 at 95% confidence level – 4.5 < s < 6.8 at 68% confidence level • 3 Bayesian credible intervals – Bayesian statements about model parameters – s<12.7 at 95% credibility Wouter Verkerke, NIKHEF Parameter estimation using Maximum Likelihood • Likelihood is high for values of p that result in distribution similar to data • Define the maximum likelihood (ML) estimator to be the procedure that finds the parameter value for which the likelihood is maximal. Wouter Verkerke, NIKHEF Parameter estimation – Maximum likelihood • Practical estimation of maximum likelihood performed by minimizing the negative log-Likelihood – Advantage of log-Likelihood is that contributions from events can be summed, rather than multiplied (computationally easier) • In practice, find point where derivative of –logL is zero d ln L( p) 0 dp pi pˆi • Standard notation for ML estimation of p is^ p Wouter Verkerke, UCSB Example of Maximum Likelihood estimation • Illustration of ML estimate on Poisson counting model -log L(N|s) versus N [s=0,5,10,15] -log L(N|s) versus s [N=7] s=0 s=5 s=10 s=15 ^ s=2 • Note that Poisson model is discrete in N, but continuous in s! Wouter Verkerke, NIKHEF Properties of Maximum Likelihood estimators • In general, Maximum Likelihood estimators are – Consistent (gives right answer for N) – Mostly unbiased (bias 1/N, may need to worry at small N) – Efficient for large N (you get the smallest possible error) – Invariant: (a transformation of parameters 2 2 will Not change your answer, e.g pˆ p • MLE efficiency theorem: the MLE will be unbiased and efficient if an unbiased efficient estimator exists – Proof not discussed here – Of course this does not guarantee that any MLE is unbiased and efficient for any given problem Estimating parameter variance • Note that ‘error’ or ‘uncertainty’ on a parameter estimate is an ambiguous statement • Can either mean an interval with a stated confidence or credible, level (e.g. 68%), or simply assume it is the square-root of the variance of a distribution For a Gaussian distribution Mean= mean and variance <x> map to parameters for mean and sigma2 Variance = and interval defined by 2 2 √V contains 68% <x >-<x> of the distribution (=‘1 sigma’ by definition) Thus for Gaussian distributions all common definitions of ‘error’ work out to the same numeric value Wouter Verkerke, NIKHEF Estimating parameter variance • Note that ‘error’ or ‘uncertainty’ on a parameter estimate is an ambiguous statement • Can either mean an interval with a stated confidence or credible, level (e.g. 68%), or simply assume it is the square-root of the variance of a distribution For other distributions Mean= intervals by √V do <x> not necessarily contain 68% of the distribution Variance = <x2>-<x>2 Wouter Verkerke, NIKHEF The Gaussian as ‘Normal distribution’ • Why are errors usually Gaussian? • The Central Limit Theorem says • If you take the sum X of N independent measurements xi, 2 each taken from a distribution of mean mi, a variance Vi=si , the distribution for x (a) has expectation value X i i 2 (b) has variance V (X ) Vi si i i (c) becomes Gaussian as N Wouter Verkerke, UCSB Demonstration of Central Limit Theorem 5000 numbers taken at random from a uniform distribution between [0,1]. 1 1 – Mean = /2, Variance = /12 N=1 5000 numbers, each the sum of 2 random numbers, i.e. X = x1+x2. – Triangular shape N=2 Same for 3 numbers, X = x1 + x2 + x3 N=3 Same for 12 numbers, overlaid curve is exact Gaussian distribution Important: tails of distribution converge very slowly CLT N=12 often not applicable for ‘5 sigma’ discoveries Estimating variance on parameters • Variance on of parameter can also be estimated from Likelihood using the variance estimator 2 1 From Rao-Cramer-Frechet d ln L inequality 2 ˆ db sˆ( p) V ( p) 1 dp 2 V( pˆ) d 2 ln L d p d 2 p b = bias as function of p, inequality becomes equality • Valid if estimator is efficient and unbiased! in limit of efficient estimator • Illustration of Likelihood Variance estimate on a Gaussian distribution 1 é 1 æ x - m ö2 ù f (x | m,s ) = expê- ç ÷ ú s 2p ëê 2 è s ø ûú 1 æ x - m ö2 ln f (x | m,s ) = -lns - ln 2p + ç ÷ 2 è s ø d ln f -1 d 2 ln f 1 = Þ 2 = 2 ds x=m s d s x=m s Wouter Verkerke, NIKHEF Relation between Likelihood and c2 estimators • Properties of c2 estimator follow from properties of ML estimator using Gaussian probability density functions Gaussian Probability Density Function in p for single measurement y±σ from a predictive function f(x|p) Take log, Sum over all points (xi ,yi ,si) The Likelihood function in p for given points xi(si) and function f(x ;p) i • The c2 estimator follows from ML estimator, i.e it is – Efficient, consistent, bias 1/N, invariant, – But only in the limit that the error on xi is truly Gaussian Wouter Verkerke, UCSB Interval estimation with fundamental methods • Can also construct parameters intervals using ‘fundamental’ methods explored earlier (Bayesian or Frequentist) • Construct Confidence Intervals or Credible Intervals with defined probabilistic meaning, independent of assumptions on normality of distribution (Central Limit Theorem) “95% C.L.” • With fundamental methods you greater flexibility in types of interval. E.g when no signal observed usually wish to set an upper limit (construct ‘upper limit interval’) Wouter Verkerke, NIKHEF Reminder - the Likelihood as basis for hypothesis testing • A probability model allows us to calculate the probability of the observed data under a hypothesis • This probability is called the Likelihood s=0 s=5 P(obs|theo) s=10 is called the s=15 Likelihood Wouter Verkerke, NIKHEF Reminder - Frequentist test statistics and p-values • Definition of ‘p-value’: Probability to observe this outcome or more extreme in future repeated measurements is x%, if hypothesis is true • Note that the definition of p-value assumes an explicit ordering of possible outcomes in the ‘or more extreme’ part s=0 p Poisson (N;b 0)dN ( 0.23) b Nobs s=5 s=10 s=15 Wouter Verkerke, NIKHEF P-values with a likelihood ratio test statistic • With the introduction of a (likelihood ratio) test statistic, hypothesis testing of models of arbitrary complexity is now reduced to the same procedure as the Poisson example λobs ¥ log(λ) • Except that we generally p - value = ò f (l | Hb ) don’t know distribution f(λ)… lobs A different Likelihood ratio for composite hypothesis testing • On composite hypotheses, where both null and alternate hypothesis map to values of μ, we can define an alternative likelihood-ratio test statistics that has better properties Hypothesis ‘simple hypothesis’ ‘composite hypothesis’ μ that is being tested L(N | H0 ) L(N | m) l (N) = lm (Nobs ) = L(N | H1) L(N | mˆ) ‘Best-fit value’ • Advantage: distribution of new λμ has known asymptotic form • Wilks theorem: distribution of –log(λμ) is asymptotically 2 distribution as a χ with Nparam degrees of freedom* *Some regularity conditions apply obs • Asymptotically, we can directly calculate p-value from λμ Wouter Verkerke, NIKHEF What does a χ2 distribution look like for n=1? • Note that it for n=1, it does not peak at 1, but rather at 0… Wouter Verkerke, NIKHEF Composite hypothesis testing in the asymptotic regime • For ‘histogram example’: what is p-value of null-hypothesis ‘likelihood assuming zero signal strength’ L(data | m = 0) μ^ is best fit t0 = -2ln L(data | mˆ) value of μ ‘likelihood of best fit’ -logm On signal-like
Details
-
File Typepdf
-
Upload Time-
-
Content LanguagesEnglish
-
Upload UserAnonymous/Not logged-in
-
File Pages88 Page
-
File Size-